EMARec: a sequential recommendation with exponential moving average
Neural Computing and Applications(2024)
摘要
Capturing dynamic preference features from user historical behavioral data is widely applied to improve the accuracy of recommendations in sequential recommendation tasks. However, existing deep neural network-based sequential recommendation methods often ignore the noise information in user behavioral data that influence recommendation effectiveness, making recommendation models sensitive to noisy data. Additionally, these deep learning-based recommendation models often require a large number of parameters to capture user behavior patterns, leading to higher time complexity and susceptibility to overfitting due to noise fluctuations. To address these issues, in this paper, we apply the moving average concept from the field of time-series analysis to sequential recommendation tasks. The approach smooths sequence data, removes noise, making the data more stable and reliable, increases the model’s insensitivity to noise information, and better understands the evolution of user interests and behavioral patterns. Specifically, in our experiments, the moving average algorithm effectively denoises sequential data with low time complexity. Therefore, we propose a Sequential Recommendation with Exponential Moving Average with MLP architecture that makes the model more competitive in terms of time complexity. Experimental results conducted on two datasets demonstrate that EMARec outperforms state-of-the-art sequential recommendation methods across various common evaluation metrics.
更多查看译文
关键词
Sequential recommendation,Moving average algorithm,MLP model,Recommender systems
AI 理解论文
溯源树
样例
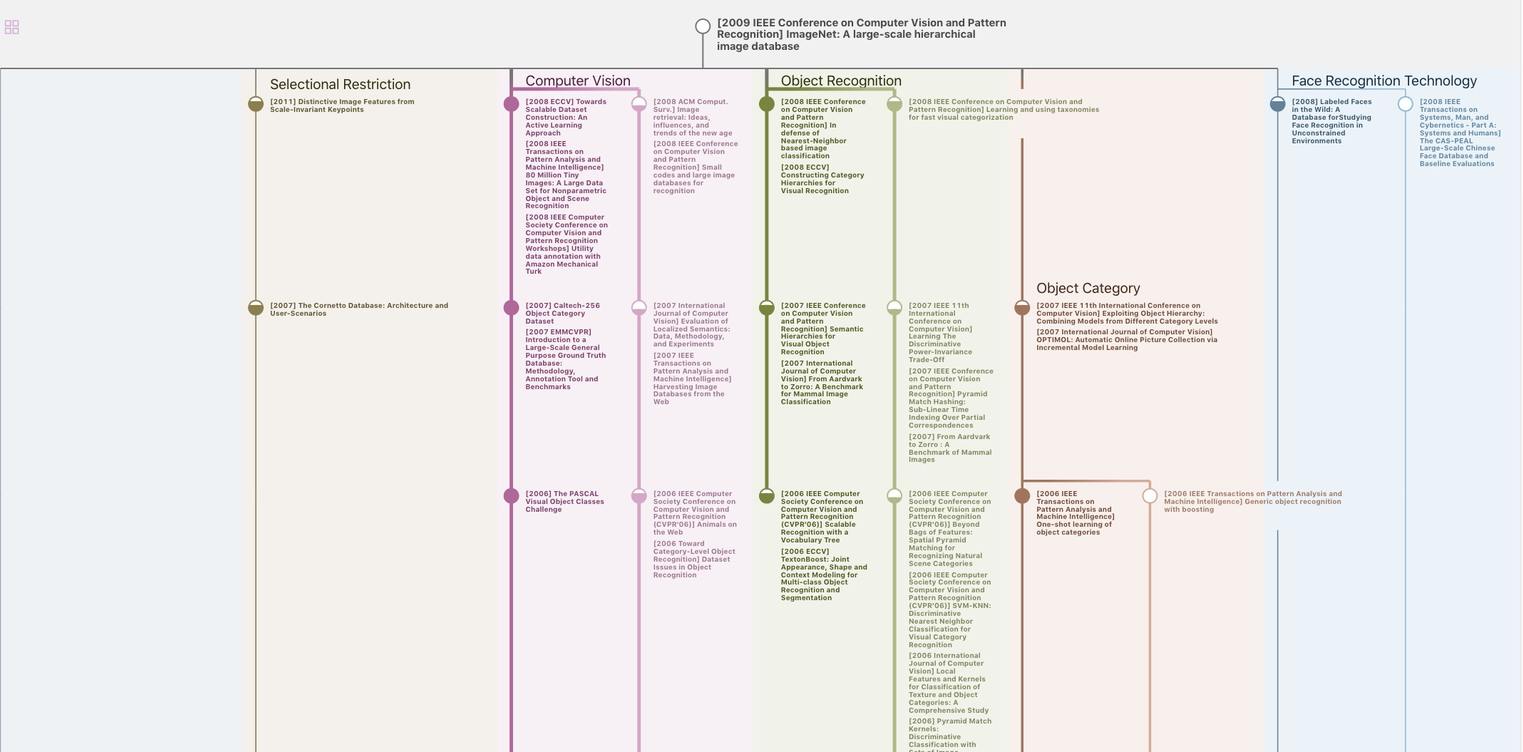
生成溯源树,研究论文发展脉络
Chat Paper
正在生成论文摘要