Organoids Segmentation Using Self-Supervised Learning: How Complex Should the Pretext Task Be?
ICBBE '23 Proceedings of the 2023 10th International Conference on Biomedical and Bioinformatics Engineering(2024)
摘要
Most popular supervised-learning approaches require large annotated data sets that are time-consuming and costly to create. Self-supervised learning (SSL) has proven to be a viable method for increasing downstream performance, through pre-training models on a pretext task. However, the literature is not conclusive on how to choose the best pretext task. This research sheds light on how the complexity of the pretext task affects organoid segmentation performance, in addition to understanding whether a self-prediction or innate relationship SSL strategy is best suited for organoid segmentation. Eight novel self-prediction distortion methods were implemented, creating eight simple and twenty-eight complex pretext tasks. Thosewere compared to two innate relationship pretext tasks: Jigsaw and Predict rotation. Results showed that the complexity of the pretext tasks does not correlate with segmentation performance. However, complex models (mu F1 = 0.862) consistently, albeit with a small effect size, outperform simple models (mu F1 = 0.848). Possibly due to acquiring a wider variety of learned features after pretext learning, despite not being necessarily more complex. Comparing SSL strategies showed that self-prediction models (mu F1 = 0.856) slightly outperform innate relationship models (mu F1 = 0.848). Furthermore, more pretext training data improves downstream performance under the condition that there is a minimum amount of downstream training data available. Too little downstream training data combined with more pretext training data leads to a decrease in segmentation performance.
更多查看译文
关键词
Deep Learning,Self-supervised Learning,Pretext Task,Segmentation,Organoids
AI 理解论文
溯源树
样例
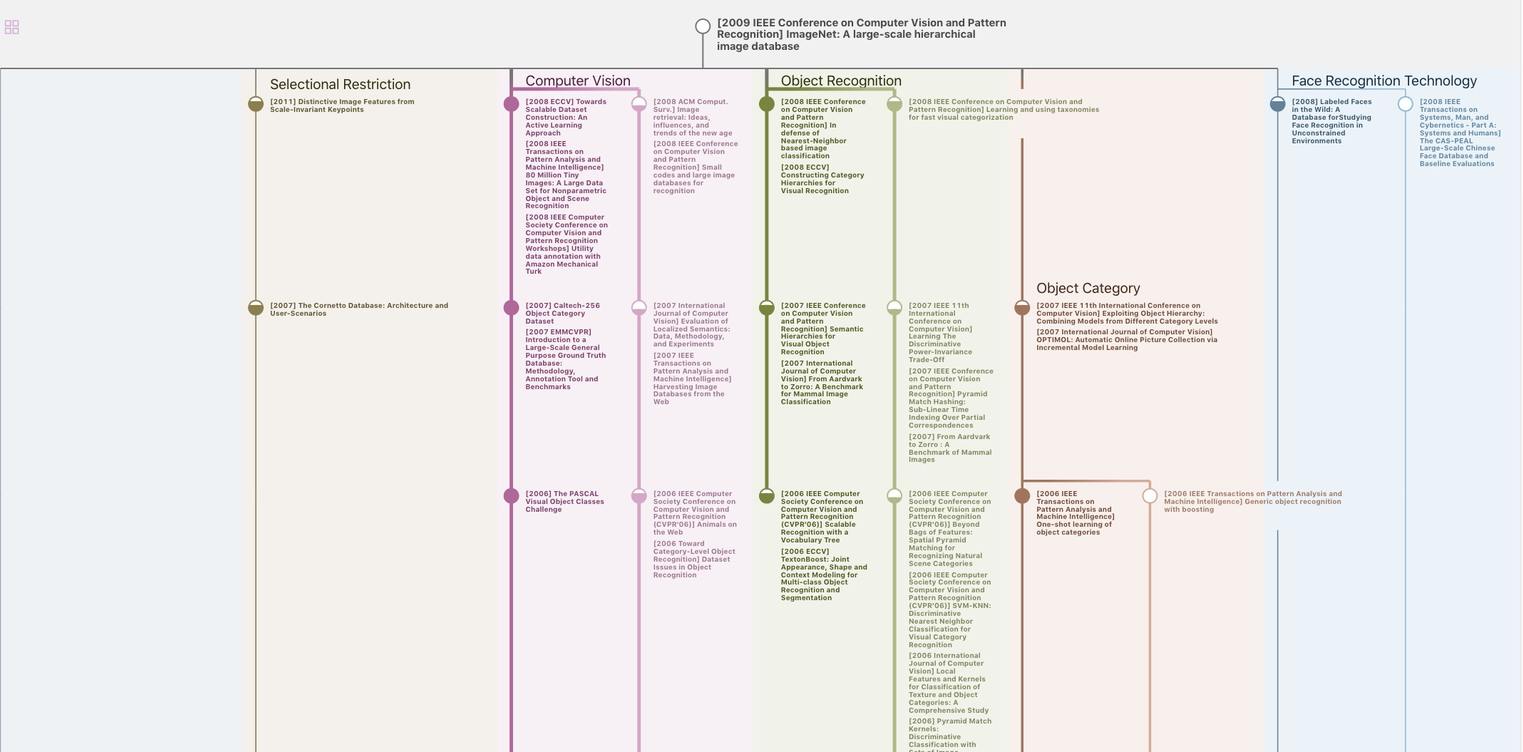
生成溯源树,研究论文发展脉络
Chat Paper
正在生成论文摘要