An Improved Particle Swarm Optimization Algorithm for P300 Neural Network Recognizer
ICCNS '23 Proceedings of the 2023 13th International Conference on Communication and Network Security(2024)
摘要
P300 is an important control system signal in the brain, so there is an urgent need and practical significance to work on the efficient classification of P300 event-related potentials. In this article, we design a convolutional neural network CNNnet based on chaotic adaptive particle swarm optimization (CAPSO) algorithm for efficient and accurate detection and classification of P300 EEG signals. The chaotic adaptive particle swarm optimization algorithm uses Logistic chaotic mapping to initialize the initial position of particles, and adopts a dynamic adaptive weighting strategy. Compared with traditional particle swarm optimization algorithms, it can effectively improve the optimization speed and convergence speed of particles. The experimental results show that compared with other P300 detection neural networks and traditional particle swarm optimization algorithms, this algorithm has faster convergence speed and higher convergence accuracy, and can effectively avoid the problem of particle swarm falling into local optima.
更多查看译文
关键词
Backpropagation Learning,Deep Learning,Machine Learning,Self-Organizing Maps,Feedforward Neural Networks
AI 理解论文
溯源树
样例
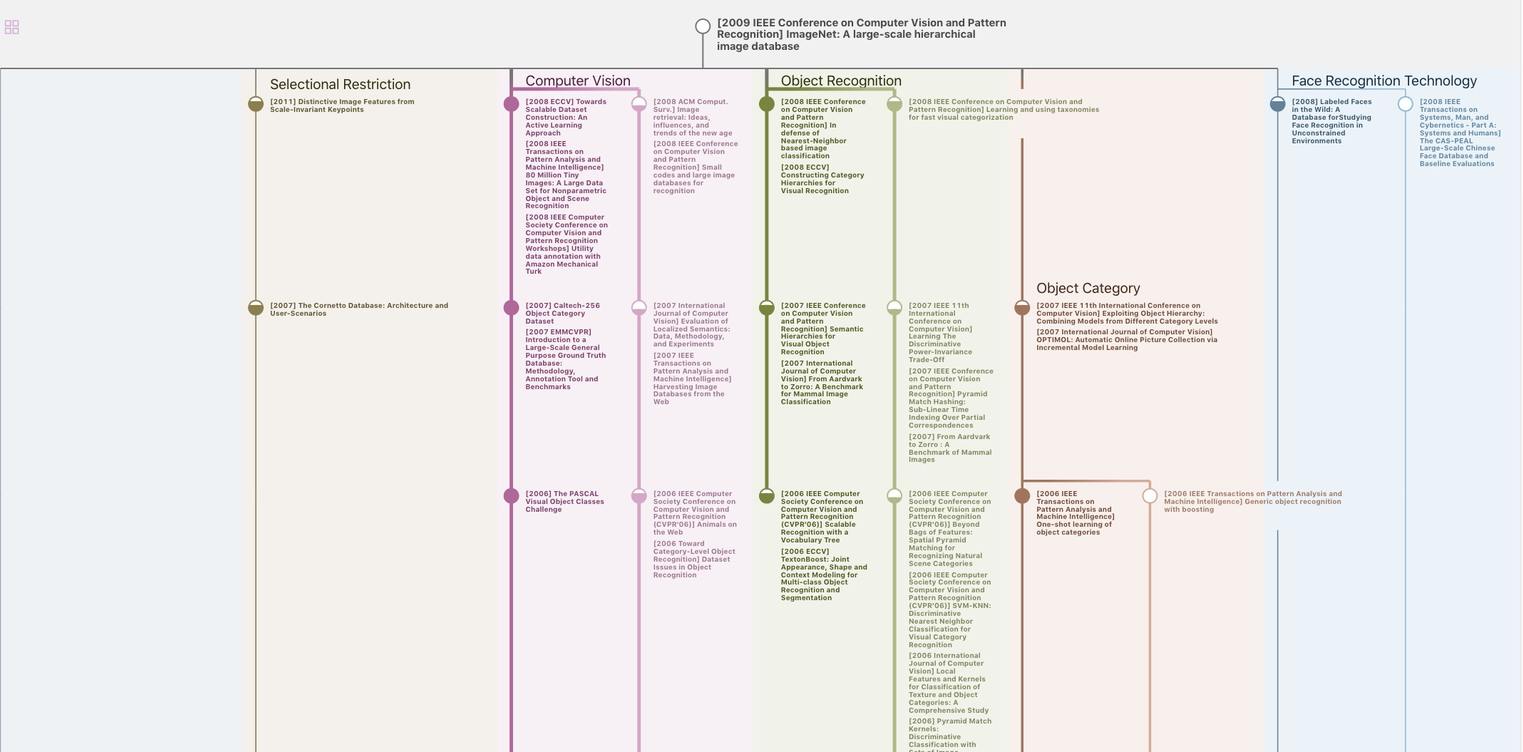
生成溯源树,研究论文发展脉络
Chat Paper
正在生成论文摘要