Enhance Robustness of Language Models Against Variation Attack Through Graph Integration
arXiv (Cornell University)(2024)
关键词
Language Modeling,Topic Modeling,Word Representation,Syntax-based Translation Models,Part-of-Speech Tagging
AI 理解论文
溯源树
样例
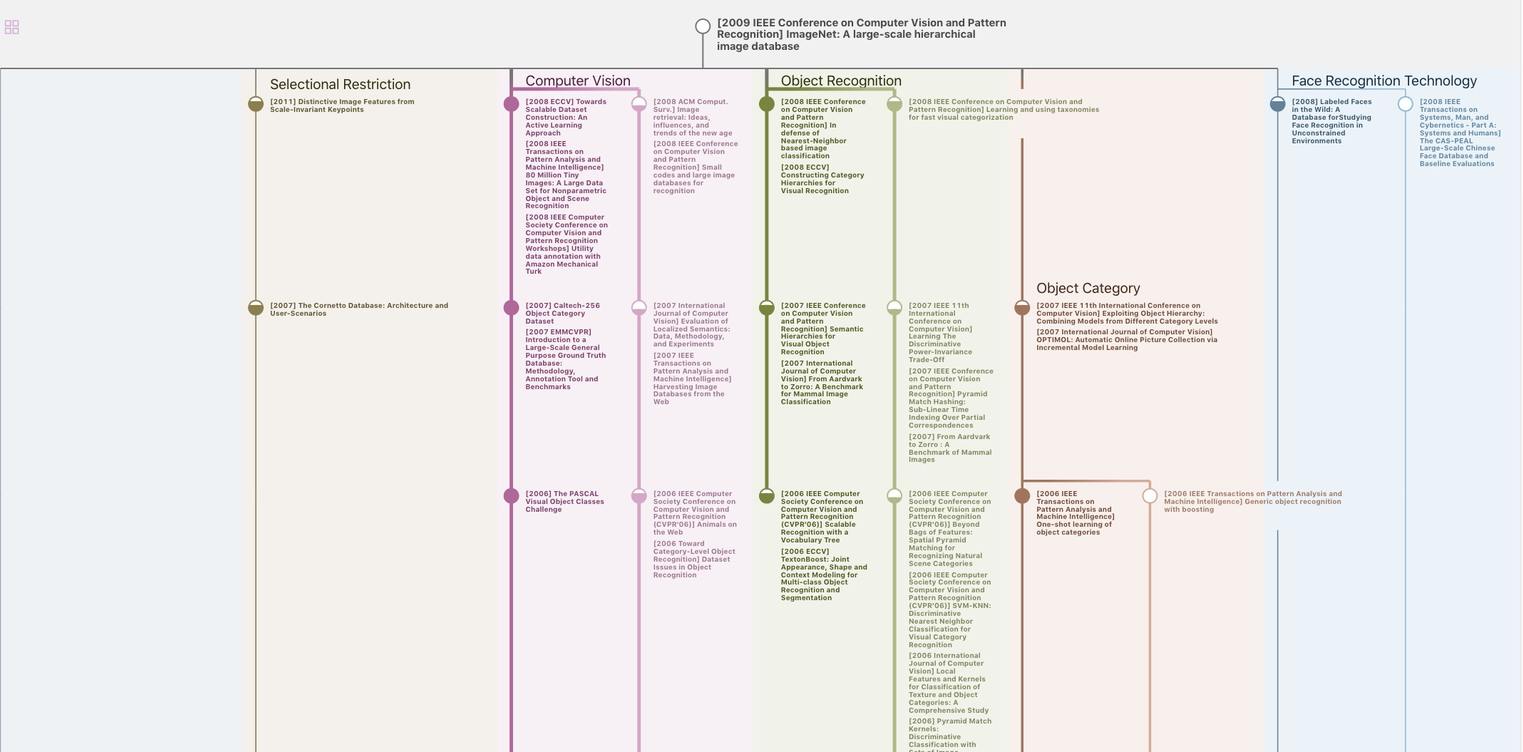
生成溯源树,研究论文发展脉络
Chat Paper
正在生成论文摘要