Single imbalanced domain generalization network for intelligent fault diagnosis of compressors in HVAC systems under unseen working conditions
Energy and Buildings(2024)
摘要
Effective fault diagnosis of compressors in heating, ventilation, and air conditioning (HVAC) systems is critical to ensure service reliability and boost energy efficiency. HVAC compressors are distributed in different areas and work under heterogeneous conditions, which poses emerging challenges to their data-driven modeling. Most existing methods assume multiple category-balanced source domains for model training. Although domain adaptation and generalization methods have emerged to address the data distribution discrepancies in cross-domain fault diagnosis, limited source domains and imbalanced fault categories across domains still constrain the real-world applicability of data-driven models in HVAC compressor fault diagnosis under unseen working conditions. Therefore, this paper studies a significant fault diagnosis problem named single imbalanced domain generalization (SIDG) and proposes a corresponding network (SIDGNet) for intelligent HVAC compressor fault diagnosis. Specifically, a rare fault diagnosis module combined with focal loss is introduced to tackle the class imbalance problem. To achieve better diagnostic boundaries and resist unknown data distribution discrepancies, joint supervised contrastive learning and adversarial learning with specialized data augmentation are introduced as auxiliary modules to improve the robustness and generalizability of SIDGNet. An improved uncertainty-based dynamic weighting mechanism is developed to intelligently balance the weights of module-specific losses during training, which ensures an efficient and stable optimization process. Extensive SIDG fault diagnosis experiments conducted on HVAC compressors demonstrate the superiority of SIDGNet over existing models in SIDG scenarios.
更多查看译文
关键词
HVAC system,Fault diagnosis,Neural networks,Data-driven models,Class imbalance
AI 理解论文
溯源树
样例
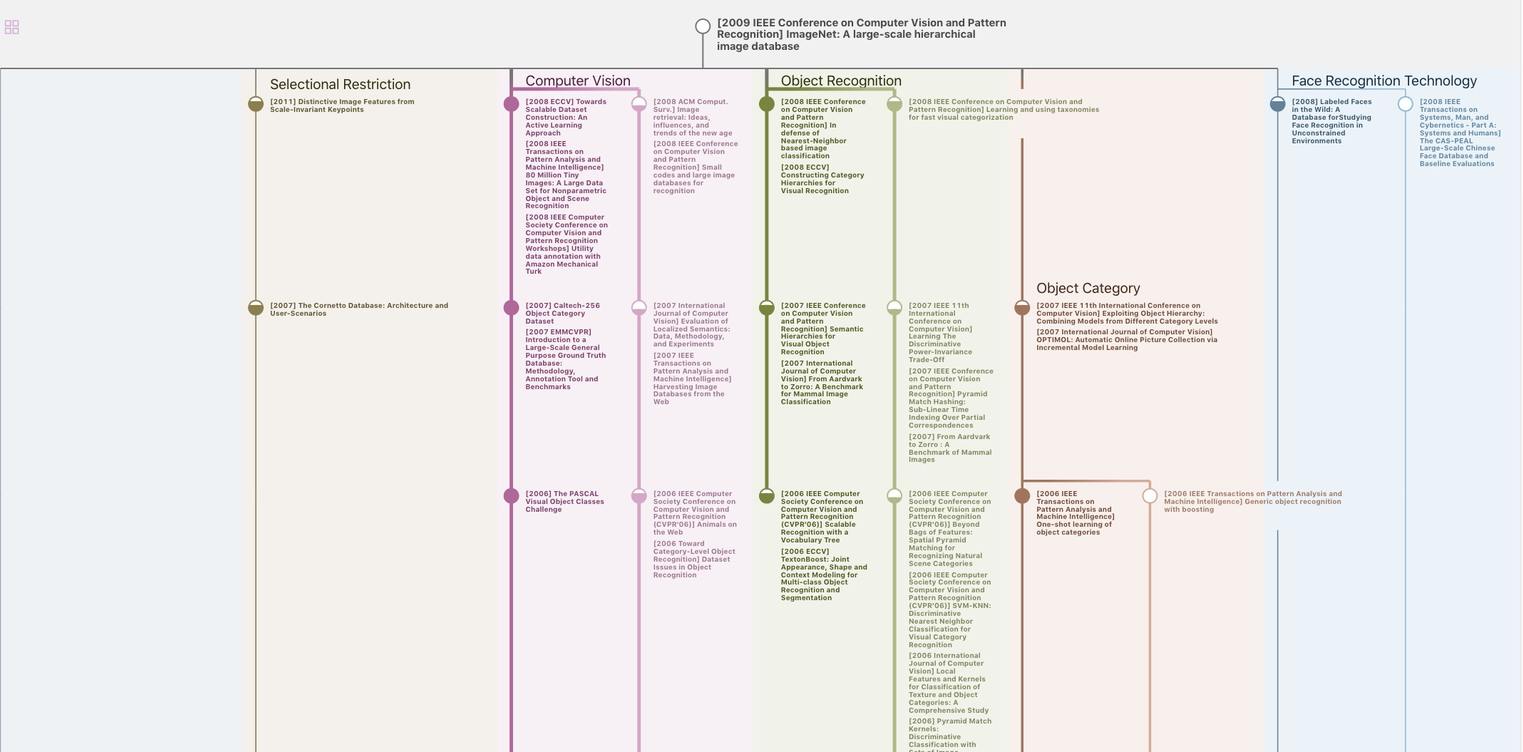
生成溯源树,研究论文发展脉络
Chat Paper
正在生成论文摘要