Accelerating sampling-based optimal path planning via adaptive informed sampling
Autonomous Robots(2024)
摘要
This paper improves the performance of RRT^* -like sampling-based path planners by combining admissible informed sampling and local sampling (i.e., sampling the neighborhood of the current solution). An adaptive strategy regulates the trade-off between exploration (admissible informed sampling) and exploitation (local sampling) based on online reward from previous samples. The paper demonstrates that the resulting algorithm is asymptotically optimal and has a better convergence rate than state-of-the-art path planners (e.g., Informed-RRT ^* ) in several simulated and real-world scenarios. An open-source, ROS-compatible implementation of the algorithm is publicly available.
更多查看译文
关键词
Motion planning,Sampling-based algorithms,Informed sampling,Optimal path planning,Informed-RRT*
AI 理解论文
溯源树
样例
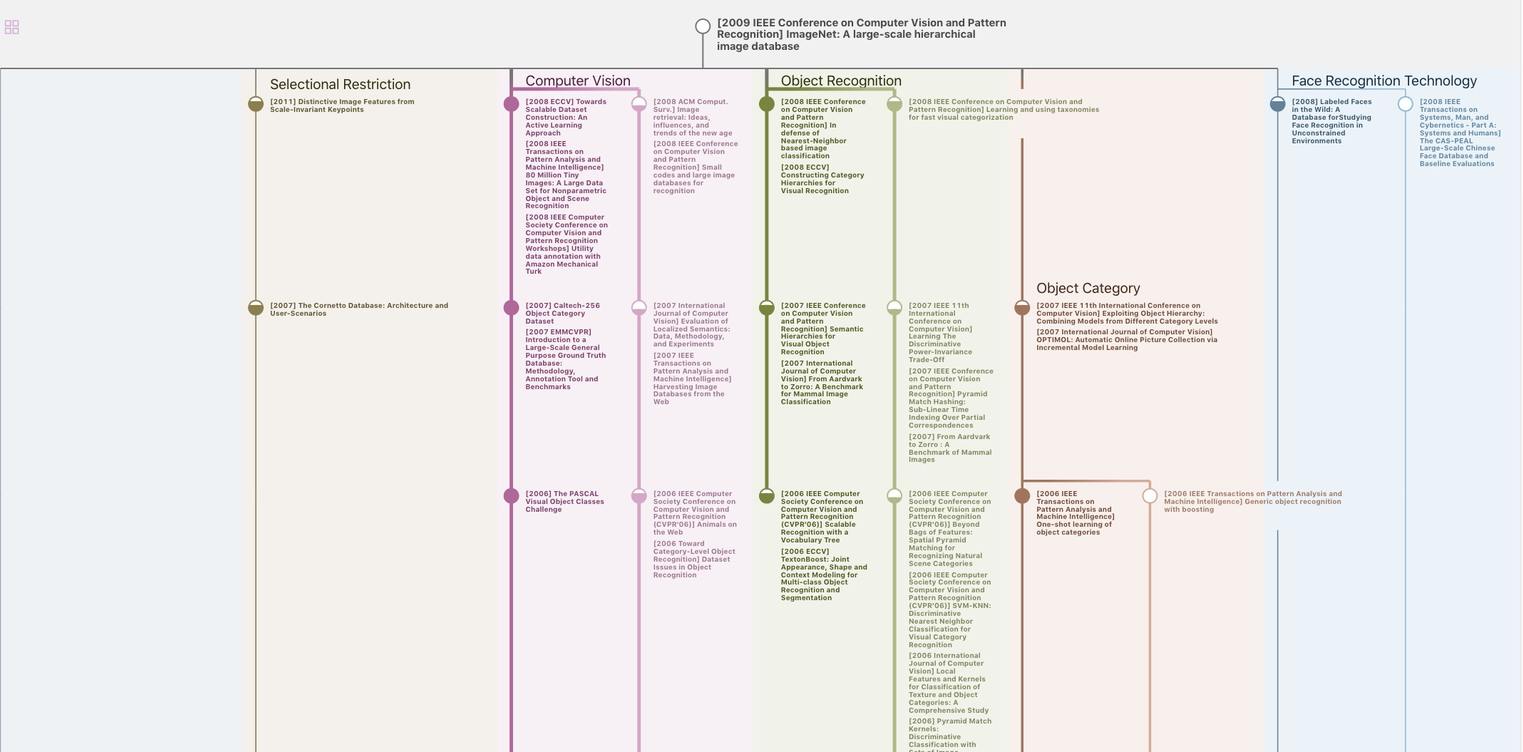
生成溯源树,研究论文发展脉络
Chat Paper
正在生成论文摘要