A Deep Learning Short Text Classification Model Integrating Part of Speech Features
2024 4th International Conference on Neural Networks, Information and Communication (NNICE)(2024)
摘要
In short text classification, extracting text features is crucial. Static word vector training, the traditional method, has limitations such as insufficient semantics and sparse features, while dynamic training word vectors lack part-of-speech features. To overcome these issues, this paper proposes a new text classification model, PB-DPCNN, which combines part-of-speech tagging technology with dynamic word vector training using BERT. Part-of-speech vectors are constructed with part-of-speech attributes through part of speech related weights, and double-layer convolution captures long-distance patterns to improve word embedding richness. The output layer is combined with residual pooling, and the connection layer obtains the final text feature representation. Experimental results on the THUCNews dataset demonstrate that the model enhances each classification index and has good text semantic recognition ability.
更多查看译文
关键词
BERT,Neural network,Part-of-speech feature,Text classification
AI 理解论文
溯源树
样例
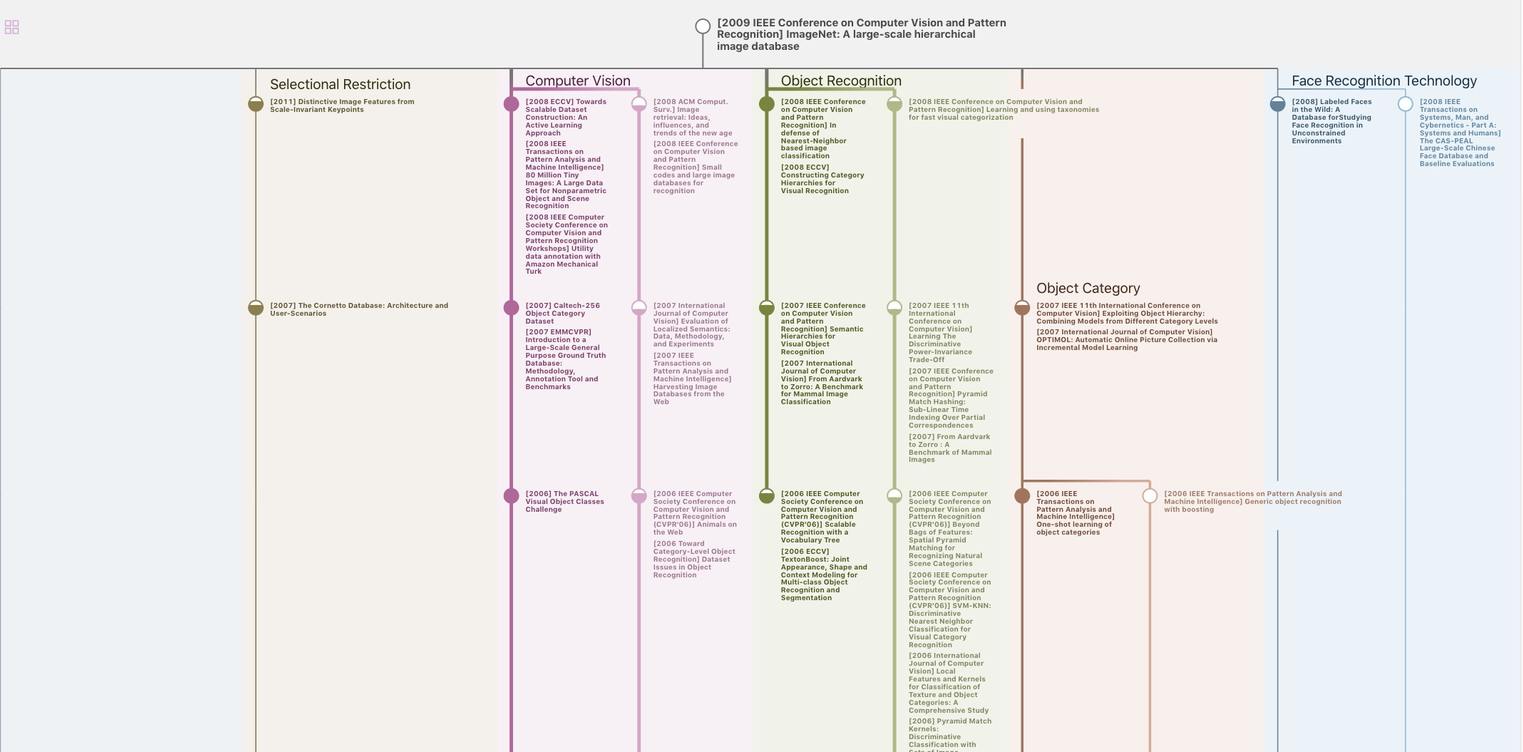
生成溯源树,研究论文发展脉络
Chat Paper
正在生成论文摘要