RSTFusion: an end-to-end fusion network for infrared and visible images based on residual swin transfomer
Neural Computing and Applications(2024)
摘要
Infrared and visible image fusion techniques have emerged as powerful methods to harness the unique advantages of diverse sensors, resulting in improved image quality through the preservation of complementary and redundant information from the original images. While deep learning-based methods have gained widespread adoption in this domain, their predominant reliance on convolutional neural networks and limited utilization of transforms pose certain limitations. Notably, convolutional operations fail to effectively capture long-range dependency between images, which hampers the generation of fused images with optimal complementarities. Because of this, we provide an original end-to-end fusion model built on the swin transform. By modeling long-range dependency using a full-attention feature-encoding backbone, which is a pure transform network with stronger representational capabilities than convolutional neural networks, the model overcomes the shortcomings of manual design as well as complex activity-level measurement and fusion rule design. In addition, we present three loss function strategies that are created expressly to enhance similarity constraints and network parameter training, improving the quality of the detailed information in the fused images. Finally, experimental results on four datasets indicated that our method reached the state-of-the-art in both subjective and objective evaluation compared to eleven state-of-the-art methods.
更多查看译文
关键词
Image fusion,Swin transformer,Deep learning
AI 理解论文
溯源树
样例
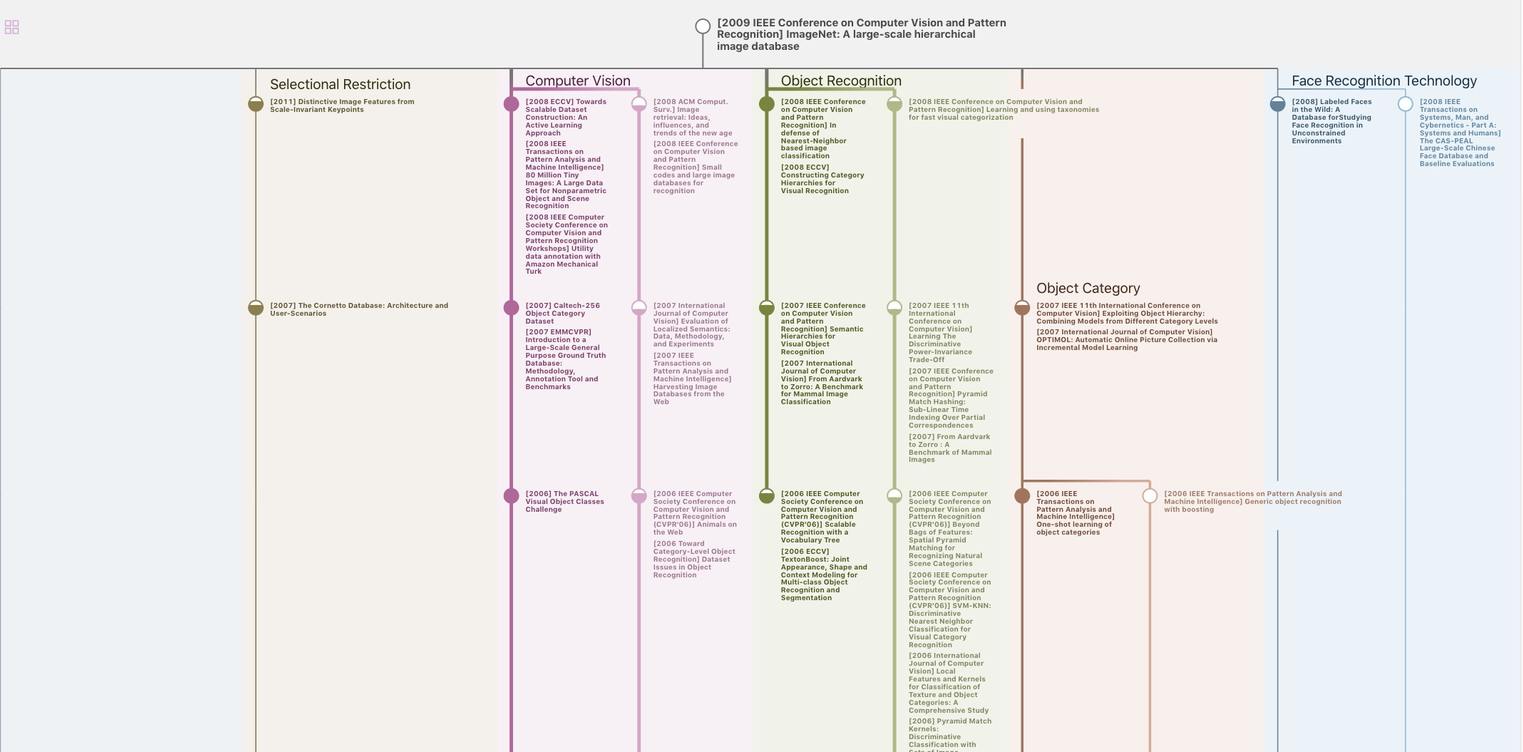
生成溯源树,研究论文发展脉络
Chat Paper
正在生成论文摘要