Improving a hydrological model by coupling it with an LSTM water use forecasting model
JOURNAL OF HYDROLOGY(2024)
摘要
Long short-term memory (LSTM) networks have demonstrated excellent capabilities in identifying nonlinear relationships between variables and have proven to be effective in water use forecast modeling. However, current LSTM water use forecasting models do not consider the influence of regional water resources, which limits their ability to make full use of hydrological data. To address this issue, a distributed hydrological model (WetSpaWW) was integrated in this study with a deep learning model (LSTM) based on water use activities. The water resources results simulated by WetSpa-WW were considered as an additional training input in the LSTM for water use forecasting. The coupled WetSpa-LSTM model was tested on the upper-middle of the Weihe River Basin, and the simulations were compared with those obtained from an experiment that did not consider water resources factors. The results indicated that the WetSpa-LSTM model performed better than the original model in simulating the streamflow. During calibration, logarithmic form of Nash-Sutcliffe efficiency (NSEln) increased from 0.33 to 0.54 and the relative error (RE) decreased from 28 % to 13 %. Moreover, we observed that water resources factors can improve the accuracy of agricultural water use forecasting (NSE increases by 0.10) more than that of non-agricultural water use forecasting (NSE increases by 0.04). Overall, this study demonstrates the value of considering regional water resources in water use forecasting, and provides a perspective for integrating a distributed hydrological model (WetSpa-WW) and a deep learning model (LSTM) based on water use activities.
更多查看译文
关键词
LSTM model,WetSpa-WW model,Weihe River Basin,Water use forecasting
AI 理解论文
溯源树
样例
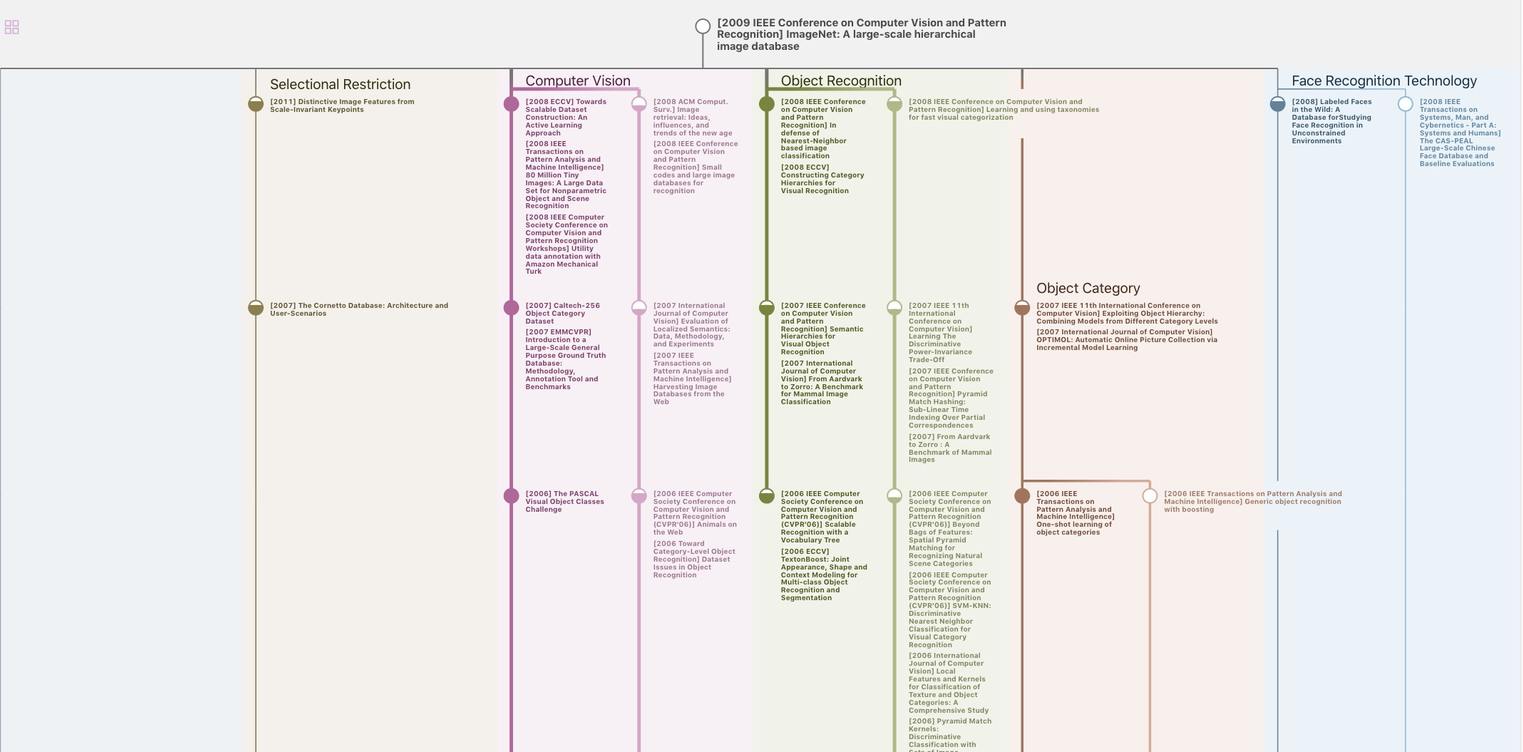
生成溯源树,研究论文发展脉络
Chat Paper
正在生成论文摘要