Spatial-temporal Attention-Based Time Series Prediction Network for Lithium Battery Remaining Useful Life Estimation
2024 4th International Conference on Neural Networks, Information and Communication (NNICE)(2024)
摘要
The accurate estimation of the remaining useful life (RUL) of lithium batteries is a pivotal aspect in battery management systems, essential for efficient battery management, optimization of battery performance, and enhanced user experience. Presently, prevailing deep learning methods for battery RUL estimation mainly focus on individual neural networks, overlooking the crucial spatial-temporal characteristics. In this study, we introduce a time series prediction framework employing a gate recurrent unit (GRU) in tandem with a spatial-temporal attention mechanism. Our methodology involves initially screening data associated with high correlation to battery capacity through correlation coefficient analysis. This screened data is then processed by a series of GRU network layers to model the relationship between battery input and capacity. Following this, the extracted features undergo a deeper level analysis, whereby a spatial-temporal attention mechanism assigns varying weights to these features in the temporal and spatial dimensions to capture correlations more intricately. We evaluated the proposed method on the NASA dataset, and the experimental findings validate that our network achieves accurate time series predictions with an MAE of less than 1.5%.
更多查看译文
关键词
Remaining Useful Life,Gate Recurrent Unit,Spatio-temporal Attention,Lithium Battery
AI 理解论文
溯源树
样例
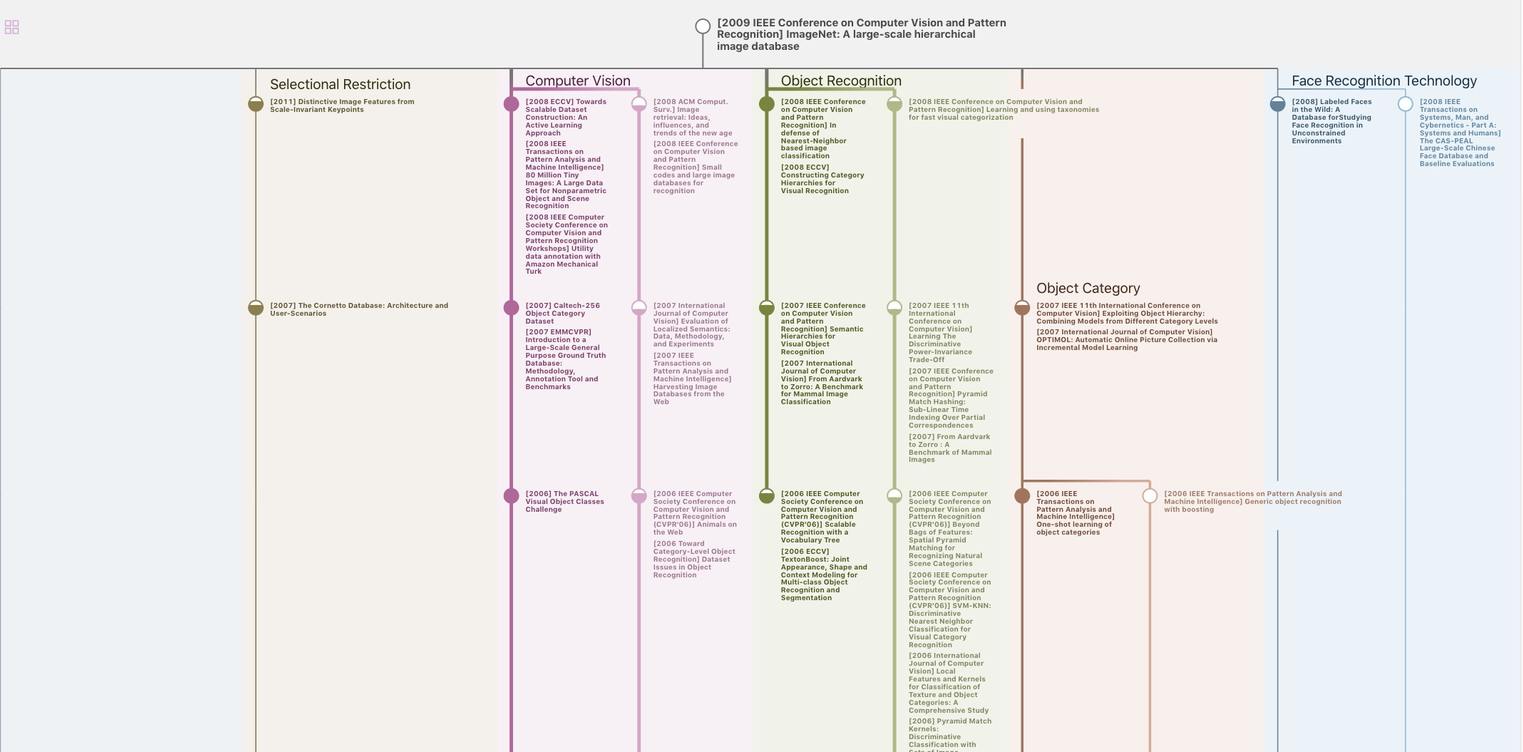
生成溯源树,研究论文发展脉络
Chat Paper
正在生成论文摘要