Machine Learning in Complex Organic Mixtures: Applying Domain Knowledge Allows for Meaningful Performance with Small Datasets.
crossref(2024)
摘要
The ability to quantify individual components of complex mixtures is a challenge found throughout the life and physical sciences. An improved capacity to generate large datasets along with the uptake of machine-learning (ML) based analysis tools has allowed for various ‘omics’ disciplines to realize exceptional advances. Other areas of chemistry that deal with complex mixtures often cannot leverage these advances. Environmental samples, for example, can be more difficult to access and the resulting small datasets are less appropriate for unconstrained ML approaches. Herein, we present an approach to address this latter issue. Using a very small environmental dataset—35 high-resolution mass spectra gathered from various solvent extractions of Canadian petroleum fractions—we show that the application of specific domain knowledge can lead to ML models with notable performance.
更多查看译文
AI 理解论文
溯源树
样例
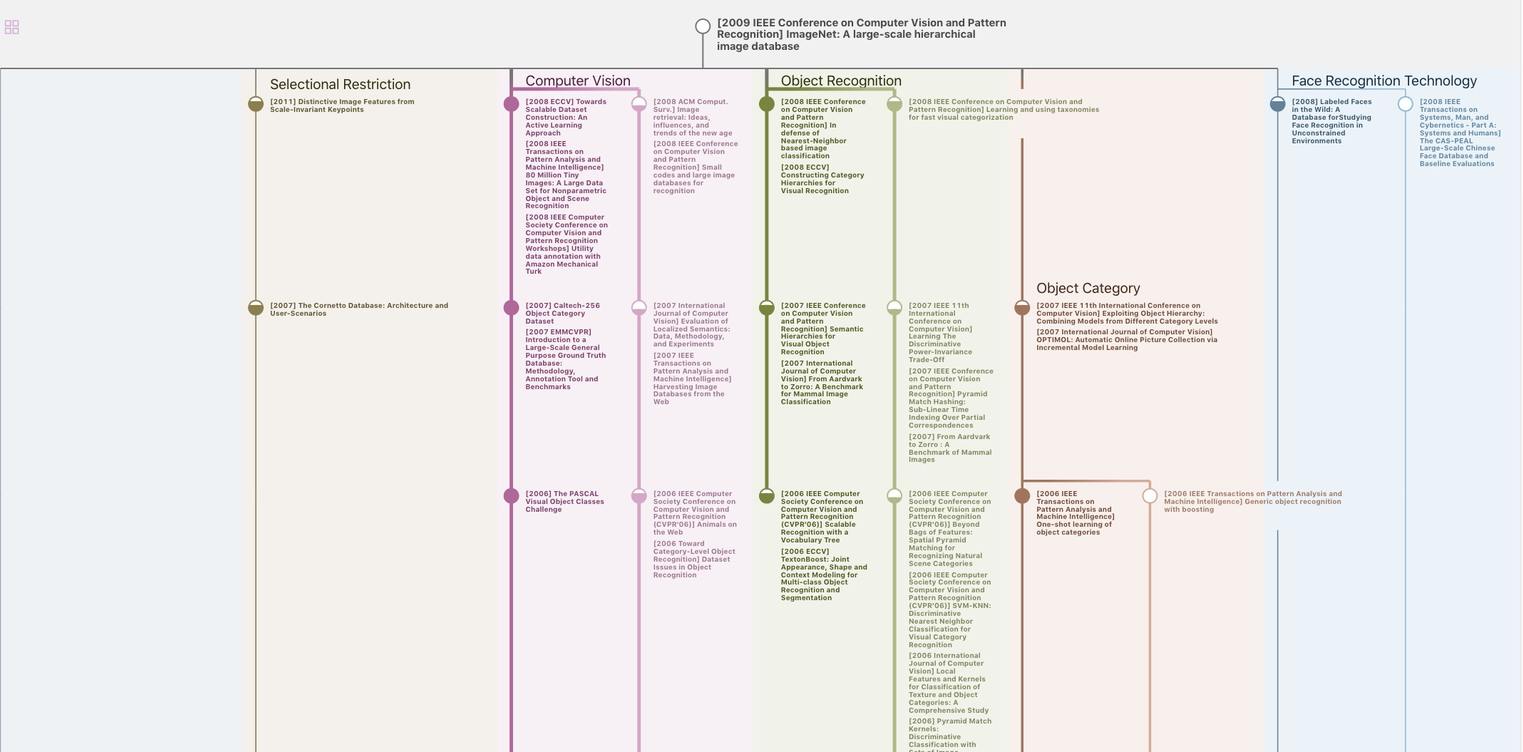
生成溯源树,研究论文发展脉络
Chat Paper
正在生成论文摘要