Predictive active control of building structures using LQR and artificial intelligence
Earthquake Engineering and Engineering Vibration(2024)
摘要
This study presents a neural network-based model for predicting linear quadratic regulator (LQR) weighting matrices for achieving a target response reduction. Based on the expected weighting matrices, the LQR algorithm is used to determine the various responses of the structure. The responses are determined by numerically analyzing the governing equation of motion using the state-space approach. For training a neural network, four input parameters are considered: the time history of the ground motion, the percentage reduction in lateral displacement, lateral velocity, and lateral acceleration, Output parameters are LQR weighting matrices. To study the effectiveness of an LQR-based neural network (LQRNN), the actual percentage reduction in the responses obtained from using LQRNN is compared with the target percentage reductions. Furthermore, to investigate the efficacy of an active control system using LQRNN, the controlled responses of a system are compared to the corresponding uncontrolled responses. The trained neural network effectively predicts weighting parameters that can provide a percentage reduction in displacement, velocity, and acceleration close to the target percentage reduction. Based on the simulation study, it can be concluded that significant response reductions are observed in the active-controlled system using LQRNN. Moreover, the LQRNN algorithm can replace conventional LQR control with the use of an active control system.
更多查看译文
关键词
active control system,linear quadratic regulator,artificial neural networks,state-space approach,response effectiveness factor,resilience
AI 理解论文
溯源树
样例
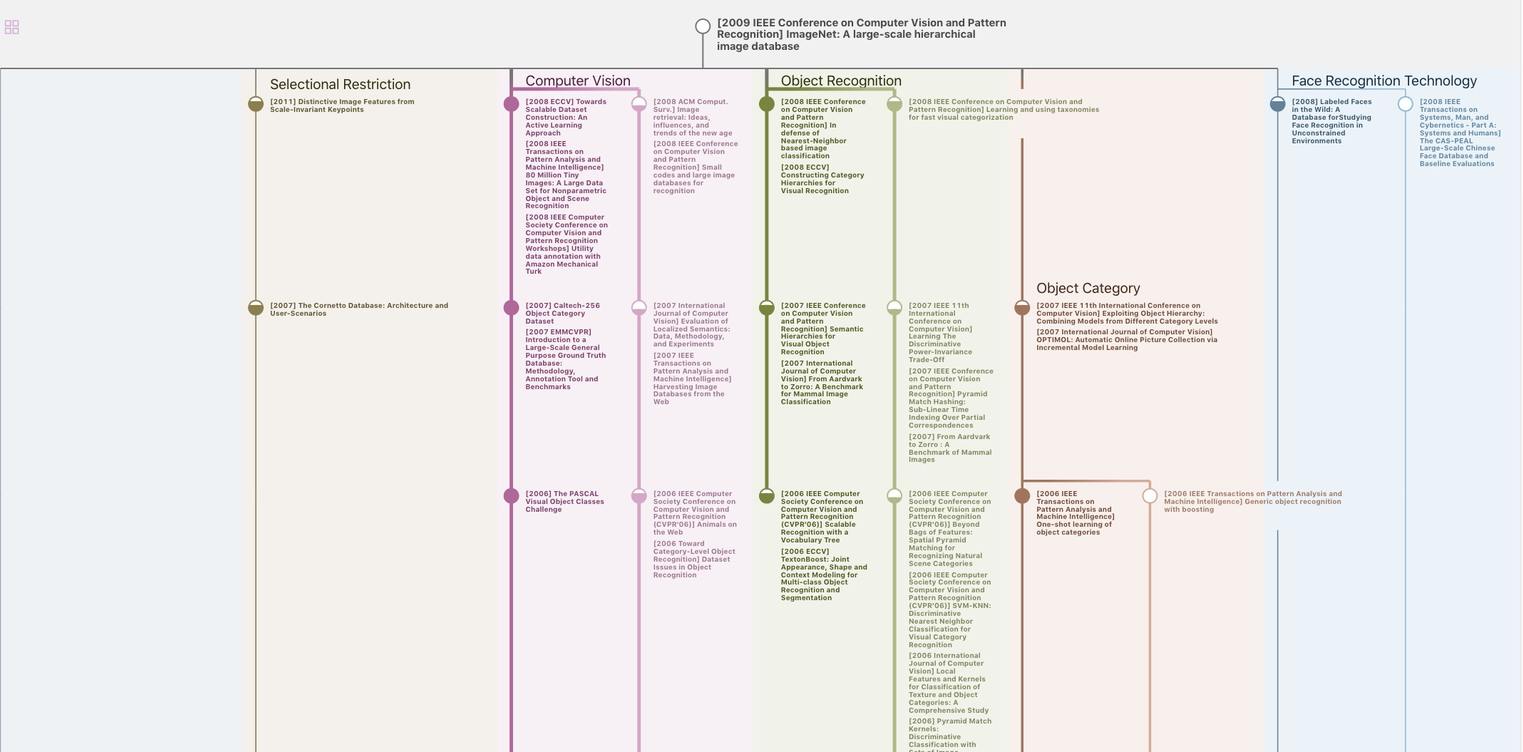
生成溯源树,研究论文发展脉络
Chat Paper
正在生成论文摘要