Active Learning for Detecting Hardware Sensors-Based Side-Channel Attack on Smartphone
Arabian Journal for Science and Engineering(2024)
摘要
The evolution of smartphones made people more dependent on them since they save their data on smartphones for convenience. This convenience aroused threats to the stored data through cyber attacks. Smartphones consist of various hardware sensors, including a magnetometer, an accelerometer, and a gyroscope, which can be vulnerable to cyberattacks such as side-channel attacks. These attacks can deduce the typed keystrokes of a soft keyboard through the vibration they produce; it can cause data leakage and threaten user privacy. This paper proposes a novel approach to detecting side-channel attacks that combine active learning-based machine learning models: Random Forest, eXtreme Gradient Boosting, Decision Tree, Gradient Boosting, K-Nearest Neighbors, and Light Gradient-Boosting Machine classifier. The approach is evaluated on a dataset generated through the smartphone’s hardware sensors (i.e., accelerometer, gyroscope, and magnetometer). The performance is evaluated in three rounds; evaluation measures demonstrate that the keystrokes can be inferred with an accuracy of 91.99
更多查看译文
关键词
Active learning,Machine learning,Side-channel attack,Data leakage,User privacy,Hardware sensors
AI 理解论文
溯源树
样例
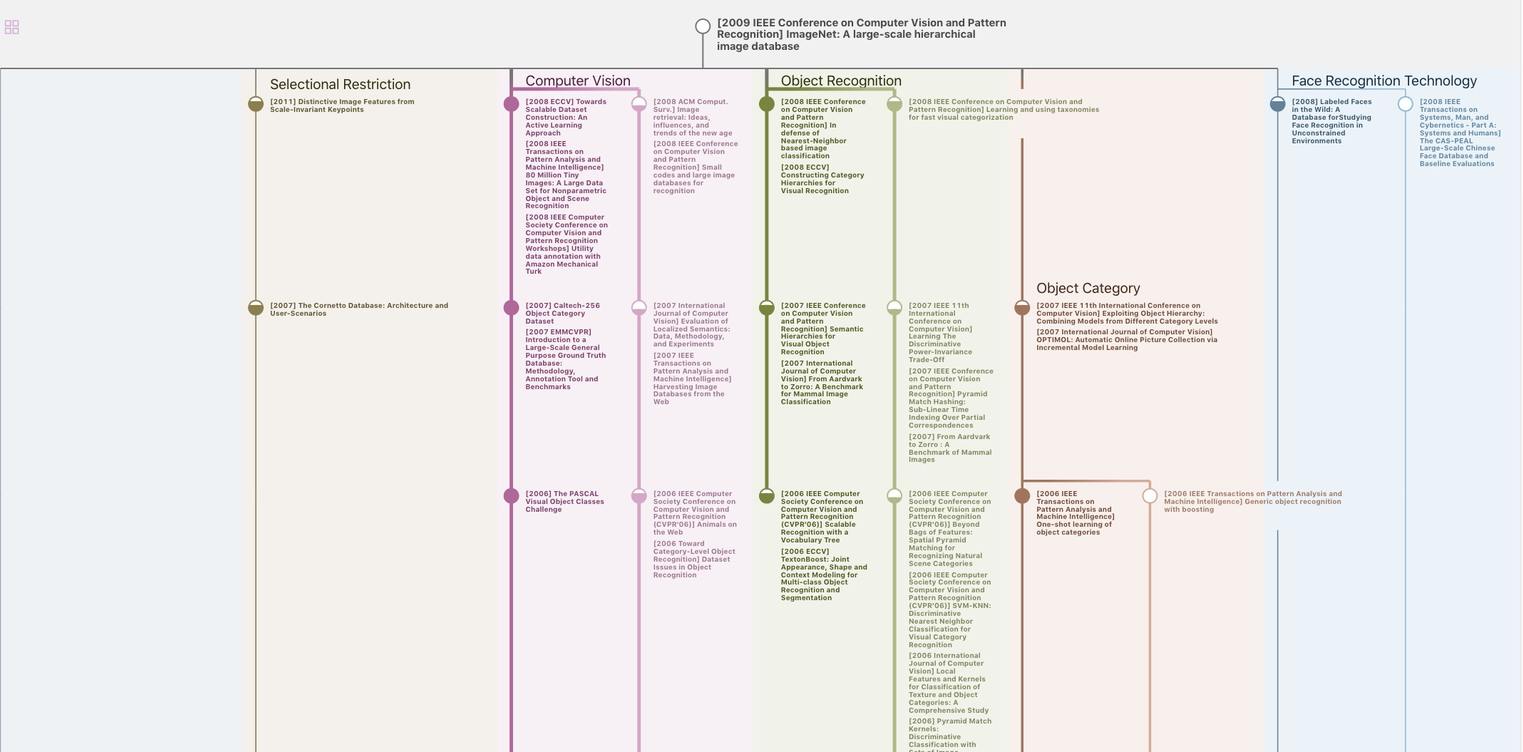
生成溯源树,研究论文发展脉络
Chat Paper
正在生成论文摘要