Automatic Reconstruction of Closely Packed Fabric Composite RVEs Using Yarn-Level Micro-Ct Images Processed by Convolutional Neural Networks (cnns) and Based on Physical Characteristics
COMPOSITES SCIENCE AND TECHNOLOGY(2024)
摘要
Micro-CT scanning is an advanced technique to reconstruct inner architectures for RVEs of fabric composites. Currently, however, there exist few automatic approaches to separate closely packed yarns in the micro-CT images with economical resolution that can only identify yarns but not individual fibers. To tackle this issue, an innovative method has been developed in this work to identify cross-section area of the yarns via deep-learning-based image segmentation, and then reconstruct 3D geometry model of the composites containing local fiber orientations. The image segmentation was achieved through semantic segmentation by U-Net with validation accuracy of 87 % counted by mIOU and object detection by YOLO v8 with validation accuracy of 99.5 % counted by mAP50. Micro-CT slices with different morphological characteristics were divided into three groups via a ResNet50-based image classification network and selected in ratio to form the training datasets for U-Net and YOLO v8 with high representativity and efficiency. Extraction of individual cross-sections of weft and warp yarns were conducted only using the micro-CT scanning from one angle of view to reduce scanning cost and yarn-to-yarn penetration error. An algorithm considering physical constraints of the yarns was also completed to estimate local fiber orientations with maximum error of 18.065 degrees, degrees , nearly 50 % smaller than the existing method.
更多查看译文
关键词
Fabric Defect Detection,Texture Analysis,Inverse Modeling,Textile Engineering,Surface Defect Detection
AI 理解论文
溯源树
样例
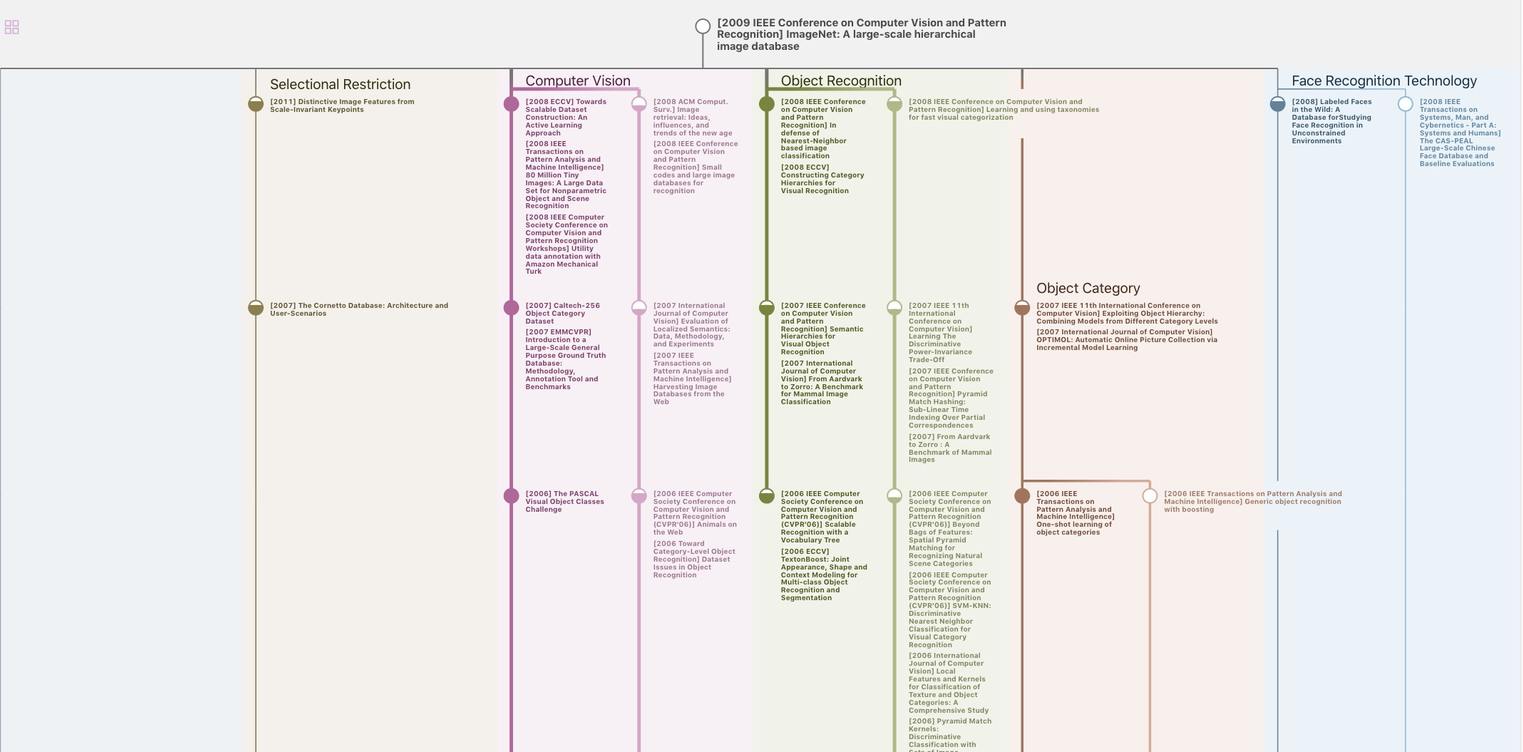
生成溯源树,研究论文发展脉络
Chat Paper
正在生成论文摘要