Graph Neural Networks with Selective Attention and Path Reasoning for Document-Level Relation Extraction
Applied intelligence(2024)
摘要
Document-level Relation Extraction (DocRE) aims to extract relations from multiple sentences simultaneously. Existing graph-based methods adopt static graphs to represent the document structure, which is unable to capture complex interactions. Besides, they take all sentences in the document as the scope of relation extraction (RE) while introducing noise by irrelevant sentences. Furthermore, they do not explicitly model the reasoning chain, leading to a lack of explainability in the reasoning results. These limitations may significantly hinder their performance in practical applications. In this paper, we propose a model based on selective attention and path reasoning for DocRE. Firstly, we adopt hierarchical heterogeneous graph neural networks and recurrent neural networks to realize document modeling and capture complex interactions in the document. Secondly, we adopt selective attention to select sentences related to the entity pair to generate document subgraphs as the scope of RE. Lastly, we adopt path reasoning to explicitly model the reasoning chain between multiple entities in the document subgraph, infer the relations between entities and provide corresponding supporting evidence. Extensive experiment results on three benchmark datasets show that the proposed framework is effective and achieves superior performance compared to most methods. Further analysis demonstrates that selective attention and path reasoning can discover more accurate inter-sentence relations and supporting evidence.
更多查看译文
关键词
Graph neural network,Selective attention,Path reasoning,Document-level relation extraction,Supporting evidence
AI 理解论文
溯源树
样例
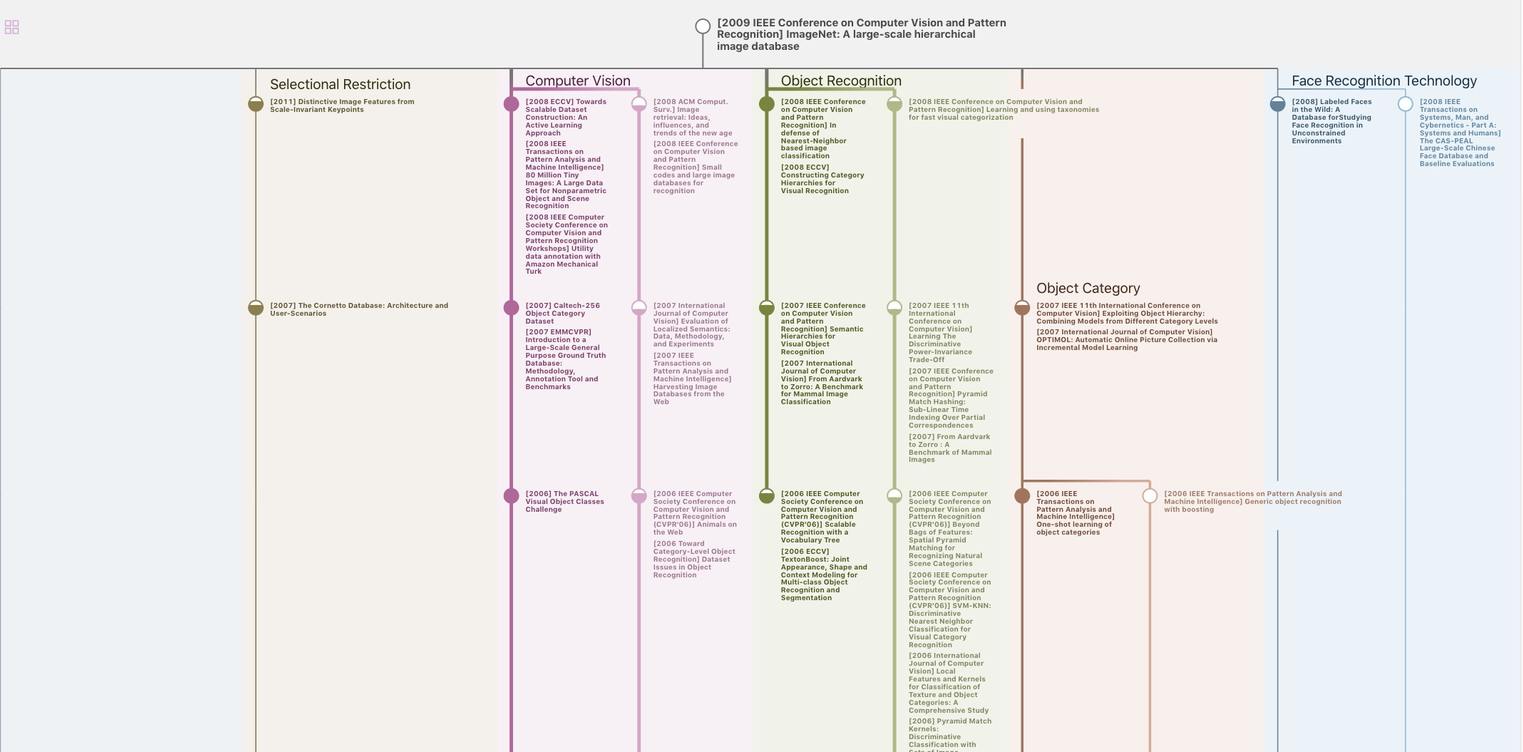
生成溯源树,研究论文发展脉络
Chat Paper
正在生成论文摘要