A Novel Framework for Risk Warning That Utilizes an Improved Generative Adversarial Network and Categorical Boosting
Electronics(2024)
摘要
To address the problems of inadequate training and low precision in prediction models with small-sample-size and incomplete data, a novel SALGAN-CatBoost-SSAGA framework is proposed in this paper. We utilize the standard K-nearest neighbor algorithm to interpolate missing values in incomplete data, and employ EllipticEnvelope to identify outliers. SALGAN, a generative adversarial network with a self-attention mechanism of label awareness, is utilized to generate virtual samples and increase the diversity of the training data for model training. To avoid local optima and improve the accuracy and stability of the standard CatBoost prediction model, an improved Sparrow Search Algorithm (SSA)–Genetic Algorithm (GA) combination is adopted to construct an effective CatBoost-SSAGA model for risk warning, in which the SSAGA is used for the global parameter optimization of CatBoost. A UCI heart disease dataset is used for heart disease risk prediction. The experimental results show the superiority of the proposed model in terms of accuracy, precision, recall, and F1-values, as well as the AUC.
更多查看译文
关键词
small-sample datasets,data augmentation,improved sparrow search algorithm,novel risk warning,GAN
AI 理解论文
溯源树
样例
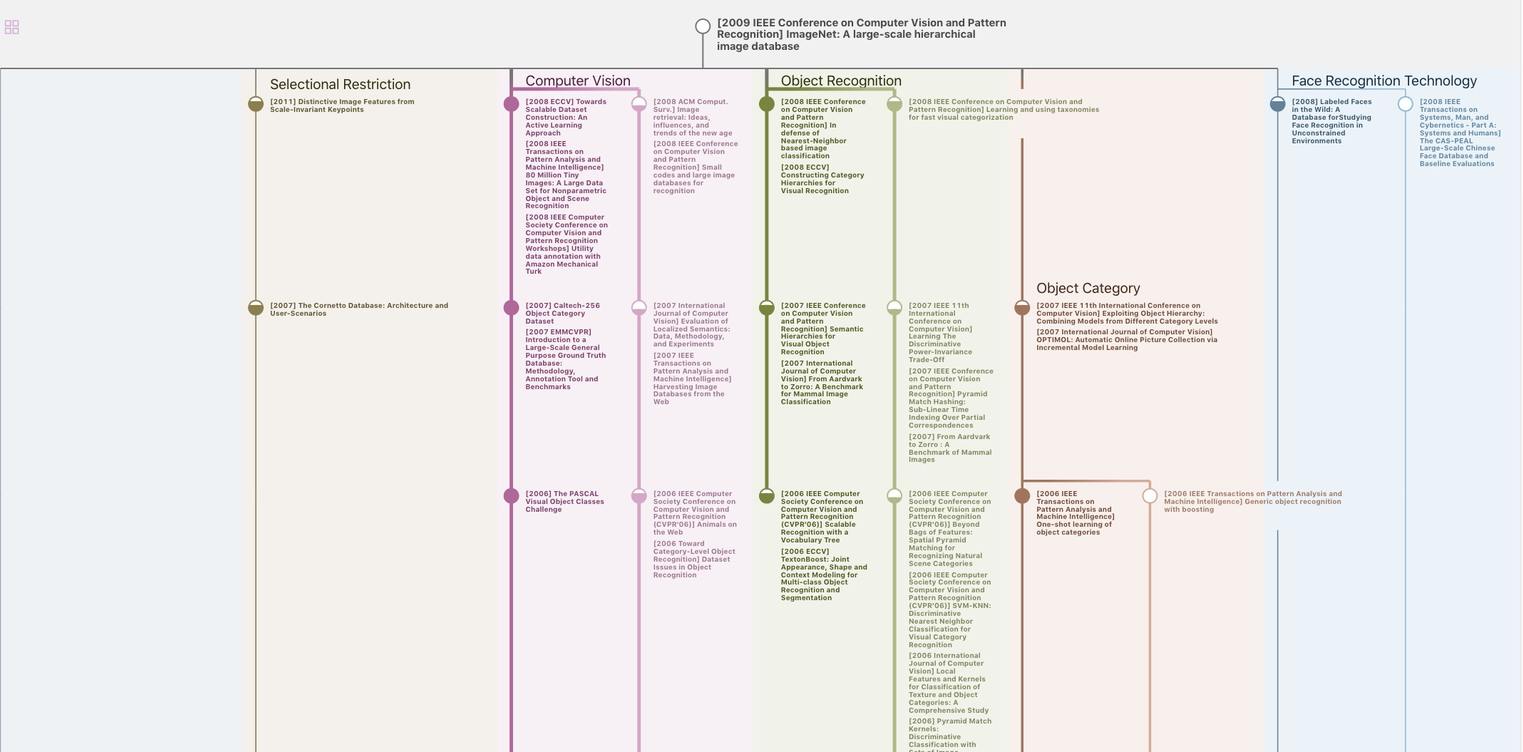
生成溯源树,研究论文发展脉络
Chat Paper
正在生成论文摘要