Residual neural network with spatiotemporal attention integrated with temporal self-attention based on long short-term memory network for air pollutant concentration prediction
Atmospheric Environment(2024)
摘要
Accurate prediction of air pollutant concentrations can be effective in controlling and preventing air pollution, which is crucial for both the government's policy response and the public's reasonable ability to avoid air pollution. In this study, we propose a model based on the principle of big data correlation and deep learning techniques for predicting the concentration of air pollutant concentrations. The proposed model, named STARes-SaLSTM, consists of a residual neural network with spatiotemporal attention (STAResNet) and a temporal self-attention based on long short-term memory network (SaLSTM). The spatiotemporal attention module in STAResNet enhances the original input information by weighting it in both spatial and temporal dimensions, meanwhile the features of the spatial distribution of pollutant and meteorological information across many cities are deeply extracted using ResNet. A novel self-attention method known as temporal self-attention is developed to extract the temporal dependency of air pollutants. LSTM is used as a sequence encoder in this temporal attention method to calculate queries, keys, and values to acquire a more comprehensive temporal dependence than is possible with normal self-attention. The STARes-SaLSTM model successfully predicts air pollution concentrations in the target city for the future by extracting spatiotemporal correlation of feature sequence. In comparison to previous neural network models and conventional methods, the model increases the accuracy of pollutant concentration prediction. The suggested prediction model works well for the one to three hours prediction job, with a root mean square error (RMSE) ranging from 6.716 to 11.648. Furthermore, even for the 1 to 24 hours prediction assignment, we executed multi-scale prediction in the target city and obtained a reasonable performance, with an average RMSE value of 20.576.
更多查看译文
关键词
Long short-term memory,Residual network,Spatiotemporal attention,Self-attention
AI 理解论文
溯源树
样例
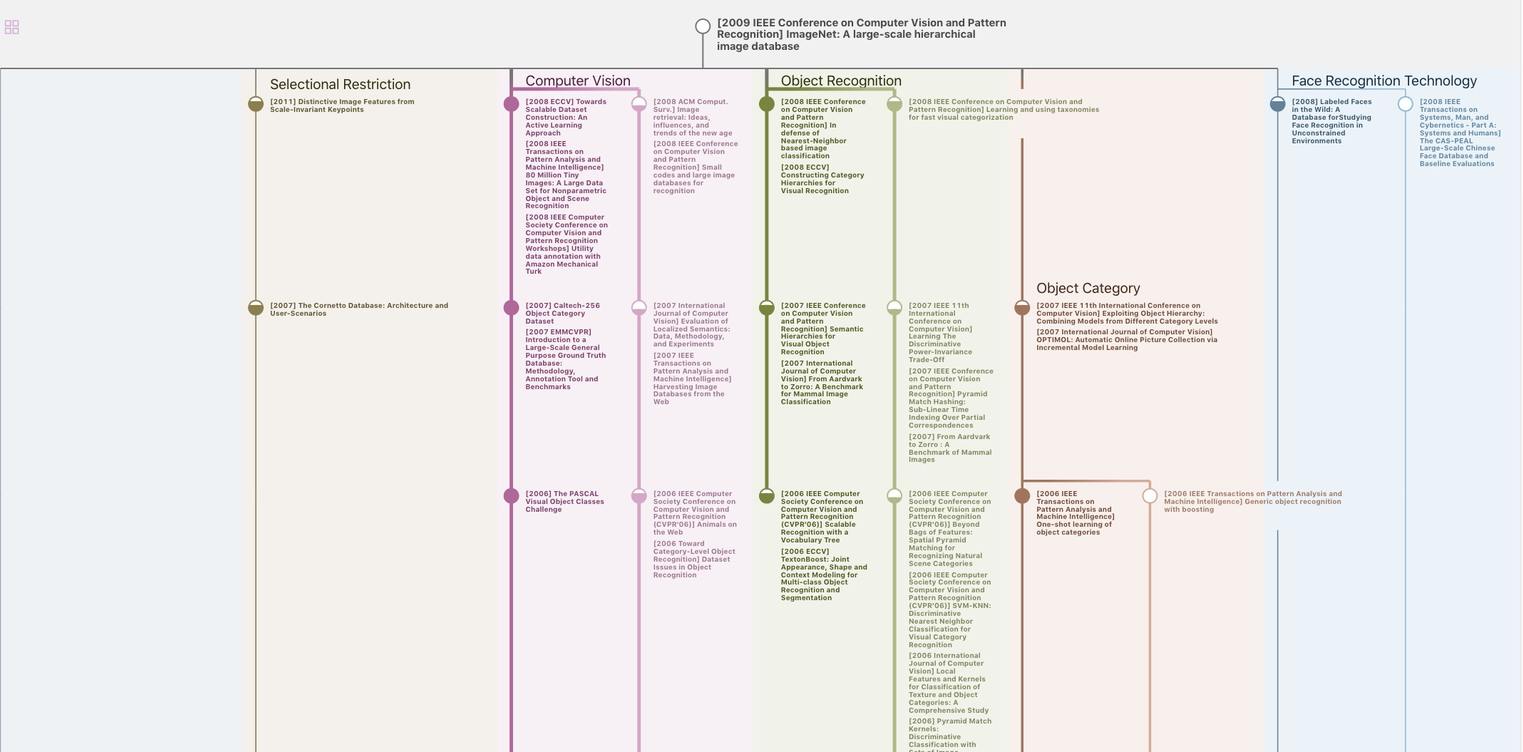
生成溯源树,研究论文发展脉络
Chat Paper
正在生成论文摘要