Affine Collaborative Normalization: A shortcut for adaptation in medical image analysis
Pattern Recognition(2024)
摘要
The paradigm of “pretraining-then-finetuning” (PT-FT) has been extensively explored to enhance the performance of clinical applications with limited annotations. A major impediment to applying such workflow to various medical imaging modalities is the lack of parameter-free approaches boosting the transferability against notable domain shifts. The success of normalization techniques in domain adaptation provides a promising solution, while inaccessible source data in finetuning (FT) poses new challenges. In this paper, we revisit the Batch Normalization module in PT-FT and propose a unified framework for both fast transferability estimation and transferability-aware finetuning. We discovered that the vanilla FT suffers from the issue that feature patterns of corresponding channels could be misaligned across domains. Hence, we present an Affine Collaborative Normalization (AC-Norm) to dynamically recalibrate the channels in the target model according to the cross-domain channel-wise correlations without any source data and extra parameters. AC-Norm provides very competitive results compared to state-of-the-art FT methods in six classification/segmentation tasks. We also demonstrate the capability of AC-Norm in estimating the transferability of pretrained models in only single-step backpropagation. Our code is available at https://github.com/EndoluminalSurgicalVision-IMR/ACNorm.
更多查看译文
关键词
Fine-tuning,Transfer learning,Transferability estimation,Self-supervised learning
AI 理解论文
溯源树
样例
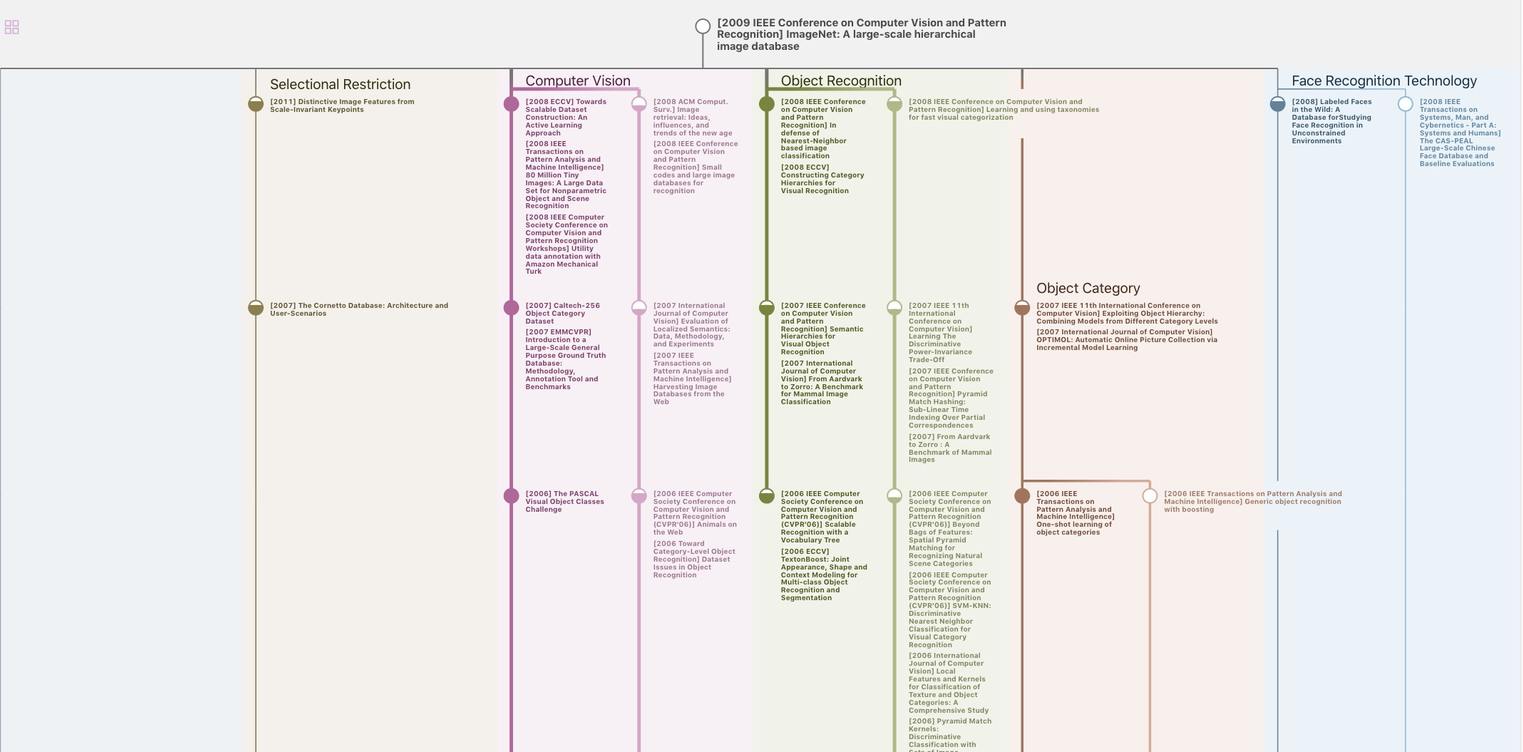
生成溯源树,研究论文发展脉络
Chat Paper
正在生成论文摘要