A Neural Network Modeling Method With Low-Rate Sampling for Wide Temperature Range SiC MOSFETs Application
IEEE TRANSACTIONS ON ELECTRON DEVICES(2024)
摘要
With the rapid development of semiconductor technology, conventional modeling based on physical equations encounters challenges related to accuracy and development time. The study proposes a behavioral-level modeling approach based on artificial neural networks (ANNs), aiming to swiftly and accurately model SiC MOSFETs when used in CMOS integrated circuits over a wide temperature range. Nevertheless, achieving precise ANN model training typically demands a substantial volume of data, incurring costs related to measurements and lengthy training periods. To address this issue, sampling-based methods for acquiring training data play a crucial role, but they come with a notable limitation. Lower sampling rates result in a considerable reduction in model accuracy, whereas higher sampling rates fail to effectively tackle the time-consuming issue and the associated costs of model training. To train the ANN model with less data without compromising accuracy, this study uses the uniform random sampling (URS) method and the Latin hypercube sampling (LHS) method based on stratified sampling during the training set acquisition process. The results demonstrate that LHS significantly outperforms URS in terms of accuracy at the same sampling rate of 2%. For further enhancement of fitting accuracy in the transition region, a segmented LHS (SLHS) method is proposed, showcasing superior modeling capability. The ANN model constructed using this sampling method enhances fitting accuracy in the transition region between linear and saturation regions by 38.6% and overall fitting accuracy by 17.3%, when compared with LHS method.
更多查看译文
关键词
Artificial neural network (ANN),behavioral models,sampling method,SiC MOSFET
AI 理解论文
溯源树
样例
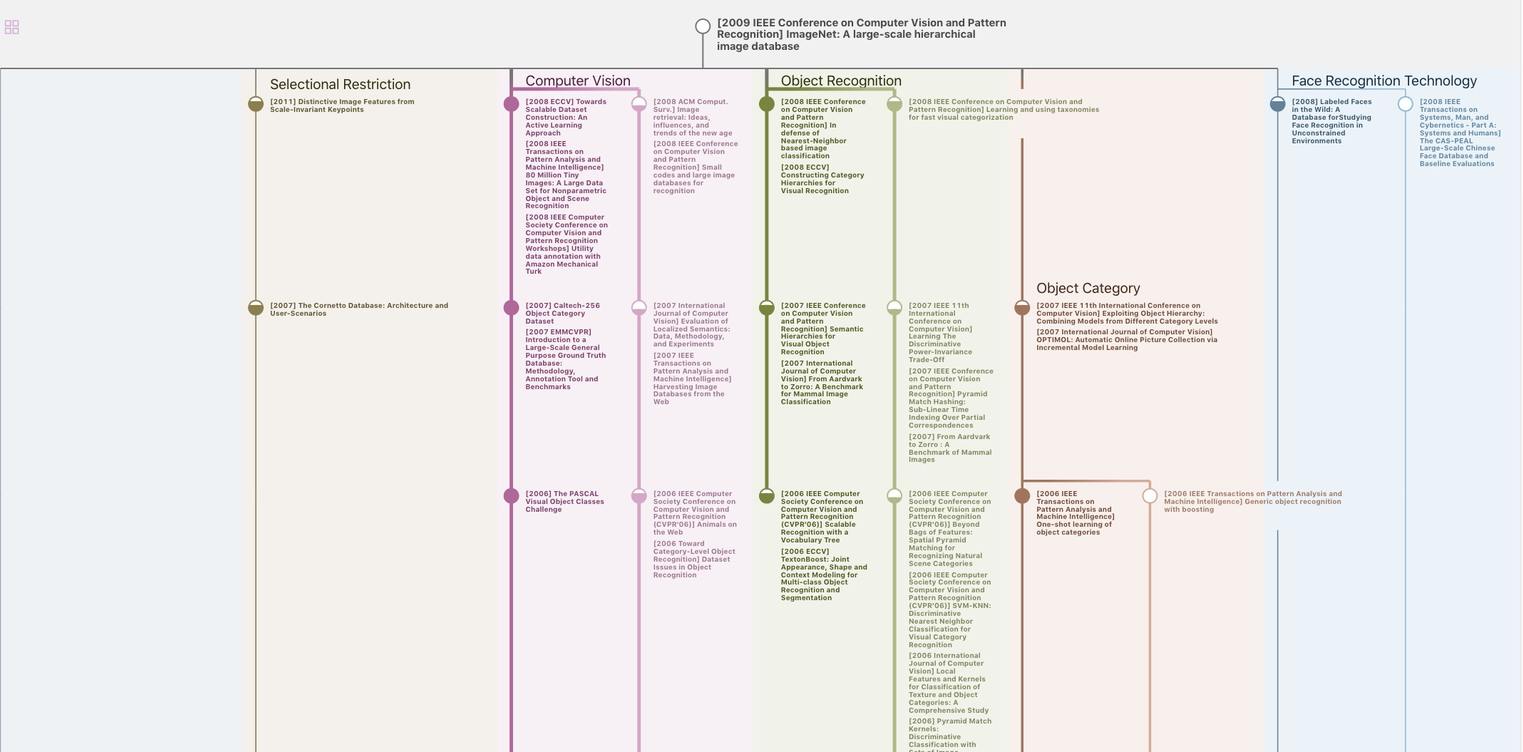
生成溯源树,研究论文发展脉络
Chat Paper
正在生成论文摘要