Data Authenticity, Consent, Provenance for AI are all broken: what will it take to fix them?
arxiv(2024)
摘要
New capabilities in foundation models are owed in large part to massive,
widely-sourced, and under-documented training data collections. Existing
practices in data collection have led to challenges in documenting data
transparency, tracing authenticity, verifying consent, privacy, representation,
bias, copyright infringement, and the overall development of ethical and
trustworthy foundation models. In response, regulation is emphasizing the need
for training data transparency to understand foundation models' limitations.
Based on a large-scale analysis of the foundation model training data landscape
and existing solutions, we identify the missing infrastructure to facilitate
responsible foundation model development practices. We examine the current
shortcomings of common tools for tracing data authenticity, consent, and
documentation, and outline how policymakers, developers, and data creators can
facilitate responsible foundation model development by adopting universal data
provenance standards.
更多查看译文
AI 理解论文
溯源树
样例
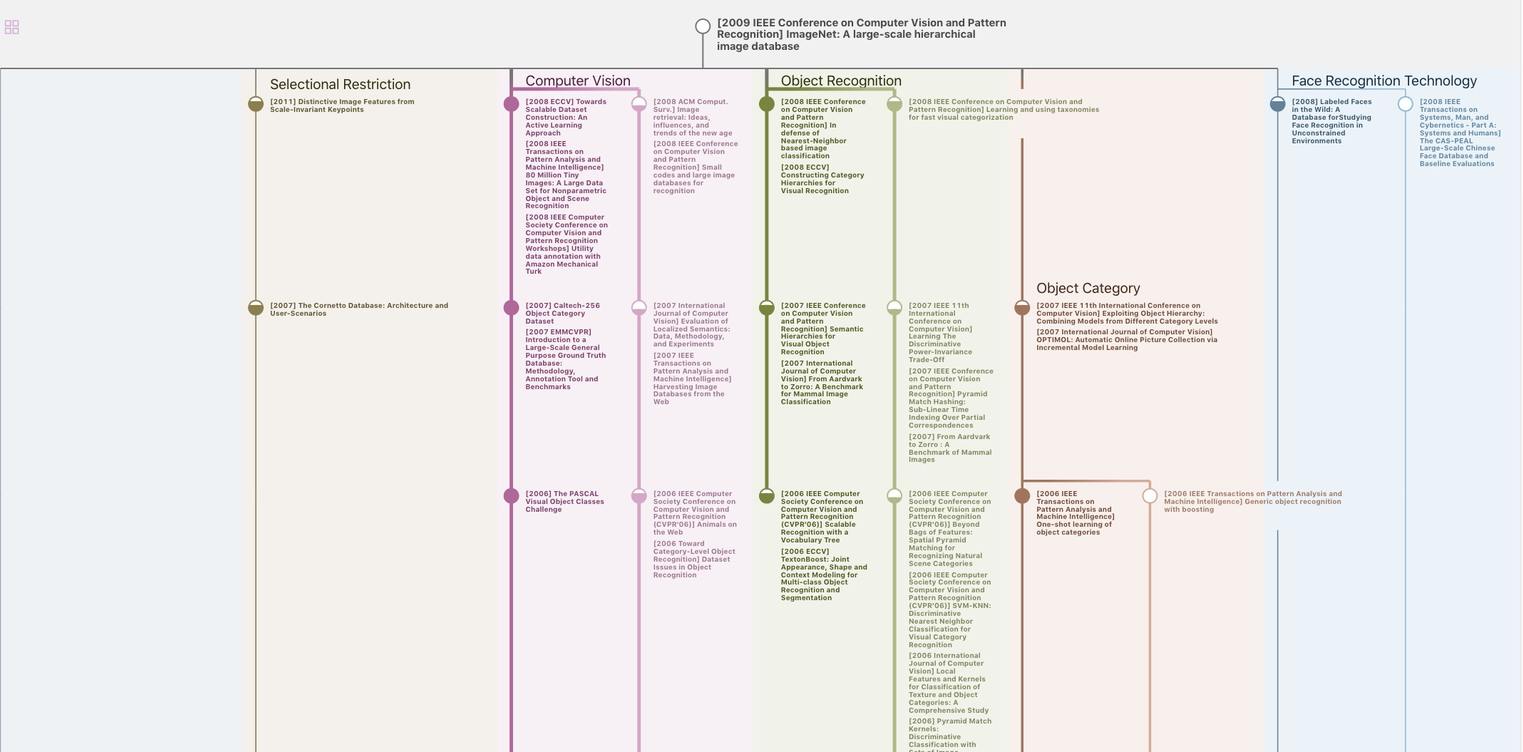
生成溯源树,研究论文发展脉络
Chat Paper
正在生成论文摘要