Tracking-Removed GRU with Denoising Autoencoder for Multivariate Time Series Imputation
2024 4th International Conference on Neural Networks, Information and Communication (NNICE)(2024)
摘要
We propose the Tracking-Removed Gated Recurrent Unit (TRGRU) with Denoising Autoencoder (DAE) for handling missing values in the incomplete multivariate time series. The internal network of TRGRU is replicated into several subnetworks, each tasked with reconstructing a corresponding attribute of the multivariate time series utilizing information from other attributes, which removes the attribute’s self-tracking during the reconstruction. The output of TRGRU is the reconstruction of its input, enabling the utilization of observed values at the current time step in estimating missing values. These network groups share weights, leading to a reduction in overall network parameters. Additionally, we design an imputation unit based on DAE, which leverages historical information and the hidden features of input data to impute missing values within the input data and generate completed data for the training of TRGRU, enabling the model to be trained using incomplete datasets. Experimental results across multiple datasets have corroborated the effectiveness of TRGRU-DAE.
更多查看译文
关键词
Multivariate time series,Missing value imputation,GRU,DAE
AI 理解论文
溯源树
样例
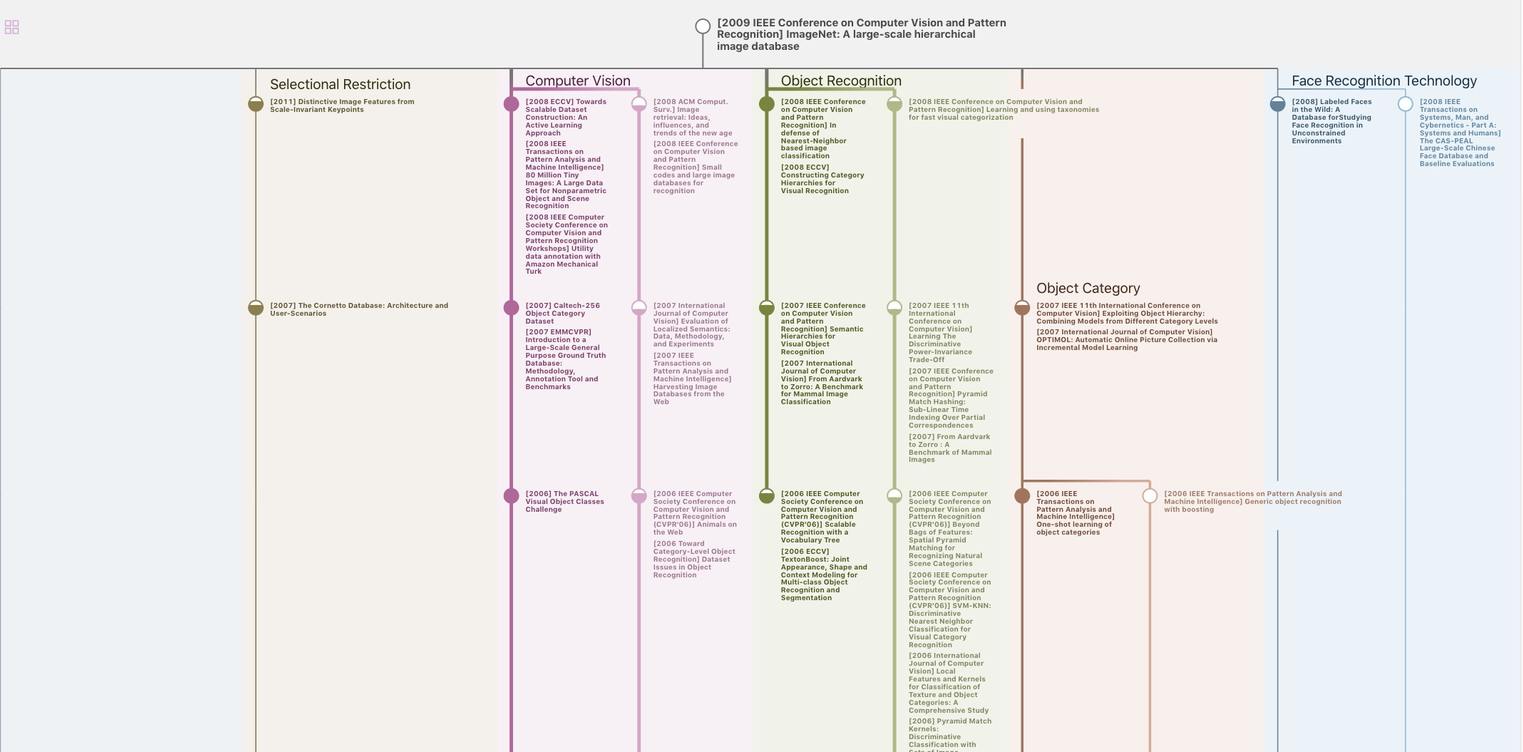
生成溯源树,研究论文发展脉络
Chat Paper
正在生成论文摘要