Fault Diagnosis for Power Batteries Based on a Stacked Sparse Autoencoder and a Convolutional Block Attention Capsule Network
Processes(2024)
摘要
The power battery constitutes the fundamental component of new energy vehicles. Rapid and accurate fault diagnosis of power batteries can effectively improve the safety and power performance of the vehicle. In response to the issues of limited generalization ability and suboptimal diagnostic accuracy observed in traditional power battery fault diagnosis models, this study proposes a fault diagnosis method utilizing a Convolutional Block Attention Capsule Network (CBAM-CapsNet) based on a stacked sparse autoencoder (SSAE). The reconstructed dataset is initially input into the SSAE model. Layer-by-layer greedy learning using unsupervised learning is employed, combining unsupervised learning methods with parameter updating and local fine-tuning to enhance visualization capabilities. The CBAM is then integrated into the CapsNet, which not only mitigates the effect of noise on the SSAE but also improves the model’s ability to characterize power cell features, completing the fault diagnosis process. The experimental comparison results show that the proposed method can diagnose power battery failure modes with an accuracy of 96.86%, and various evaluation indexes are superior to CNN, CapsNet, CBAM-CapsNet, and other neural networks at accurately identifying fault types with higher diagnostic accuracy and robustness.
更多查看译文
关键词
power battery,stacked sparse autoencoder,convolutional block attention capsule network,fault diagnosis
AI 理解论文
溯源树
样例
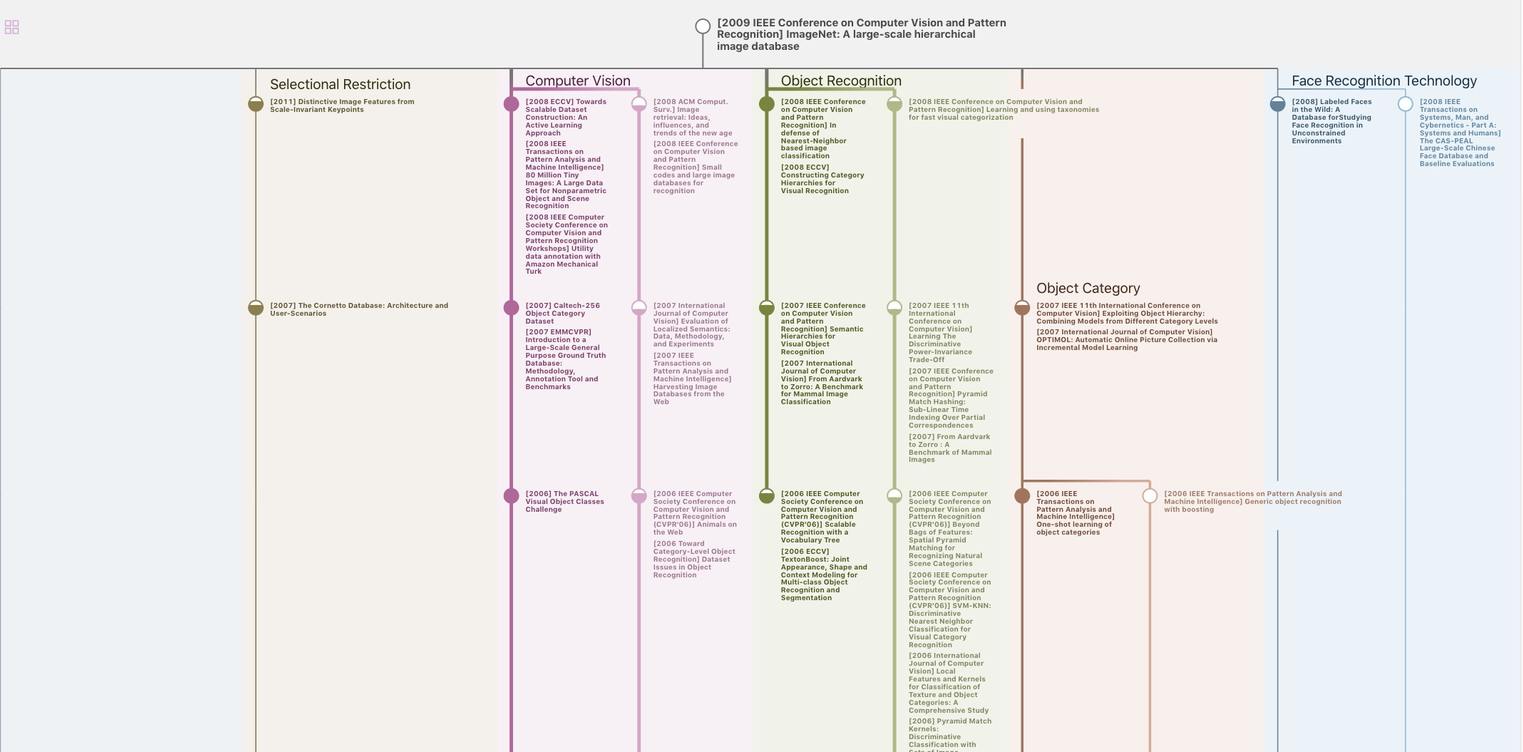
生成溯源树,研究论文发展脉络
Chat Paper
正在生成论文摘要