Metaheuristic Optimization Based- Ensemble Learners for the Carbonation Assessment of Recycled Aggregate Concrete
Applied soft computing(2024)
摘要
This study addresses the enhanced prevalence of carbonation, a process accelerating steel reinforcement corrosion, in recycled aggregate concrete (RAC) compared to natural aggregate concrete. Traditional carbonation depth assessment methods in RAC are noted for being labor-intensive, costly, and requiring specialized expertise. There’s a noted deficiency in the application of machine learning techniques for accurately predicting carbonation depth in RAC, a gap this study aims to fill. Utilizing the extreme gradient boosting (XGBoost) technique, recognized for its efficacy in ensemble machine learning, this study innovates in modeling carbonation depth in RAC. It emphasizes the criticality of hyperparameter optimization of the XGBoost algorithm for maximizing model accuracy. To achieve this, three novel metaheuristic optimization algorithms, including reptile search algorithm (RSA), Aquila optimizer (AO), and arithmetic optimization algorithm (AOA), were introduced as global optimizers for tunning the XGBoost hyperparameters. The study was underpinned by a comprehensive database compiled from extensive literature, facilitating the development of an accurate RAC carbonation depth model. Through rigorous evaluations, including sensitivity analyses, the Wilcoxon signed-rank test, and runtime comparisons, the synthesized models demonstrated exceptional accuracy, with coefficients of determination exceeding 0.95. The XGBoost-AO algorithm, in particular, showcased superior performance, with the XGBoost-RSA algorithm providing efficient predictions considering runtime. SHapley Additive exPlanations (SHAP) interpretation highlighted environmental conditions as significant carbonation depth influencers. A user-friendly graphical user interface was developed, enhancing the practical utility of the findings for predicting carbonation depth progression in RAC over time. This research significantly advances the predictive accuracy for carbonation depth in RAC, contributing to the sustainable management of concrete infrastructures and emphasizing the integration of advanced machine learning techniques with metaheuristic optimization for environmental and structural engineering advancements.
更多查看译文
关键词
Recycled aggregate concrete,Carbonation depth,XGboost ensemble technique,Equilibrium optimizer,Arithmetic optimization algorithm,Reptile search algorithm
AI 理解论文
溯源树
样例
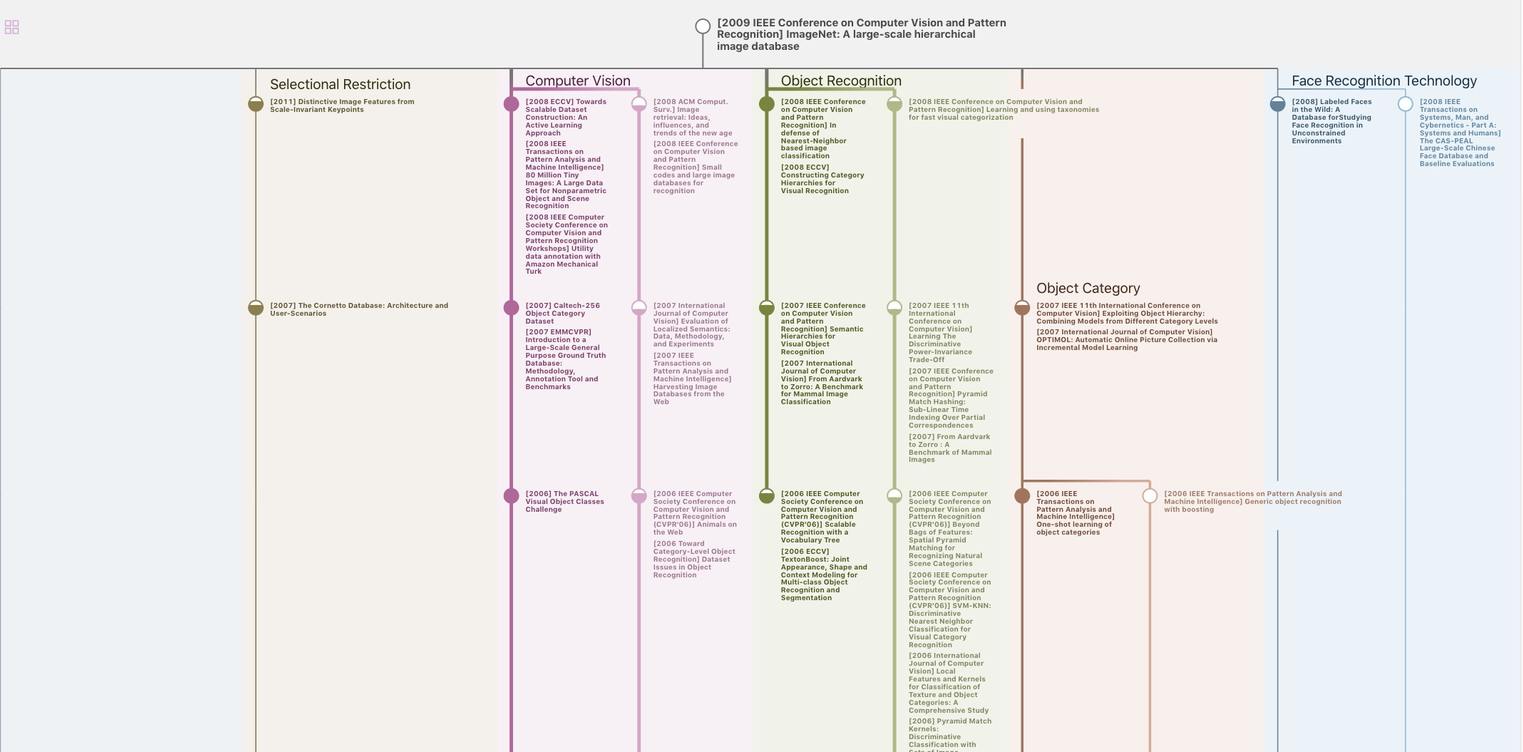
生成溯源树,研究论文发展脉络
Chat Paper
正在生成论文摘要