Toward Explainable End-to-End Driving Models Via Simplified Objectification Constraints
IEEE Transactions on Intelligent Transportation Systems(2024)
摘要
The end-to-end driving models (E2EDMs) convert environmental information into driving actions using a complex transformation which makes E2EDMs have high prediction accuracy. Due to the black-box nature of transformation, the E2EDMs have low explainability. To solve this problem, explanation methods are used to generate explanations for observation. Based on current explanation methods, previous studies tried to further improve the explainability of E2EDMs by integrating an object detection module, however, these methods have many problems: Firstly, due to the requirement of the object detection module, they lack flexibility. Secondly, they neglect an essential property, i.e. , simplicity, to improve explainability. In this paper, since humans prefer object-level and simple explanations in driving tasks, we argue that explainability is decided by two properties which are the objectification degree (the extent to which driving related-object features are utilized) and simplification degree (the simplicity of the explanation), thus we propose Simplified Objectification Branches (SOB) to improve the explainability of E2EDMs. Firstly, this structure could be integrated into any existing E2EDMs and thus have high flexibility. Secondly, the SOB explicitly improves the simplification degree without sacrificing the objectification degree of the explanations. By designing several indicators, i.e. , heatmap satisfaction, driving action reproduction score, deception level, etc. , we proved that SOB could help E2EDMs generate better explanations. Notably, the SOB could also further enhance E2EDMs’ prediction accuracy.
更多查看译文
关键词
Explainability,autonomous vehicles,deep learning,convolutional neural networks
AI 理解论文
溯源树
样例
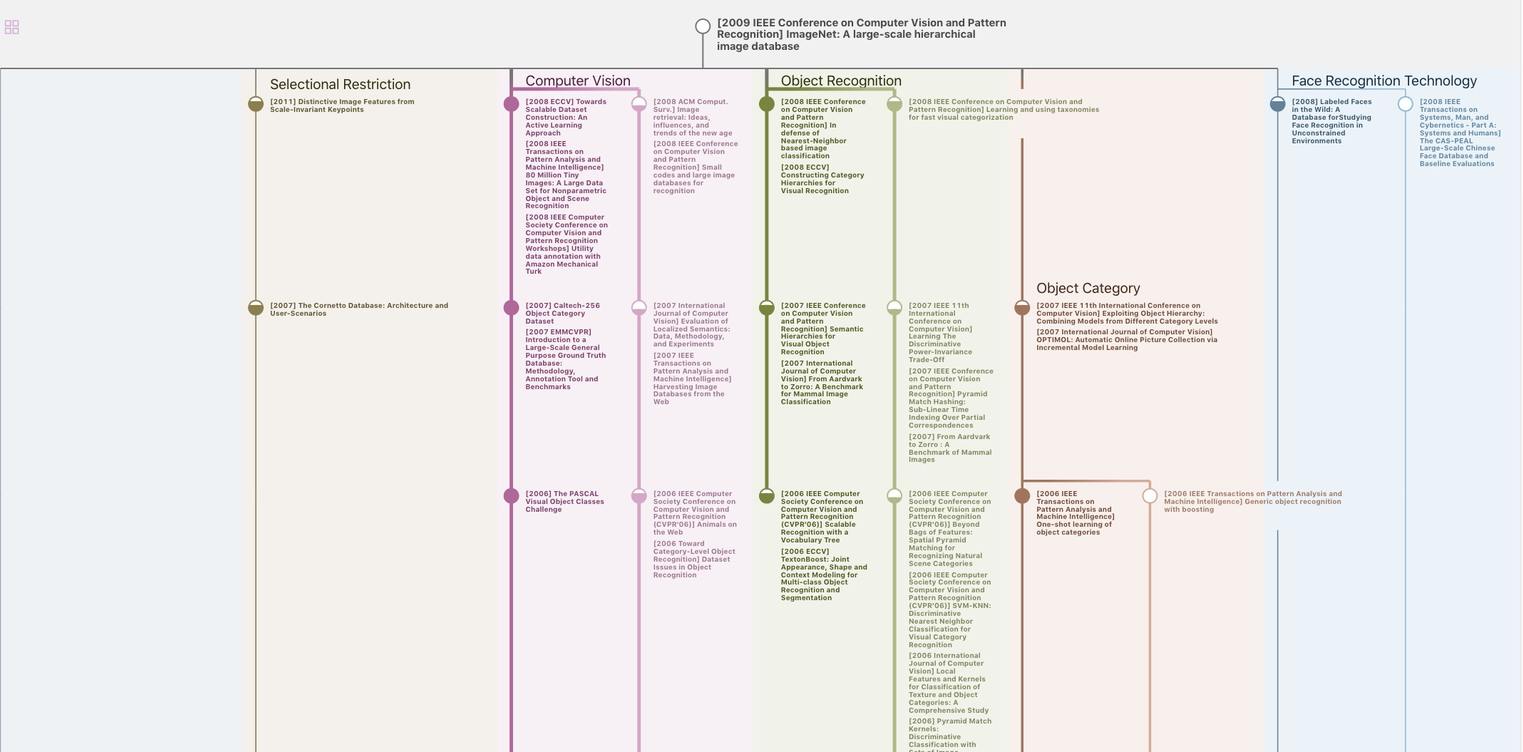
生成溯源树,研究论文发展脉络
Chat Paper
正在生成论文摘要