Lost in Space: Probing Fine-grained Spatial Understanding in Vision and Language Resamplers
NAACL (Short Papers)(2024)
AI Read Science
Must-Reading Tree
Example
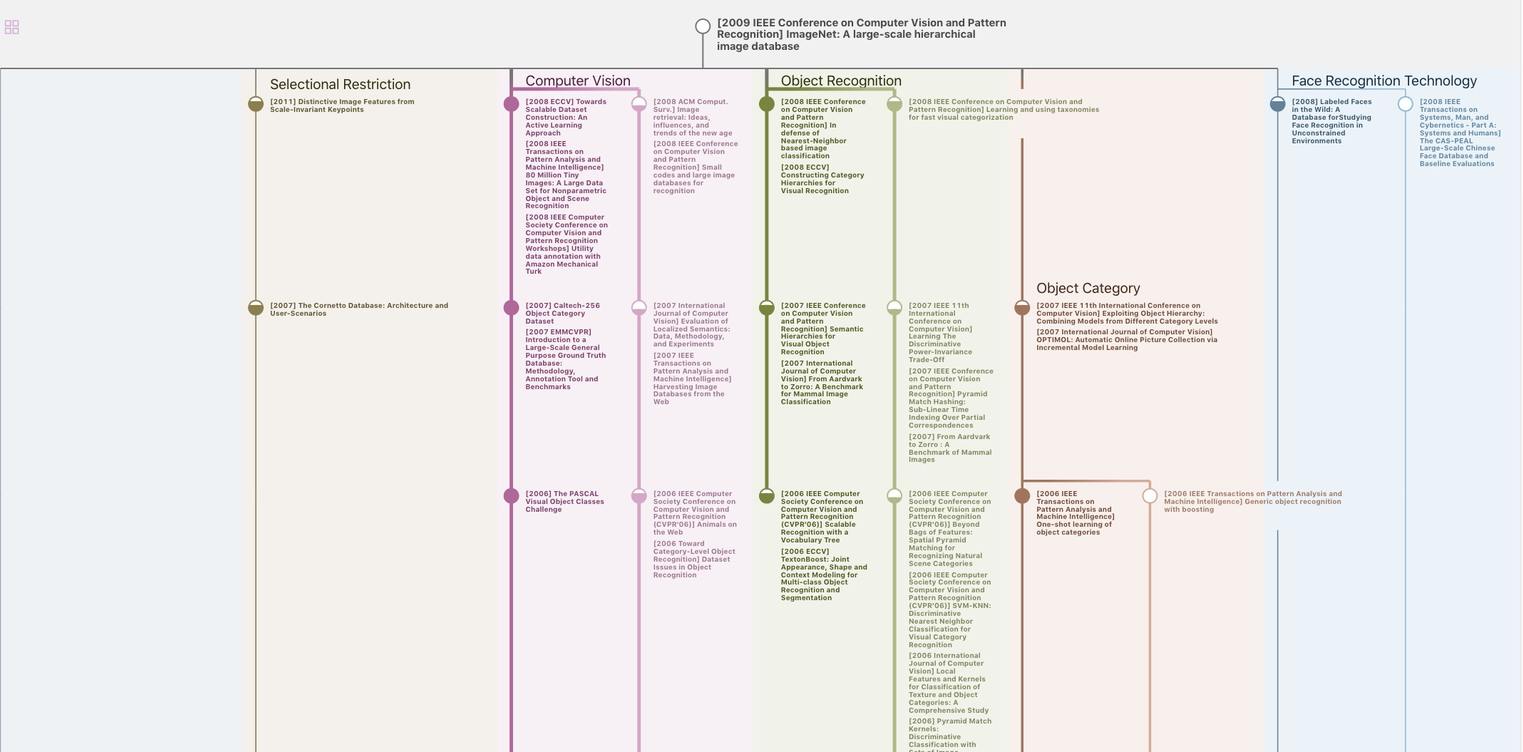
Generate MRT to find the research sequence of this paper
Chat Paper
Summary is being generated by the instructions you defined