CARLP: A Counterfactual Augmentation and Fusion Representation Model for Link Prediction
2024 4th International Conference on Neural Networks, Information and Communication (NNICE)(2024)
摘要
Link prediction is a crucial component of knowledge graphs (KGs) as it aids in the analysis, completion, and application of KGs. Existing methods focus on learning the association between the observed graph structure and the links between a pair of nodes. However, they overlook the fact that KGs consist of interconnected nodes, and there exists a causal relationship between these nodes. Therefore, it is important to consider the causal relationship between nodes while performing link prediction in KGs. In this paper, we propose a Counterfactual Augmentation and Fusion Representation Model for Link Prediction (CARLP) that uses causality between nodes as a data augmentation of the KG. To address the poor effect of embedded representation due to the lack of link data in KG, we introduce Fusion Embedding to extract more dimensional information from KG. Additionally, we propose the ODE-Encoder to adjust the depth of the neural network by choosing a discretization scheme, making the neural network more accurate. Our experiments on KG Link Prediction demonstrate the effectiveness and genericity of the CRALP. It achieves 97.19% ± 0.18%, 97.99%± 0.17%, and 99.46%± 0.03% AUC values, and achieves 76.66%± 2.36%, 87.10%± 1.88%, and 60.21% ± 2.31% Hit@20 values on Cora, Citeseer and Facebook KGs. In particular, comparing with GCN, CRALP improves 5.08%, 3.92% and 13.00% on AUC, Hit@20 and AP, respectively.
更多查看译文
关键词
Knowledge Graph,Link Prediction,Counterfactual,Ordinary Differential Equation
AI 理解论文
溯源树
样例
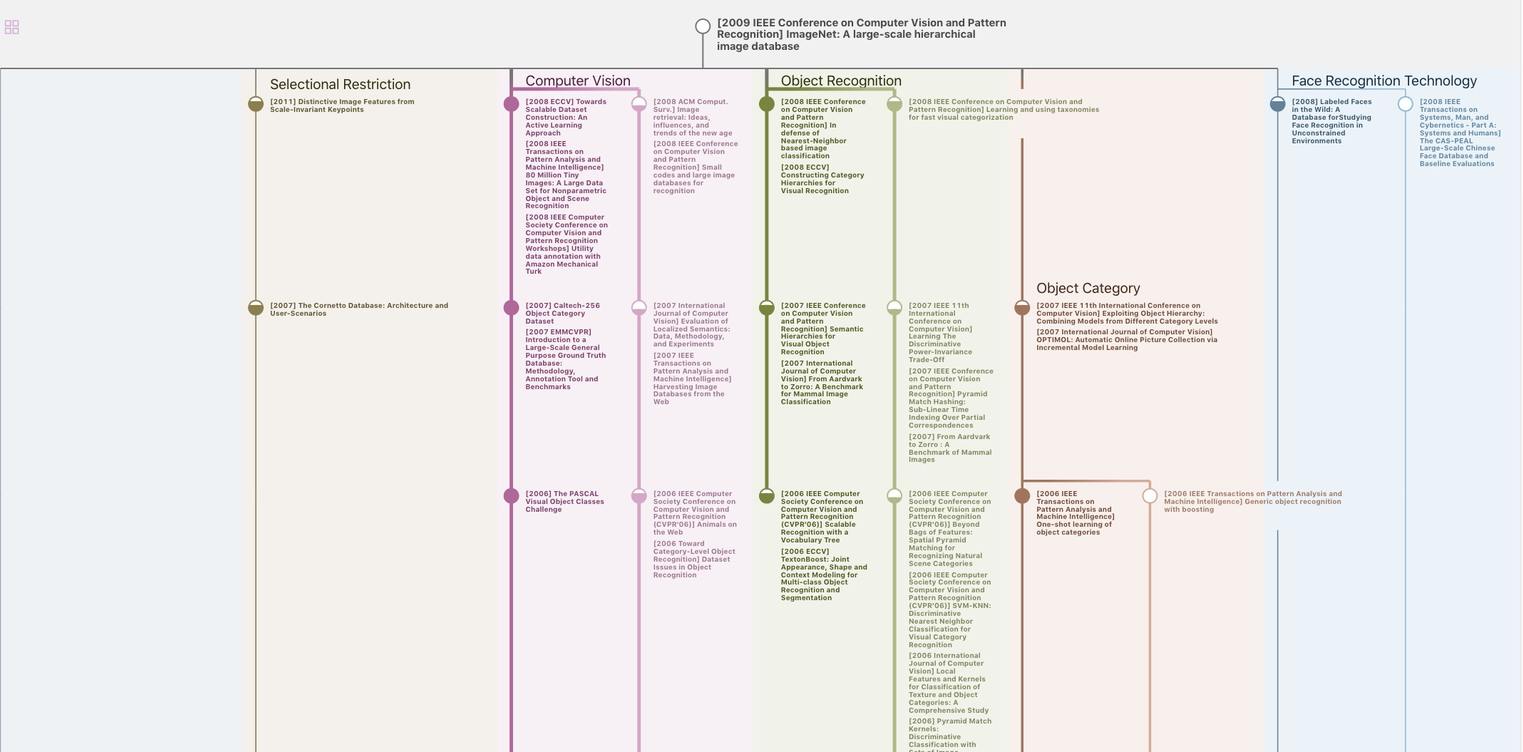
生成溯源树,研究论文发展脉络
Chat Paper
正在生成论文摘要