Deep Learning-based forgery detection and localization for compressed images using a hybrid optimization model
Multimedia Systems(2024)
摘要
Manipulation of digital images has become quite common in recent years because of the rise of various image editing tools. It has become a challenging task to identify authentic and tampered images, since tampered images are non-distinguishable by the naked eye. Hence, different approaches are used for the identification of tampered and authentic images. However, conventional techniques are considered to be inefficient in terms of identifying the tampered images effectively. Therefore, DL (Deep Learning)-based approaches are used for detecting the authentic and tampered images as DL techniques deliver improved accuracy and better automated FE (feature extraction) skills which help in achieving the desired outcomes. Moreover, neural networks can extract complex hidden features of the images, thus providing better accuracy of the model. Therefore, the proposed model utilizes DL-based approaches for effective feature optimization and classification of authentic and tampered images by employing the proposed EfficientNet model and by incorporating QF (quality factors) into images. In the proposed feature optimization, SPOA (Seagull Pelican Optimization Algorithm) is used for diminishing the number of features which drops the computational complexity and aids in refining the performance of the proposed framework by selecting the relevant and suitable features from the accessible data. Further, in the proposed EfficientNet model, CDT (Cosine Disintegration Tempering) and TAS (Ternary Attention Structure) are incorporated for classification, where CDT aids the proposed model in learning discriminant features and helps in preventing the model from getting overfitted on the training dataset using assimilate rate adopted scheduling and TAS (Ternary Attention Structure) utilized in MBConv possess the capability to capture both channel attention information and spatial attention information, thereby making the model efficient and effective for classification of authentic and tampered images. The proposed work employs CASIA 1.0 and CASIA 2.0 dataset for classification. Eventually, the proposed work utilizes different performance metrics for assessing the efficacy of the model and comparing it with the prevailing models for calculating the effectiveness of the proposed model.
更多查看译文
关键词
Forgery detection,Scale Invariant Feature Transform (SIFT),Speeded Up Robust Features (SURF),Pelican Optimization Algorithm (POA),Seagull Optimization Algorithm (SOA),EfficientNet
AI 理解论文
溯源树
样例
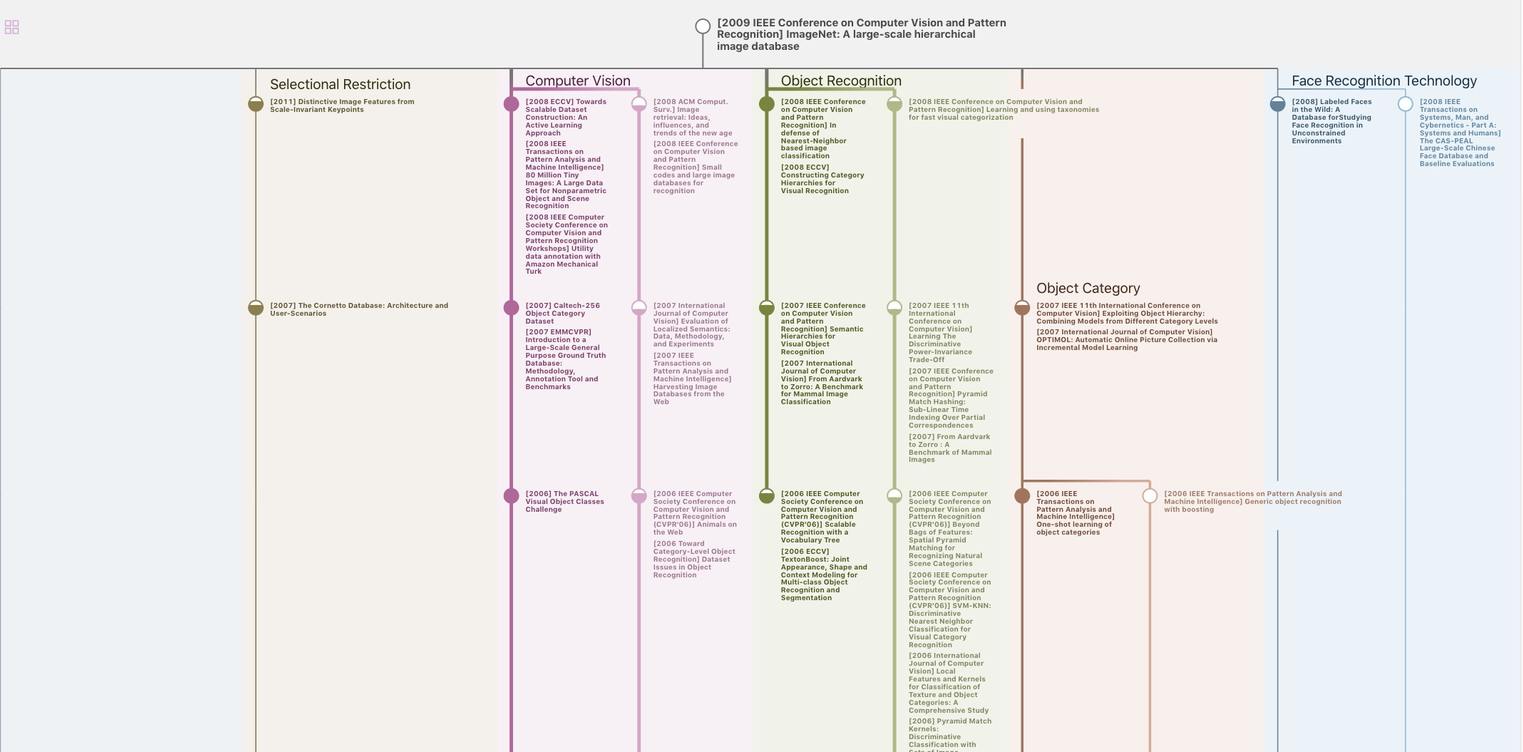
生成溯源树,研究论文发展脉络
Chat Paper
正在生成论文摘要