Residual deep fuzzy system with randomized fuzzy modules for accurate time series forecasting
Neural Computing and Applications(2024)
摘要
The data-driven modular deep fuzzy model has demonstrated excellent forecasting performance due to its clear architecture and powerful fuzzy inference ability. However, the fixed structure predesigned for specific types of datasets limits the further improvement of generalization ability, especially when facing high-dimensional datasets with the challenging problem of rule explosion, thereby suffering from the time-consuming training process. To overcome these limitations, a novel randomized fuzzy modules (RFMs) stacked residual deep fuzzy system (RFM-RDFS) is proposed in this study. Firstly, to obtain high-precision outputs, this paper presents a residual deep fuzzy framework by stacking RFMs layer by layer, where the modules in the current layer only learn the residual of the previous layer. Secondly, to improve the design freedom for different applications, the RFM with multiple inputs is raised, where the input variables are allocated by a sliding window along the input spaces of the layers, so the number of the parallel modules in the same layer can be flexibly adjusted. Meanwhile, in the RFM, inspired by the extreme learning machine, the centers and widths of Gaussian membership functions are randomly generated, which can greatly accelerate the training speed. Thirdly, to strengthen the approximation performance, the consequent parameters of the fuzzy rules in each RFM are learned by minimizing the loss function with the regularization terms through the ridge regression algorithm. Finally, extensive experiments on various datasets are conducted to compare the proposed RFM-RDFS with several state-of-the-art shallow and deep models. The detailed comparison results clearly show that the proposed RFM-RDFS is more flexible and compact, and has greater advantages in terms of the interpretability, generalization ability and robustness.
更多查看译文
关键词
Fuzzy systems,Residual approximation,Randomized fuzzy module,Forecasting
AI 理解论文
溯源树
样例
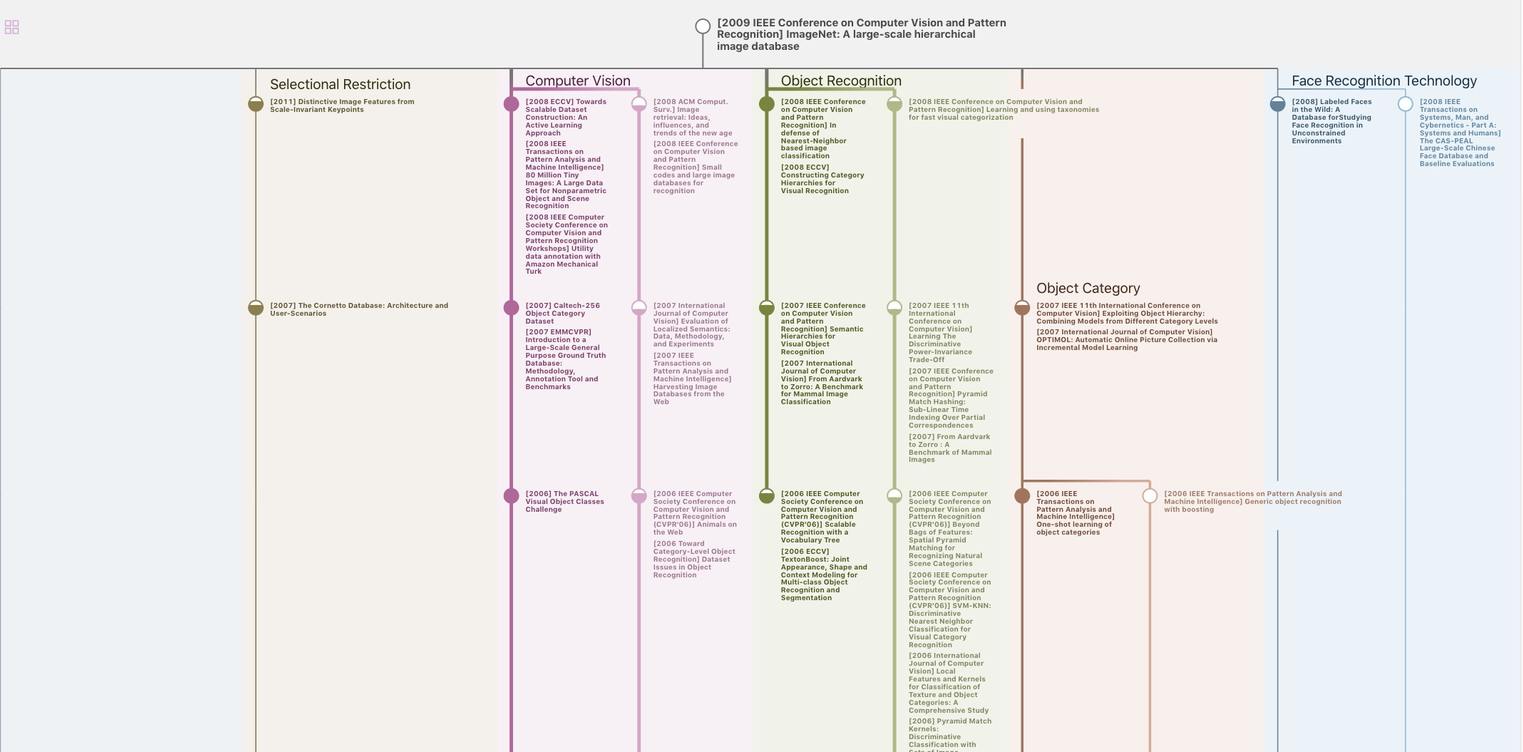
生成溯源树,研究论文发展脉络
Chat Paper
正在生成论文摘要