Improved Lightweight GFocal Method for Tile Detection
2024 4th International Conference on Neural Networks, Information and Communication (NNICE)(2024)
摘要
In the field of industrial production, tile defect detection is one of the key aspects of quality control. Aiming at the problems of high computational complexity and poor detection ability of small targets in industrial tile defect detection, a lightweight tile defect detection method based on GFocal is proposed. The method uses FasterNet as the backbone, which improves the coarse feature extraction ability of the model and increases the detection speed. Secondly, DFC-Involution is introduced in the multi-feature fusion part, which reduces the model parameters and improves the feature fusion ability of the model. Quality Focal Loss and Distribution Focal Loss are utilized to solve the inconsistency and inflexibility of classification and quality prediction. Finally, the BCKD module of knowledge distillation is utilized to replace the backbone with a smaller mobilenetv2, which significantly reduces the model parameters. Experimental results show that the model achieves an average accuracy (mAP) of 77.51 in tile defect detection and the parameters are reduced by 73% compared with GFocal. Compared with the industrial mainstream algorithms, the improved algorithm realizes a balance between parameters and accuracy.
更多查看译文
关键词
deep learning,defect detection,GFocal,feature fusion,FasterNet
AI 理解论文
溯源树
样例
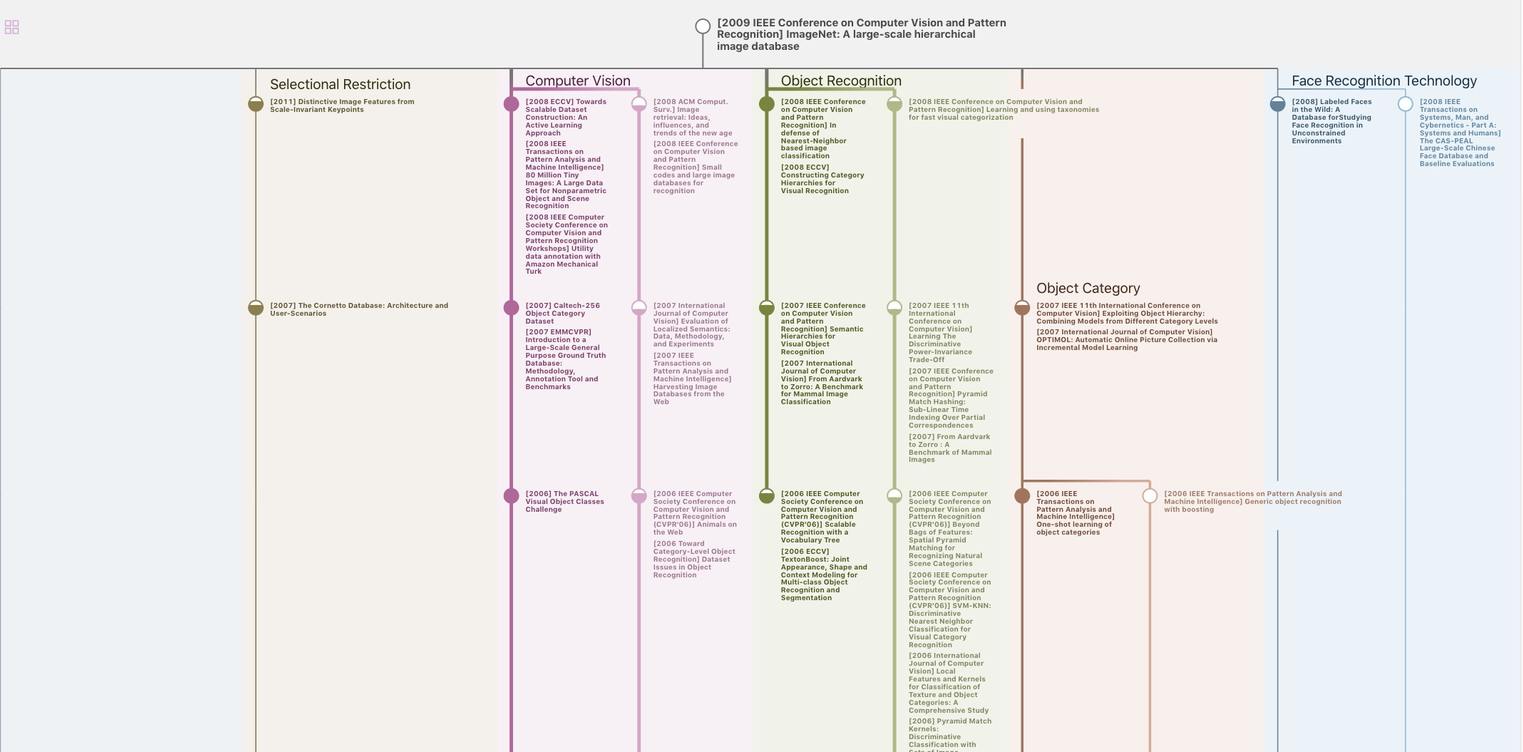
生成溯源树,研究论文发展脉络
Chat Paper
正在生成论文摘要