Assessing the accuracy and efficiency of free energy differences obtained from reweighted flow-based probabilistic generative models
crossref(2024)
摘要
Computing free energy differences between metastable states characterized by non-overlapping Boltzmann distributions is often a computationally intensive endeavour, usually requiring chains of intermediate states to connect these metastable states. Targeted free energy perturbation (TFEP) can significantly lower the computational cost of FEP calculations by choosing a set of invertible maps used to directly transform the distributions of interest, achieving the necessary statistically significant overlaps without sampling any intermediate states. Probabilistic generative models (PGMs) based on normalising-flow architectures can make it much easier via machine learning to train invertible maps needed for TFEP. However, the accuracy and applicability of approaches based on empirically learned maps depend crucially on the choice of reweighting method adopted to estimate the free energy differences. In this work, we assess the accuracy, rate of convergence, and data efficiency of different free energy estimators, including exponential averaging, BAR, and MBAR, in reweighting PGMs trained by maximum likelihood on limited amounts of molecular dynamics data sampled only from end-states of interest. We carry out the comparisons on a set of simple but representative case studies, including conformational ensembles of alanine dipeptide and ibuprofen. Our results indicate that BAR and MBAR are both data efficient and robust, even in the presence of significant model overfitting in the generation of invertible maps. This analysis can serve as a stepping stone for the deployment of efficient and quantitatively accurate ML-based FE calculation methods in complex systems.
更多查看译文
AI 理解论文
溯源树
样例
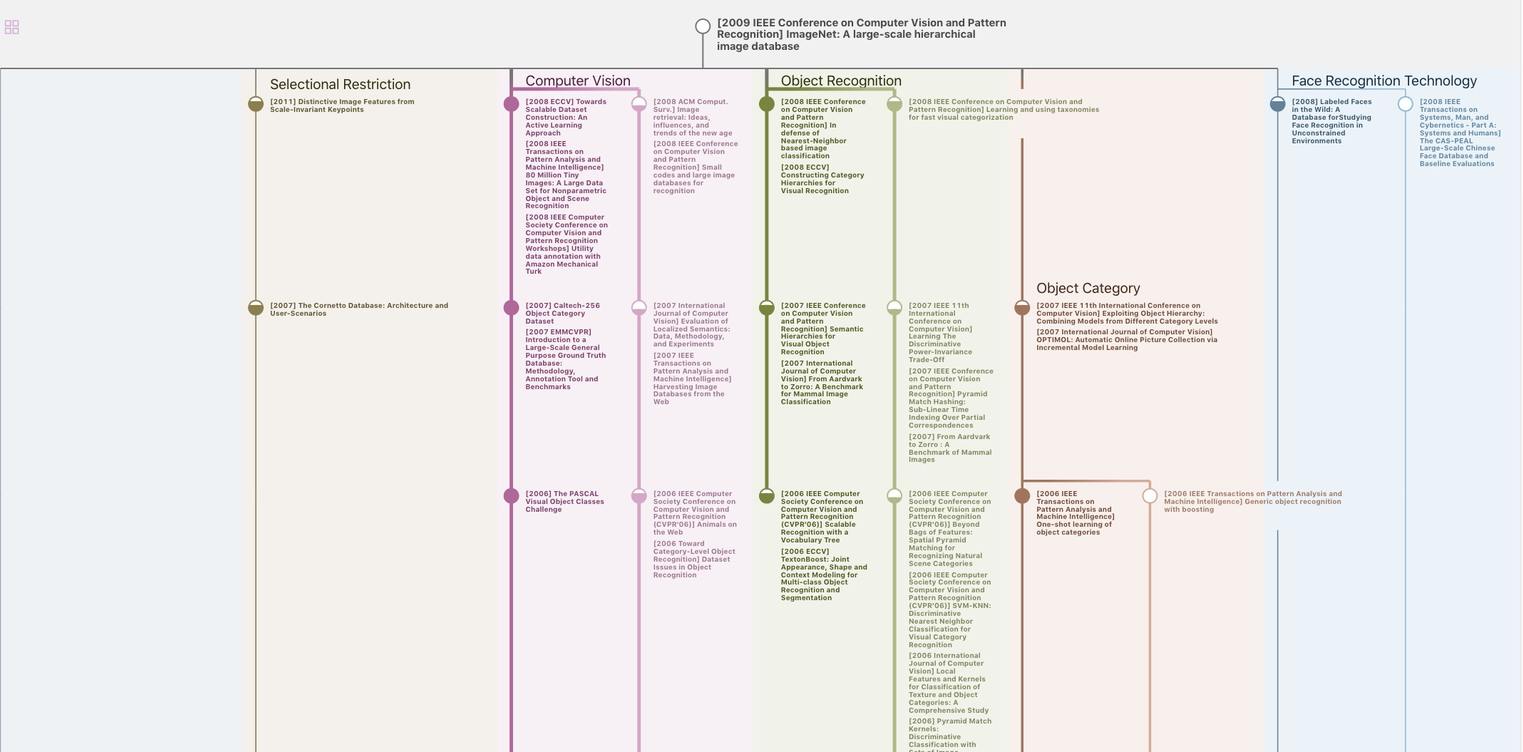
生成溯源树,研究论文发展脉络
Chat Paper
正在生成论文摘要