Attention-based Value Classification Reinforcement Learning for Collision-free Robot Navigation
IEEE Transactions on Intelligent Vehicles(2024)
摘要
Collision avoidance is a crucial technique to achieve safe and efficient robotic vehicle navigation in unknown environments. However, moving obstacles with unpredictability in dynamic scenarios, usually increase the difficulty and complexity in collision avoidance of robotic vehicles. To enhance the stability of collision avoidance and boost its adaptability to uncertain dynamic scenes, a new attention-based value classification actor-critic (AVCAC) architecture is proposed. It is an end-to-end robot navigation model that utilizes imperfect local observation to directly plan accurate collision-free motion commands. First, we design a value-classified rollout replaybuffer to categorize the experiences into different pools. It can prevent any overfitting or bias that may result from repeatedly sampling experiences of a certain type during policy learning. Then, we improve the conventional actor-critic network with a multi-head local attention module to extract the local observations at entity-level. This way, the collision avoidance system can focus on key environmental features to operate more efficiently and respond more swiftly to dynamic changes in the environment. Moreover, a lookahead multi-step prediction (LMP) reward setting is devised in the AVCAC-based reinforcement learning (RL) framework to facilitate more informed and forward-looking decision-making. Finally, the policy entropy (PE) and policy delay (PD) are extended to AVCAC model to enhance policy exploration and make policy more robust. Extensive experimental results reveal that our method can generate time-efficient and collision-free guide paths to dodge collisions under complex dynamic environments.
更多查看译文
关键词
Robotic vehicle,collision-free navigation,reinforcement learning,value classification,local attention
AI 理解论文
溯源树
样例
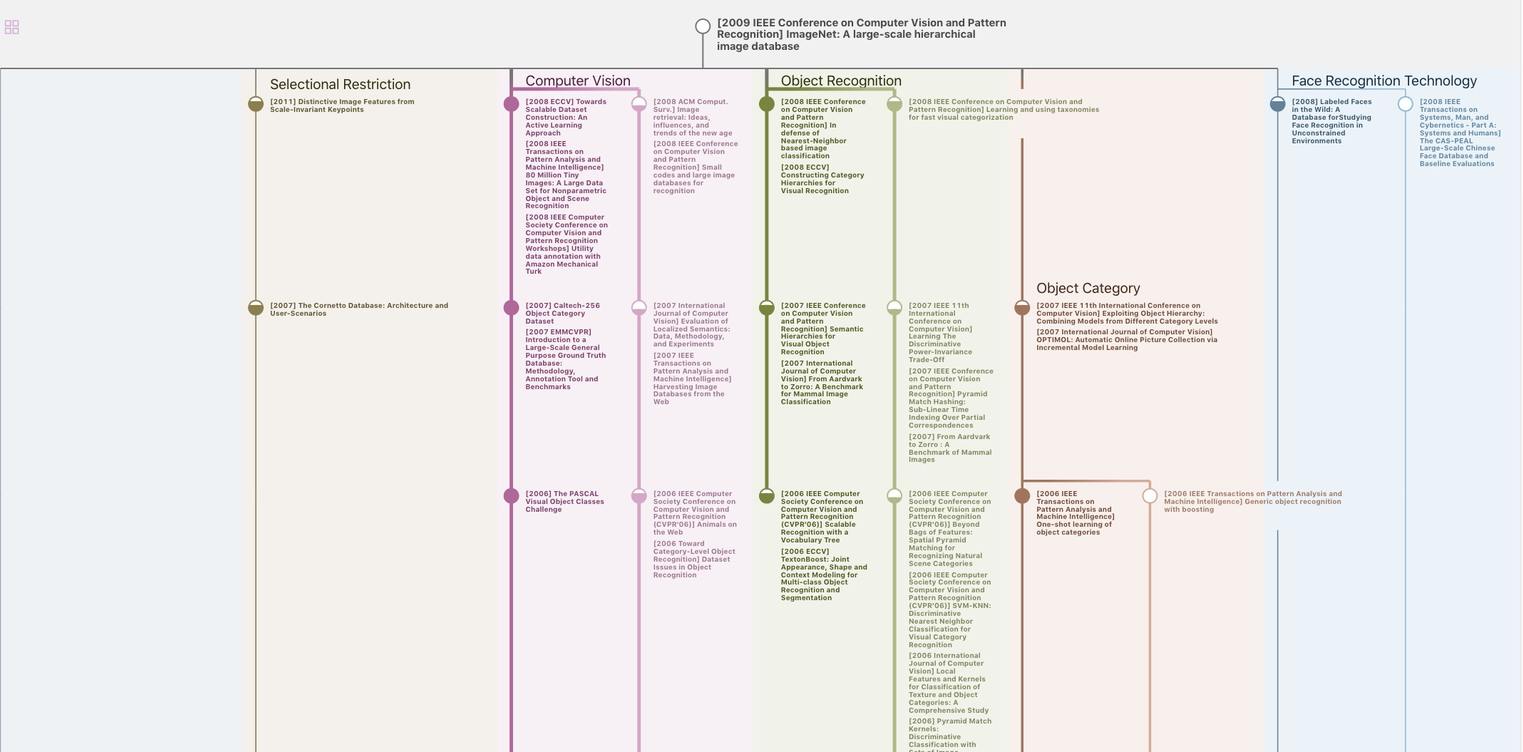
生成溯源树,研究论文发展脉络
Chat Paper
正在生成论文摘要