Enhancing Recommender Systems Performance Using Knowledge Graph Embedding with Graph Neural Networks
2024 4th International Conference on Neural Networks, Information and Communication (NNICE)(2024)
摘要
Recommender systems are a crucial component in personalized information retrieval, with their performance directly impacting user experience. However, traditional recommender systems often suffer from limitations in handling complex user-item interactions. Recently, knowledge graphs have emerged as valuable resources for enhancing recommendation effectiveness by capturing and utilizing a wide range of information. In this work, we propose a novel Auto-encoder-based structure integrated with Graph Neural Networks (GNN) layers for Knowledge Graph Embedding (KGE) tasks. This model effectively captures and leverages the rich information in knowledge graphs. Further, we combine this advanced GNN-based knowledge graph embedding model and a preference propagation mechanism inspired by RippleNet, to introduce a novel model, GraphRipple, aiming to enhance the recommendation performance. Extensive experiments on multiple datasets demonstrate the effectiveness of GraphRipple in improving recommendation quality.
更多查看译文
关键词
recommender systems,knowledge graph,graph neural networks,knowledge graph embedding
AI 理解论文
溯源树
样例
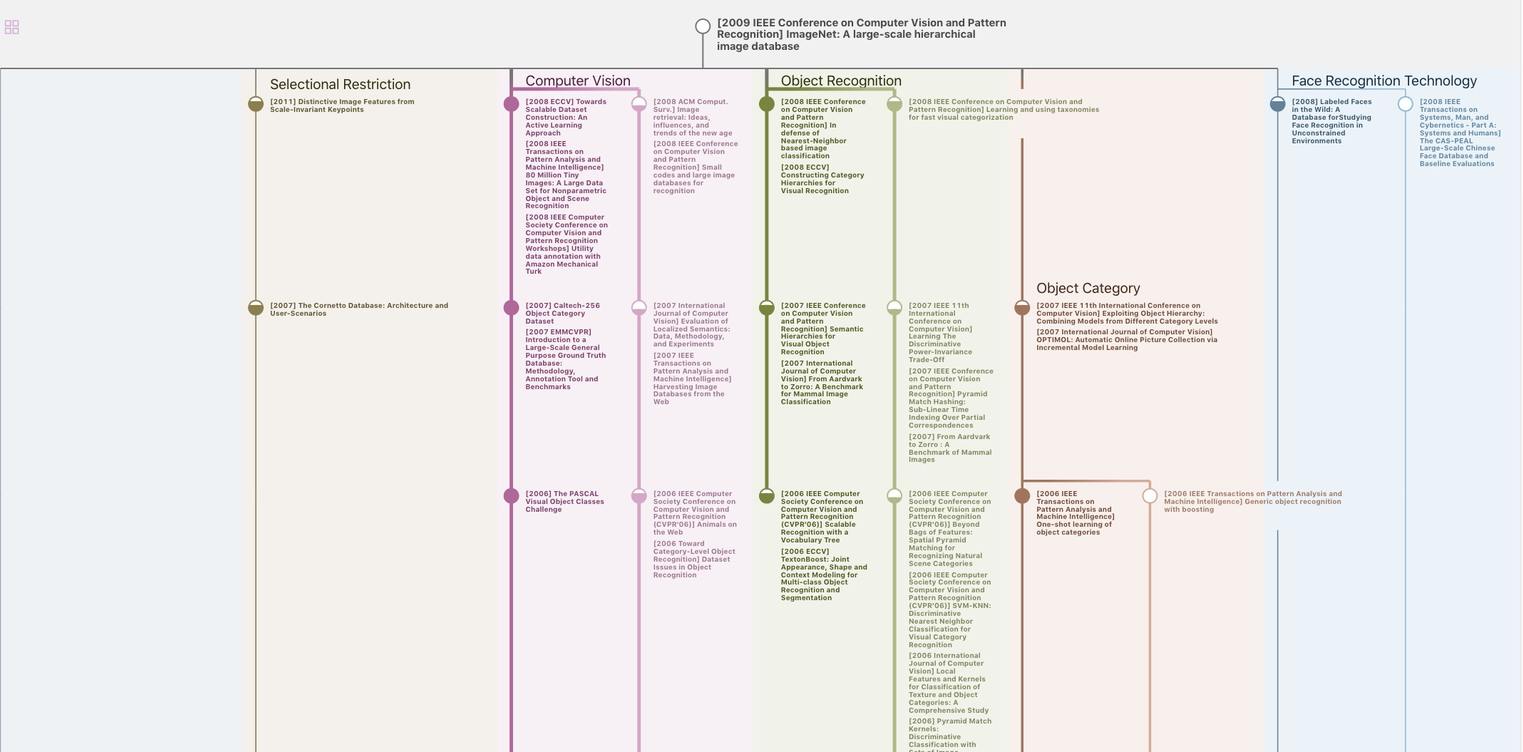
生成溯源树,研究论文发展脉络
Chat Paper
正在生成论文摘要