On the importance of integrating convolution features for Indian medicinal plant species classification using hierarchical machine learning approach
Ecological Informatics(2024)
摘要
This work proposes a novel hierarchical classification framework designed to categorize hundred Indian medicinal plant species. The innovation lies in introducing a comprehensive feature representation by integrating convolutional features with geometric, texture, shape, and multispectral features for classification tasks. In this study, a two-level hierarchical plant classification model is proposed to address the challenges of inter-class similarity and intra-class variations. The first level classifies 100 medicinal plant species into 11 groups based on visual similarities among the plants. At level two, the specific plant species containing in each group are predicted using Random Forest classifier. The evaluation is performed at two levels to analyze the effectiveness of the proposed model. The performance analysis compares the effectiveness of individual feature types against the composite feature model. Performance is also evaluated based on specific groups that demonstrate high similarity between classes and intra-class variations among the plant species separately. Furthermore, the generality of the model is tested using two self-created datasets-RTL80 and RTP40, requiring more than 300 man-hours to collect. Experimental results demonstrate a promising accuracy of 94.54% on GSL100 leaf dataset and 75.46% on RTL80 and RTP40 real-time datasets reflecting the superiority of the proposed hierarchical model over state-of-the-art methods.
更多查看译文
关键词
Medicinal plant classification,Fusion features,Convolution features,Smartphone images,Inter-class similarities
AI 理解论文
溯源树
样例
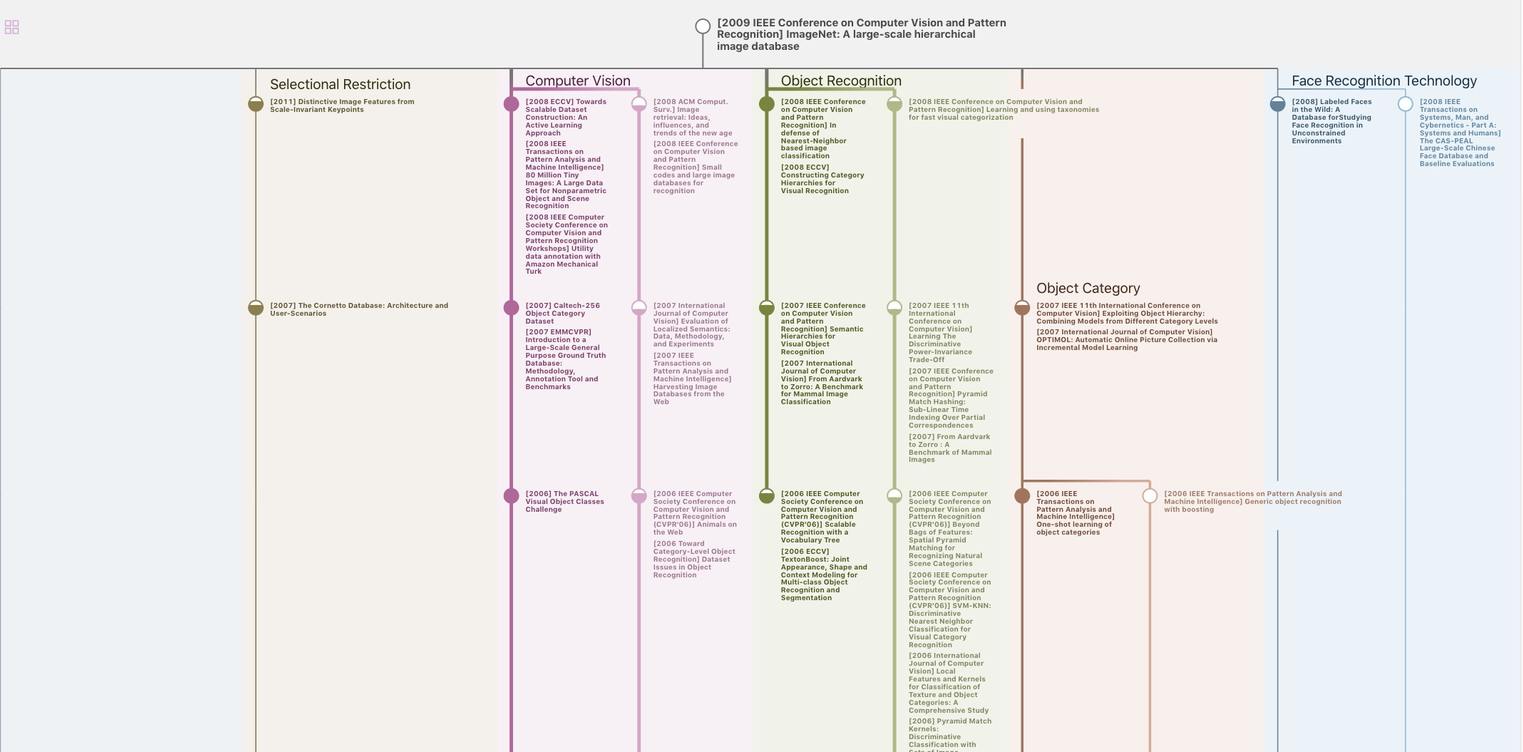
生成溯源树,研究论文发展脉络
Chat Paper
正在生成论文摘要