Elucidation of Intelligent Classification Framework for Hydroponic Lettuce Deficiency Using Enhanced Optimization Strategy and Ensemble Multi-Dilated Adaptive Networks
IEEE ACCESS(2024)
摘要
Recent technological breakthroughs enable academics to suggest automating hydroponic farms to increase productivity and reduce workforce requests, hence improving agricultural profitability and output. Traditional deep learning models for detecting plant diseases may pose challenges in terms of their compatibility with embedded systems and only a few research works show their implementation on low-resource Internet of Things (IoT) devices like smartphones or Raspberry Pi. The current models have several shortcomings, including a substantial power bill, high computing power demands, quick energy dissipation, and significant storage space demands because of their intricate structures. Hence, in this paper, an intelligent hydroponic lettuce deficiency classification method is presented by employing deep learning. Initially, collected images are taken to the developed Ensemble Multi-Dilated Adaptive Networks (E-MD-ANet)-based deficiency classification network. In this network, techniques such as Visual Geometry Group 16 (VGG16), Residual Attention Network (RAN), and Convolutional Neural Network (CNN) are adopted for the extraction of the feature stage. Further, the extracted features from these networks are given to the Long Short-Term Memory (LSTM) for the deficiency classification. The parameters presented in the E-MD-ANet network are tuned using the proposed Improvised Honey Badger Algorithm (I-HBA). Thus, the hydroponic lettuce deficiency classified image is attained in the end. Here, the experiments are conducted based on the Lettuce NPK dataset which contains the, Fully Nutritional, Potassium Deficient, and Nitrogen Deficient, and Phosphorus Deficient whereas the total count of images is gathered as 12, 58, 66, and 72. Also, various evaluation parameters are taken for the analysis like precision, NPV, specificity, F1-score, FPR, FDR, accuracy, MCC, FNR, and sensitivity. In the end, the efficacy validation of the suggested task is conducted and contrasted with numerous conventional classification mechanisms to showcase the system's efficacy. The offered approach shows elevated performance regarding accuracy and specificity rate which attains 96% and 95%. From the result analysis, the offered approach achieves greater performance while combining with the baseline techniques.
更多查看译文
关键词
Hydroponic,lettuce deficiency classification,ensemble multi-dilated adaptive networks,improvised honey badger algorithm,deep learning,smart farming
AI 理解论文
溯源树
样例
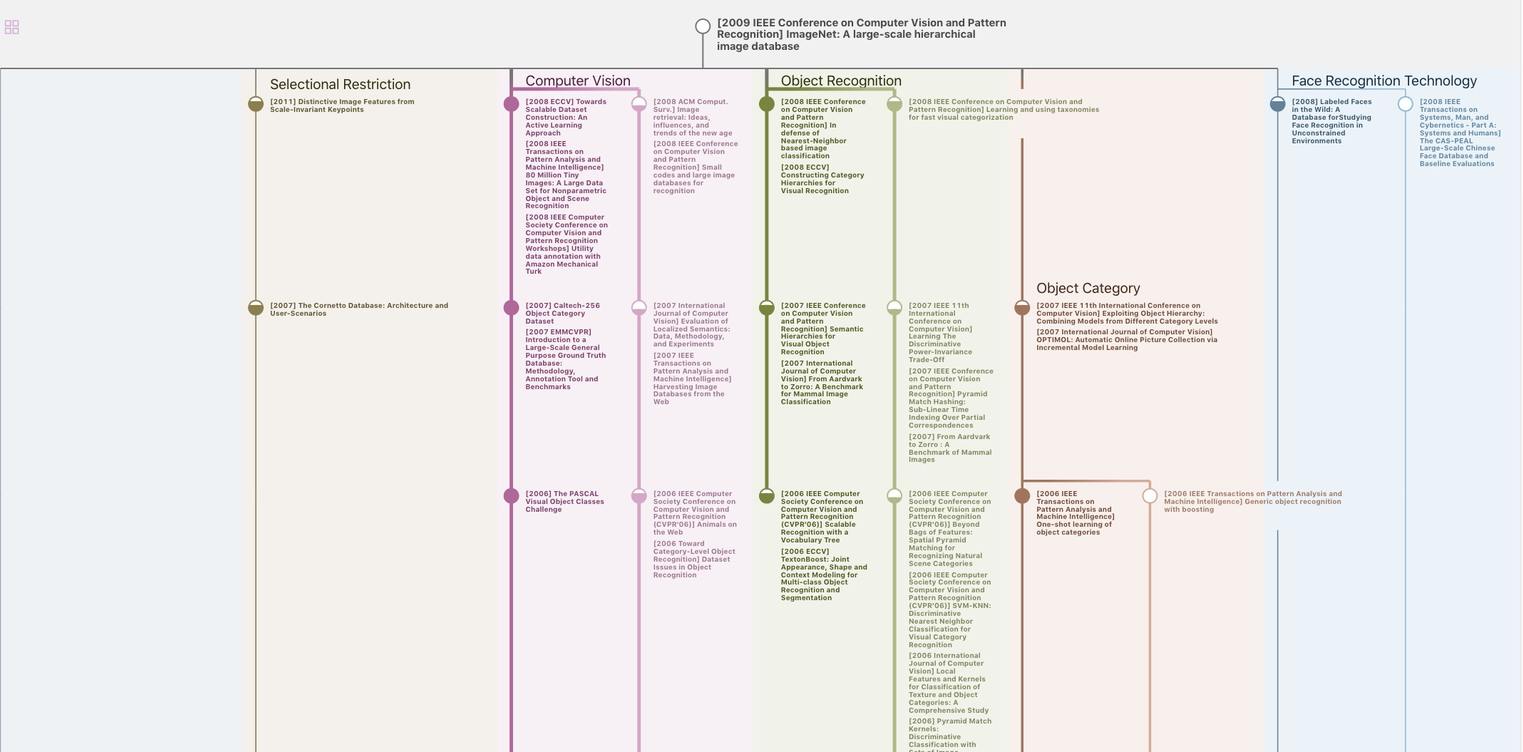
生成溯源树,研究论文发展脉络
Chat Paper
正在生成论文摘要