A reservoir computing model based on nonlinear spiking neural P systems for time series forecasting
Applied Soft Computing(2024)
摘要
Nonlinear spiking neural P (NSNP) systems are a novel class of neural-like computing models that exhibit nonlinear dynamic behaviours. Further, reservoir computing (RC) is a new type of recurrent neural network (RNN) whose dynamic behaviour is provided by a reservoir of neurones. An interesting question is whether NSNP systems can be embedded into an RC framework to develop an efficient prediction model for time series forecasting problems. To address this problem, this paper first defines a variant of NSNP systems with two outputs, called NSNP-TO systems, in which a nonlinear spiking mechanism with two outputs is the key feature. Based on NSNP-TO systems, a new variant of RC models is proposed based on NSNP systems, called the RC-NSNP model. Owing to the use of spiking neurones, the RC-NSNP model comprises state-updating and output equations that are different from those of the existing RC models. Using a similar structure, the proposed RC-NSNP model can be implemented in the RC framework, and a similar training method can be utilised to train it. Time series forecasting tasks are performed to verify the effectiveness of the proposed RC-NSNP model. The proposed RC-NSNP model achieved the best performance on nine of the twelve time series problems and obtained the second-best performance on the other two time series.
更多查看译文
关键词
Spiking neural P systems,Artificial neural networks,Reservoir computing,Time series
AI 理解论文
溯源树
样例
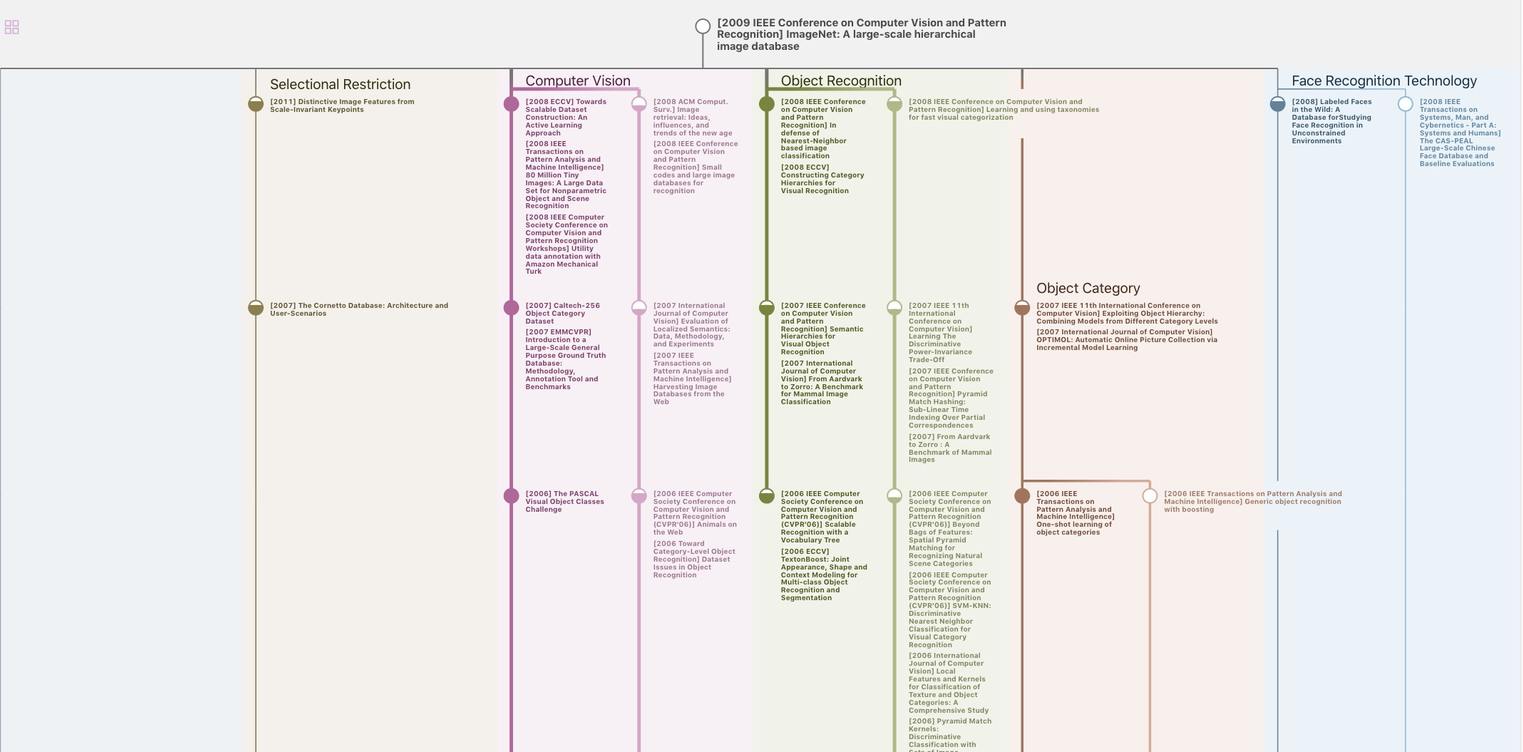
生成溯源树,研究论文发展脉络
Chat Paper
正在生成论文摘要