A SOM-LSTM combined model for groundwater level prediction in karst critical zone aquifers considering connectivity characteristics
Environmental Earth Sciences(2024)
摘要
Accurate groundwater level (GWL) prediction is crucial for the management and sustainable utilization of groundwater resources. This study proposes a method, considering spatial–temporal correlation among geographic multi-feature in data, and Self-Organizing Map (SOM)-based clustering technique to identify and partition spatially connectivity among observation wells. Finally, based on the connectivity results, the observation well dataset is determined as inputs to LSTM for GWL prediction. This approach provides a new idea to enhance the accuracy of existing data-driven methods in karst critical zones characterized by significant spatial heterogeneity in GWL. Comparing with prediction models that solely consider internal data correlations, experiments are conducted in the typical highly spatially heterogeneous karst critical zone of Jinan City, Shandong Province, China. The results show a significant improvement in prediction accuracy when considering spatial connectivity between observation wells based on geographical multi-feature spatial–temporal correlation. Confirming that considering the spatial connectivity of observation wells in GWL prediction methods are more accurate, particularly in areas with significant spatial heterogeneity in karst aquifers.
更多查看译文
关键词
Karst,Groundwater statistics,Geographic multi-feature,Spatial–temporal correlation,SOM-LSTM model
AI 理解论文
溯源树
样例
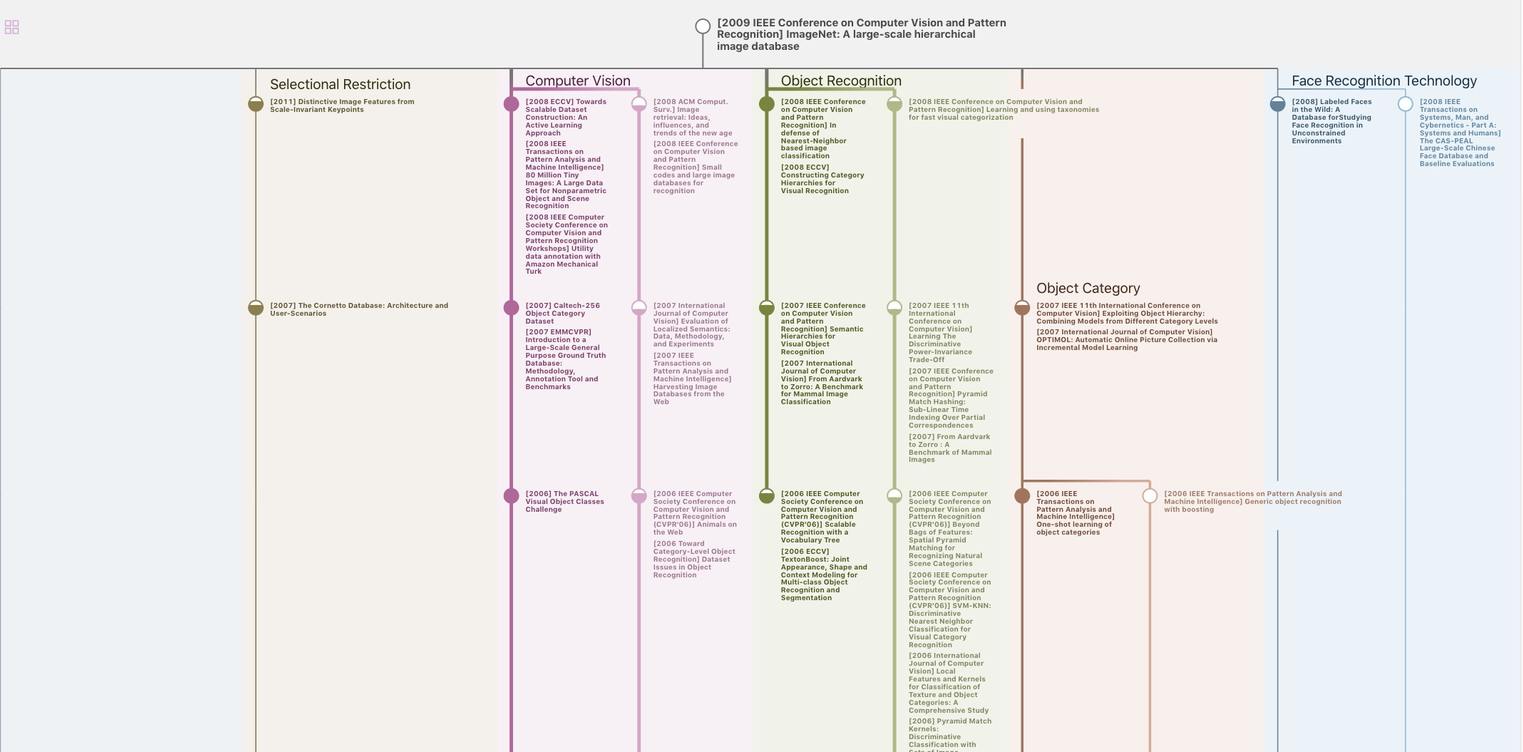
生成溯源树,研究论文发展脉络
Chat Paper
正在生成论文摘要