Flashlight-Net: A Modular Convolutional Neural Network for Motor Imagery EEG Classification
IEEE Transactions on Systems, Man, and Cybernetics: Systems(2024)
摘要
Brain-computer interface (BCI) establishes an interactive platform by translating brain activity patterns into commands of external devices. BCIs, especially motor imagery (MI)-based BCIs, have injected new vitality into the development of rehabilitation medicine and many other fields. In this work, one convolutional neural network, named as Flashlight-Net model, is proposed for multiclass MI classification. Flashlight-Net model adopts modular design, in which channel fusion module and time domain module ensure the directions of feature extraction, while feature pool module reduces the loss of effective information. Given the multi-frequency nature of the brain, we combine three frequency bands and construct an ensemble Flashlight-Net model. During the model training, by means of transfer learning, pretraining and fine-tuning processes are designed to integrate training samples from multiple subjects. The experimental results on publicly available BCI Competition IV-2a dataset show that the proposed model can achieve good results on all nine subjects, with an average classification accuracy of 81.23% for four classes. All these demonstrate that the proposed Flashlight-Net model can effectively decode multi-channel and multiclass MI signals.
更多查看译文
关键词
Brain-computer interface (BCI),convolutional neural network (CNN),electroencephalogram (EEG) signals,motor imagery (MI),transfer learning
AI 理解论文
溯源树
样例
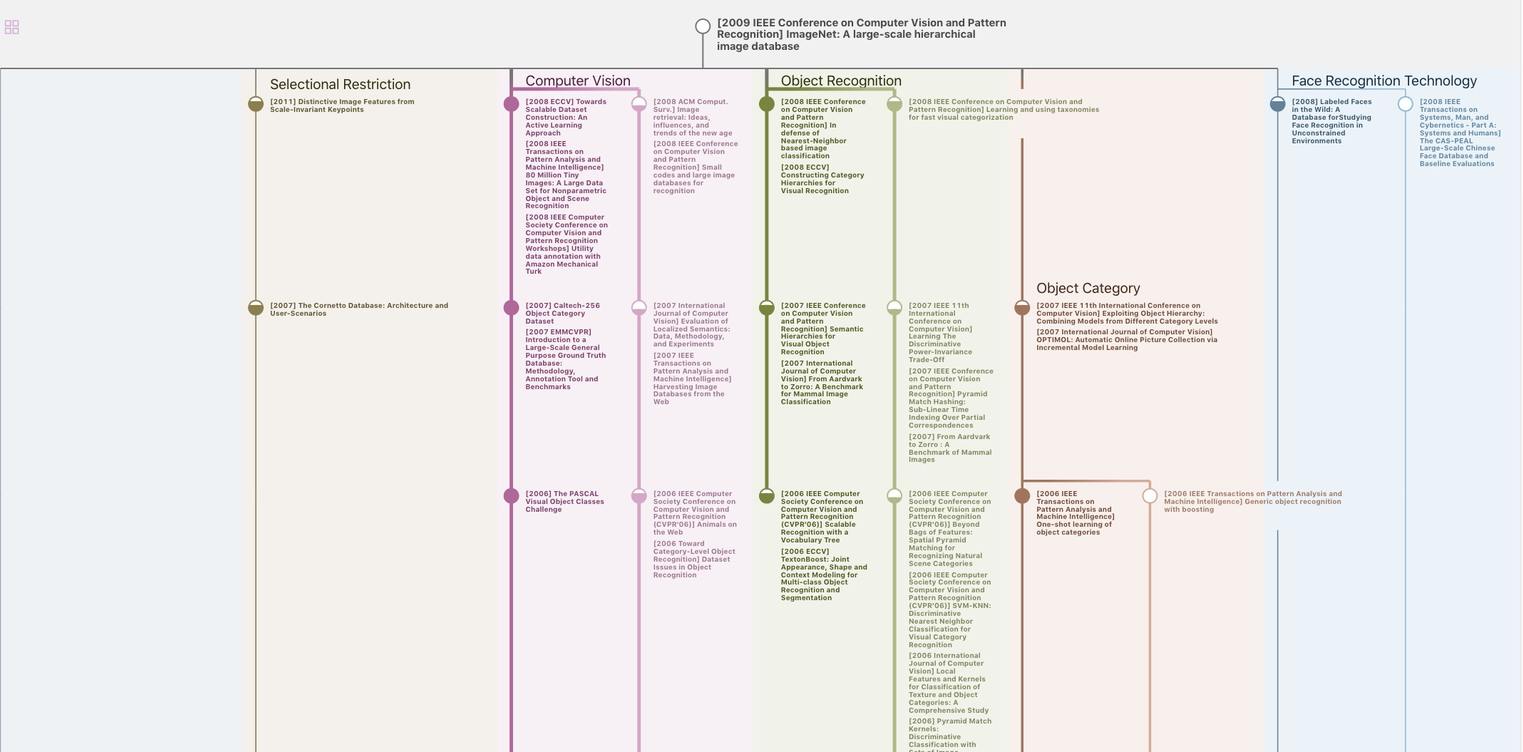
生成溯源树,研究论文发展脉络
Chat Paper
正在生成论文摘要