An end-cloud collaboration for state-of-charge estimation of lithium-ion batteries based on extended Kalman filter and convolutional neural network (CNN)-long short-term memory (LSTM)-attention mechanism (AM)
JOURNAL OF RENEWABLE AND SUSTAINABLE ENERGY(2024)
摘要
This paper introduces an innovative online state of charge (SOC) estimation method for lithium-ion batteries, designed to address the challenges of accurate and timely SOC estimation in electric vehicles under complex working conditions and computational limitations of on-board hardware. Central to this method is the concept of end-cloud collaboration, which harmonizes accuracy with real-time performance. The framework involves deploying a data-driven model on the cloud side for high-accuracy estimation, complemented by a fast model on the end side for real-time estimation. A crucial component of this system is the implementation of the extended Kalman filter on the end side, which fuses results from both ends to achieve high-accuracy and real-time online estimation. This method has been rigorously evaluated under various dynamic driving conditions and temperatures, demonstrating high accuracy, real-time performance, and robustness. The estimation results yield a root mean square error and mean absolute error of approximately 1.5% and 1%, respectively. Significantly, under the Cyber Hierarchy and Interactional Network framework, this method shows promising potential for extension to multi-state online cooperative estimation, opening avenues for advanced battery system management.
更多查看译文
AI 理解论文
溯源树
样例
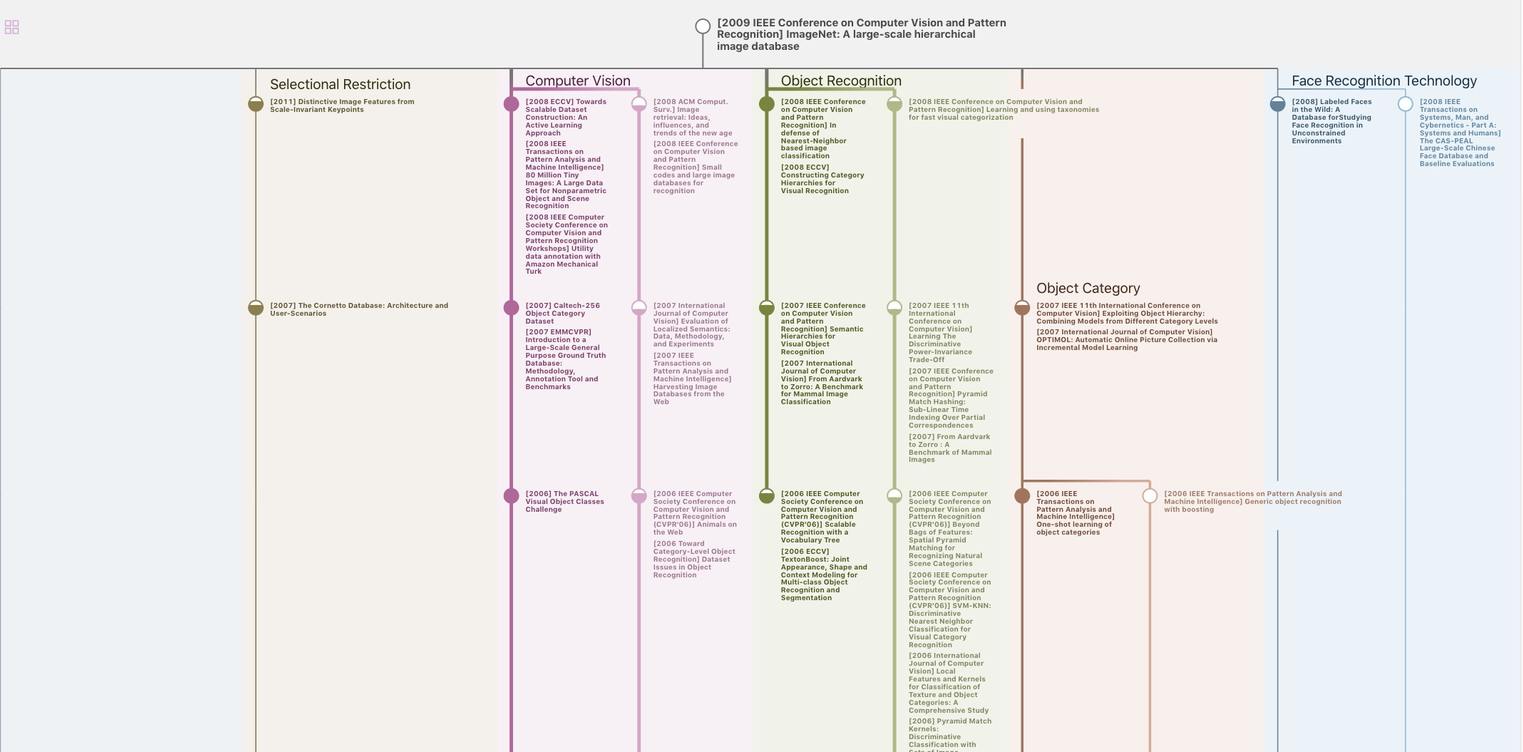
生成溯源树,研究论文发展脉络
Chat Paper
正在生成论文摘要