Reinforced Fuzzy Domain Adaptation: Revolutionizing Data-Unaccessible Rotating Machinery Fault Diagnosis Across Multiple Domains
Expert systems with applications(2024)
摘要
Existing domain adaptation methods typically require access to raw data from both the source and target domains, increasing the risk of data leakage. In light of this, a fuzzy domain adaptation-based rotation machinery fault diagnosis method that does not require access to data is proposed in this paper. Specifically, during the source domain training phase, all source models are simultaneously trained by contributing model parameters. The developed joint training strategy significantly enhances the multi-domain performance of each private source model. Simultaneously, a model incorporating a fusion fuzzy strategy is constructed to acquire fuzzy rules for the source domain samples and anchor points corresponding to each fault mode. During the target domain adaptation phase, firstly, under the setting of frozen fuzzy rules, the source feature extractor is retrained using target data. Subsequently, an anchor point alignment strategy is introduced to transfer diagnostic knowledge between domains. Finally, a filtering strategy is devised to obtain high-confidence pseudo-labels for the target domain, coupled with a self-supervised learning strategy for the final optimization of the target model. Experimental results demonstrate significant achievements of the proposed method across twelve multi-source cross-domain fault diagnosis cases, with an average diagnostic accuracy of 98.88%. Encouragingly, the proposed method maintains outstanding performance even without accessing source data, outperforming several other methods
更多查看译文
关键词
Data privacy leakage,Data-unaccessible,Fault diagnosis,Fuzzy domain adaptation,Self -supervised learning strategy
AI 理解论文
溯源树
样例
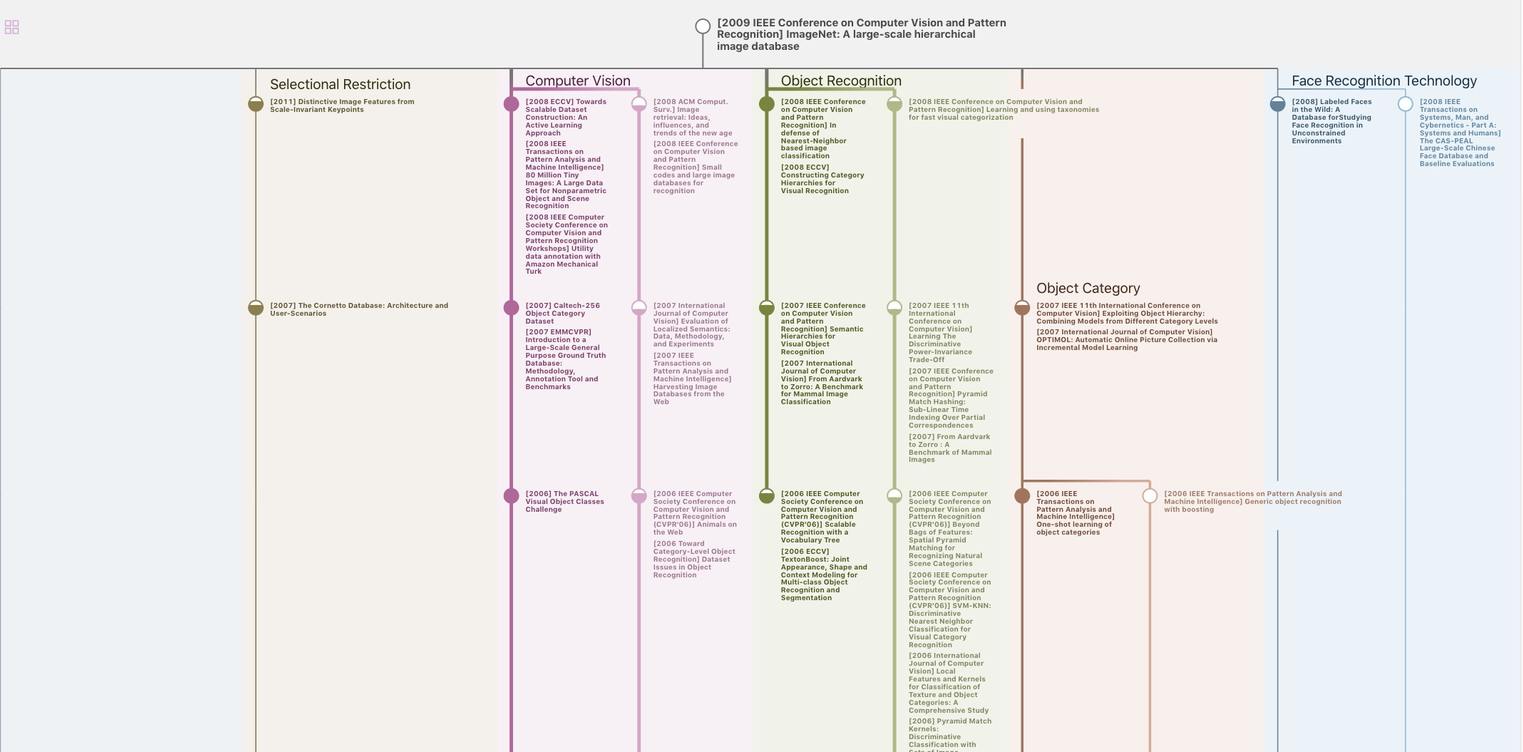
生成溯源树,研究论文发展脉络
Chat Paper
正在生成论文摘要