Neighborhood Built Environment, Obesity, and Diabetes: A Utah Siblings Study
SSM - Population Health(2024)
摘要
This study utilizes innovative computer vision methods alongside Google Street View images to characterize neighborhood built environments across Utah.
Methods
Convolutional Neural Networks were used to create indicators of street greenness, crosswalks, and building type on 1.4 million Google Street View images. The demographic and medical profiles of Utah residents came from the Utah Population Database (UPDB). We implemented hierarchical linear models with individuals nested within zip codes to estimate associations between neighborhood built environment features and individual-level obesity and diabetes, controlling for individual- and zip code-level characteristics (n=1,899,175 adults living in Utah in 2015). Sibling random effects models were implemented to account for shared family attributes among siblings (n=972,150) and twins (n=14,122)
Results
Consistent with prior neighborhood research, the variance partition coefficients (VPC) of our unadjusted models nesting individuals within zip codes were relatively small (0.5% – 5.3%), except for HbA1c (VPC=23%), suggesting a small percentage of the outcome variance is at the zip code-level. However, proportional change in variance (PCV) attributable to zip codes after the inclusion of neighborhood built environment variables and covariates ranged between 11%-67%, suggesting that these characteristics account for a substantial portion of the zip code-level effects. Non-single-family homes (indicator of mixed land use), sidewalks (indicator of walkability), and green streets (indicator of neighborhood aesthetics) were associated with reduced diabetes and obesity. Zip codes in the third tertile for non-single-family homes were associated with a 15% reduction (PR: 0.85; 95% CI: 0.79, 0.91) in obesity and a 20% reduction (PR: 0.80; 95% CI: 0.70, 0.91) in diabetes. This tertile was also associated with a BMI reduction of -0.68 kg/m2 (95% CI: -0.95, -0.40)
Conclusion
We observe modest associations between neighborhood characteristics and chronic diseases, accounting for biological, social, and cultural factors shared among siblings in this large population-based study.
更多查看译文
关键词
neighborhood built environment,chronic diseases,Google Street View,Convolutional Neural Networks,siblings
AI 理解论文
溯源树
样例
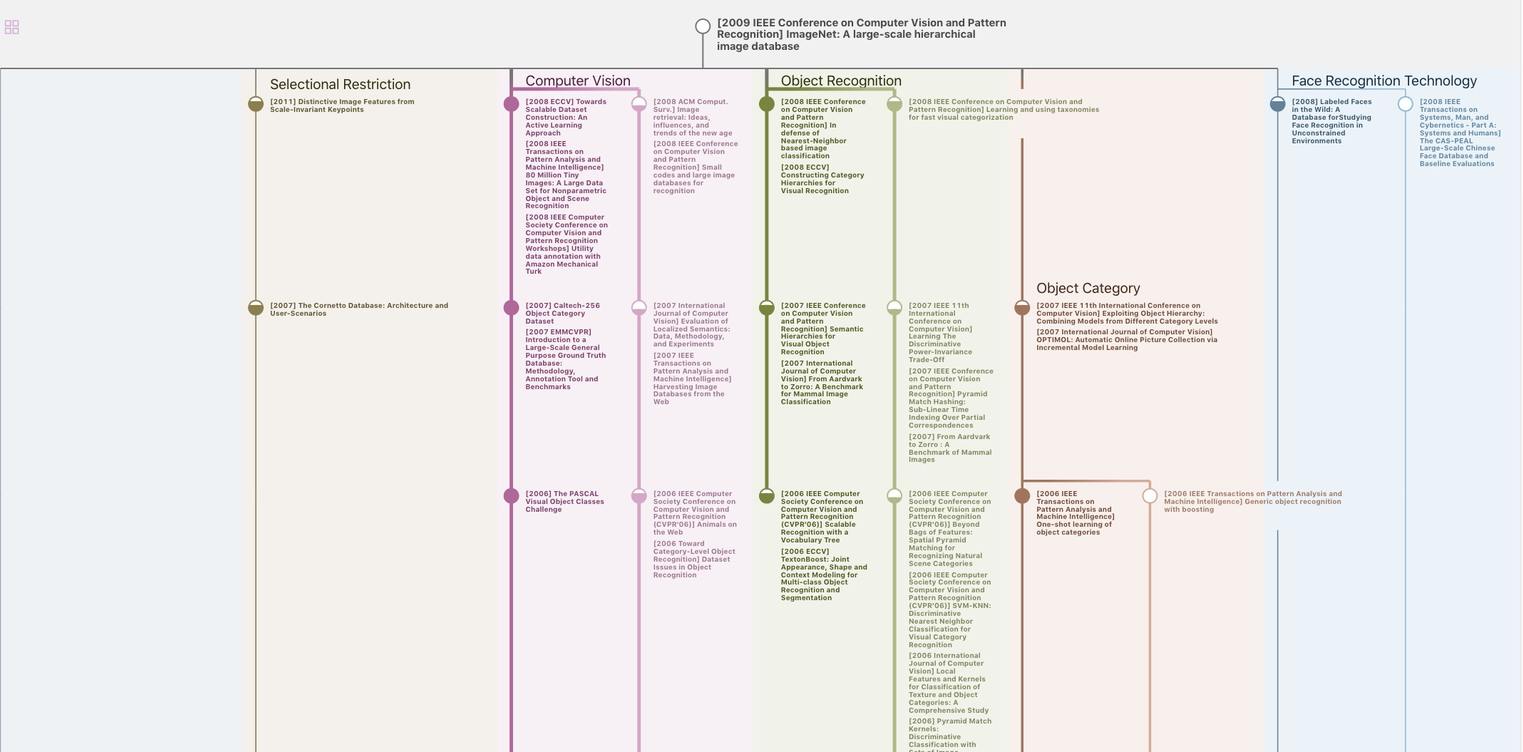
生成溯源树,研究论文发展脉络
Chat Paper
正在生成论文摘要