Embracing Federated Learning: Enabling Weak Client Participation via Partial Model Training
IEEE Transactions on Mobile Computing(2024)
摘要
In Federated Learning (FL), clients may have weak devices that cannot train the full model or even hold it in their memory space. To implement large-scale FL applications, thus, it is crucial to develop a distributed learning method that enables the participation of such weak clients. We propose
EmbracingFL
, a general FL framework that allows all available clients to join the distributed training regardless of their system resource capacity. The framework is built upon a novel form of partial model training method in which each client trains as many consecutive output-side layers as its system resources allow. Our study demonstrates that
EmbracingFL
encourages each layer to have similar data representations across clients, improving FL efficiency. The proposed partial model training method guarantees convergence to a neighbor of stationary points for non-convex and smooth problems. We evaluate the efficacy of
EmbracingFL
under a variety of settings with a mixed number of strong, moderate (
$\sim 40\%$
memory), and weak (
$\sim 15\%$
memory) clients, datasets (CIFAR-10, FEMNIST, and IMDB), and models (ResNet20, CNN, and LSTM). Our empirical study shows that
EmbracingFL
consistently achieves high accuracy as like all clients are strong, outperforming the state-of-the-art width reduction methods (i.e. HeteroFL and FjORD).
更多查看译文
关键词
Federated Learning,Heterogeneous Systems,Partial Model Training,Data Similarity
AI 理解论文
溯源树
样例
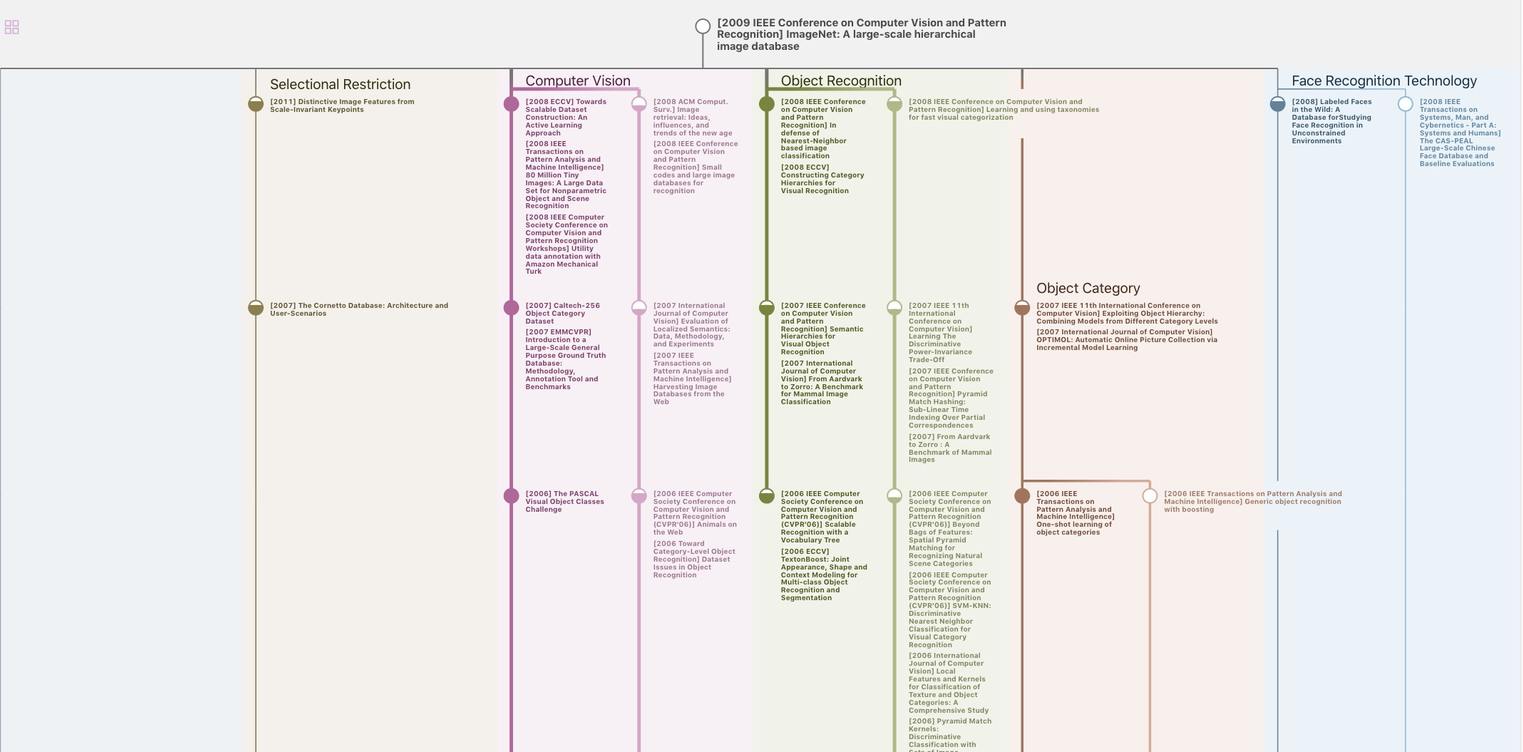
生成溯源树,研究论文发展脉络
Chat Paper
正在生成论文摘要