PIGAT: Physics-Informed Graph Attention Transformer for Air Traffic State Prediction
IEEE Transactions on Intelligent Transportation Systems(2024)
摘要
Efficient and resilient traffic management relies on accurate prediction of air traffic states. However, the complex spatial-temporal dependencies of air traffic networks make this task challenging. To address this issue, we propose a novel deep learning framework, named Physics-Informed Graph Attention Transformer (), which leverages real-world data and knowledge to predict essential air traffic state parameters. Our approach utilizes fine-grained traffic state detection to extract critical features from aviation databases. The model employs GAT-based spatial learning blocks with temporal Transformers to capture the dynamic spatial-temporal dependencies of data. A dynamic graph generator layer is also utilized to update the airport network’s topological structure adaptively, strengthening the model prediction’s effectiveness. Furthermore, the fluid queuing-theoretic PDEs are incorporated into the loss function, enhancing the model’s interpretability and reliability. Our framework is evaluated on real-world air traffic datasets from 36 major airport hubs within the US. Experimental results demonstrate that our proposed framework efficiently makes accurate predictions and outperforms eight baselines. In conclusion, our proposed framework has the potential to be applied in real-time decision-making systems for air traffic management and provides promising directions for future research. The code for our project is available at: https://github.com/ymlasu/para-atm-collection/tree/master/air-traffic-prediction/PIGAT.
更多查看译文
关键词
Air traffic management,spatial-temporal learning,dynamic graph attention network,physics-informed
AI 理解论文
溯源树
样例
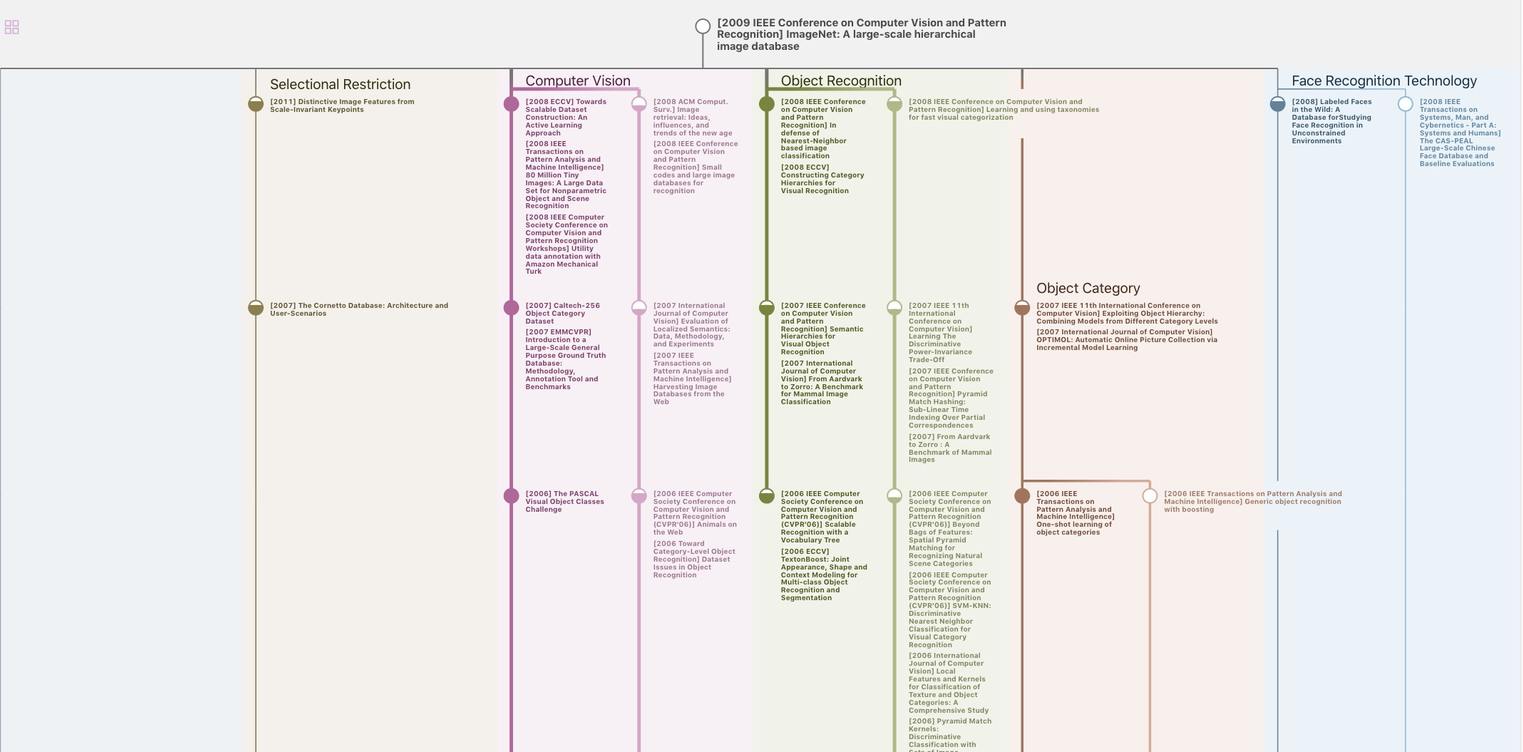
生成溯源树,研究论文发展脉络
Chat Paper
正在生成论文摘要