Utilising Intraoperative Respiratory Dynamic Features for Developing and Validating an Explainable Machine Learning Model for Postoperative Pulmonary Complications
British journal of anaesthesia(2024)
摘要
Background: Timely detection of modifiable risk factors for postoperative pulmonary complications (PPCs) could inform ventilation strategies that attenuate lung injury. We sought to develop, validate, and internally test machine learning models that use intraoperative respiratory features to predict PPCs. Methods: We analysed perioperative data from a cohort comprising patients aged 65 yr and older at an academic medical centre from 2019 to 2023. Two linear and four nonlinear learning models were developed and compared with the current gold -standard risk assessment tool ARISCAT (Assess Respiratory Risk in Surgical Patients in Catalonia Tool). The Shapley additive explanation of artificial intelligence was utilised to interpret feature importance and interactions. Results: Perioperative data were obtained from 10 284 patients who underwent 10 484 operations (mean age [range] 71 [65-98] yr; 42% female). An optimised XGBoost model that used preoperative variables and intraoperative respiratory variables had area under the receiver operating characteristic curves (AUROCs) of 0.878 (0.866-0.891) and 0.881 (0.879-0.883) in the validation and prospective cohorts, respectively. These models outperformed ARISCAT (AUROC: 0.496-0.533). The intraoperative dynamic features of respiratory dynamic system compliance, mechanical power, and driving pressure were identified as key modifiable contributors to PPCs. A simplified model based on XGBoost including 20 variables generated an AUROC of 0.864 (0.852-0.875) in an internal testing cohort. This has been developed into a web -based tool for further external validation (https://aorm.wchscu.cn/). Conclusions: These findings suggest that real-time identification of surgical patients' risk of postoperative pulmonary complications could help personalise intraoperative ventilatory strategies and reduce postoperative pulmonary complications.
更多查看译文
关键词
dynamic respiratory features,intraoperative ventilation strategy,machine learning model,postoperative pulmonary complications,real-time risk prediction
AI 理解论文
溯源树
样例
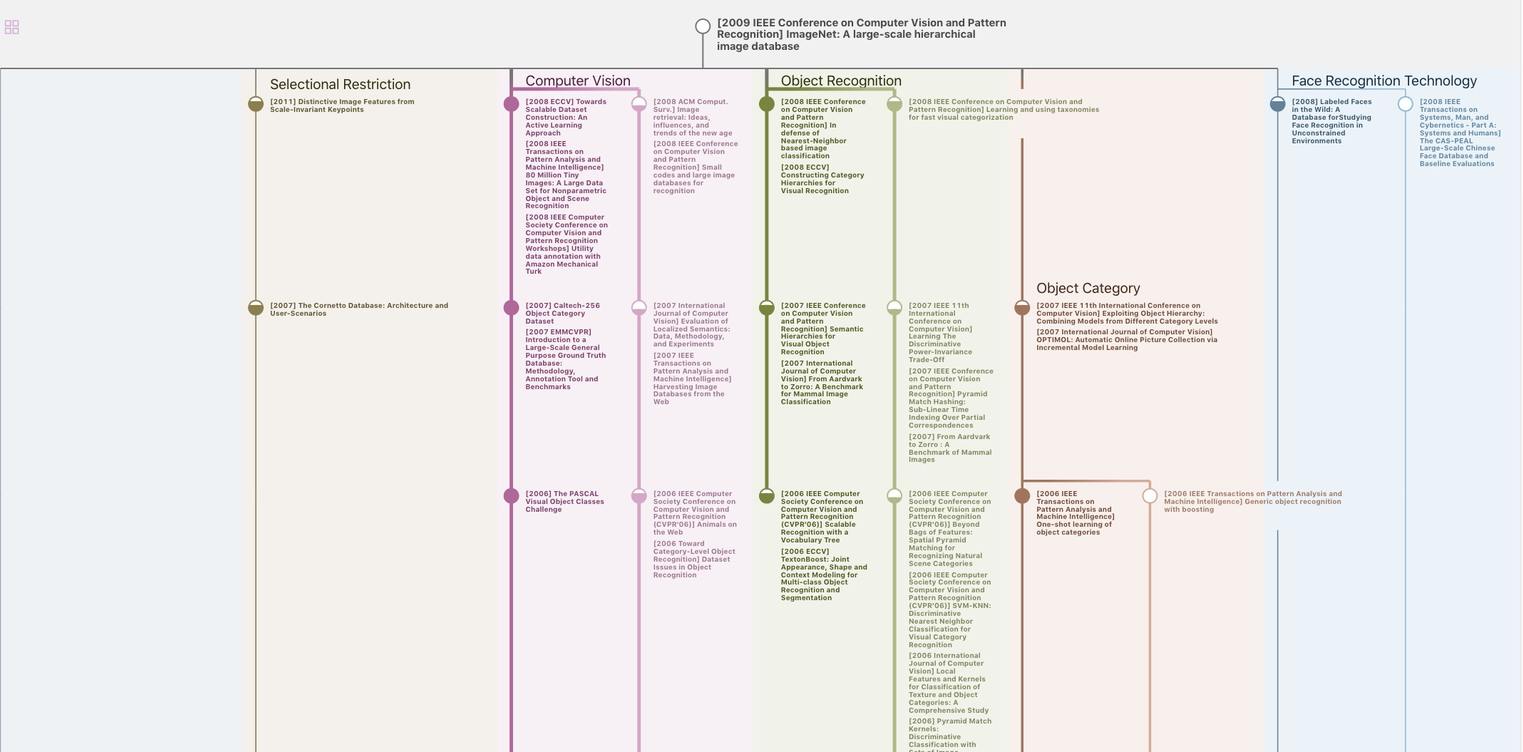
生成溯源树,研究论文发展脉络
Chat Paper
正在生成论文摘要