Comprehensive Analysis on Feature Selection, Machine Learning and Deep Learning Algorithms to Detect Driver Drowsiness-An EEG Study.
Proceedings of the 2023 10th International Conference on Biomedical and Bioinformatics Engineering(2023)
摘要
Drowsiness among drivers is one of the key reasons for traffic accidents which has been recognized as a global problem and this hazardous event causes physical injury, economic suffering, and even death to people. With the advancement of artificial intelligence, drowsiness can be detected from the brain signals and a prior alert can be generated to avoid such losses. For an optimal classification study, extensive analysis of major aspects such as optimal feature selection and choosing compatible classification algorithms should be done properly. This study focused on the classification of the drowsiness stage and active stage from continuous EEG signal (9 channels) starting with the implementation of feature selection algorithms to find the best temporal EEG features for feeding the classifiers and then undergoing different machine learning and deep learning algorithms. Recursive feature elimination and chi-square test, two popular feature selection algorithms, were utilized to find the most possible contributing features which were then fed to 17 machine learning models and 3 deep learning networks. After primary performance analysis selected machine learning models: K-Nearest Neighbor (KNN), Logistic Regression, Random Forest and Support Vector Machine (SVM) were chosen for hyperparametric tuning to achieve the highest performance. Among three deep learning algorithms such as long-short-term memory (LSTM), artificial neural network (ANN), and 1-dimensional convolutional network (1D-CNN), LSTM outperformed the rests with a classi- accuracy with lower resource consumption than the deep learning models. The goal of this study is to help in the improvement of a drowsiness classification system that is effective in preventing accidents and reducing the risk of harm to people and property.
更多查看译文
关键词
Drowsiness detection,Machine Learning,Deep Learning,Feature Engineering
AI 理解论文
溯源树
样例
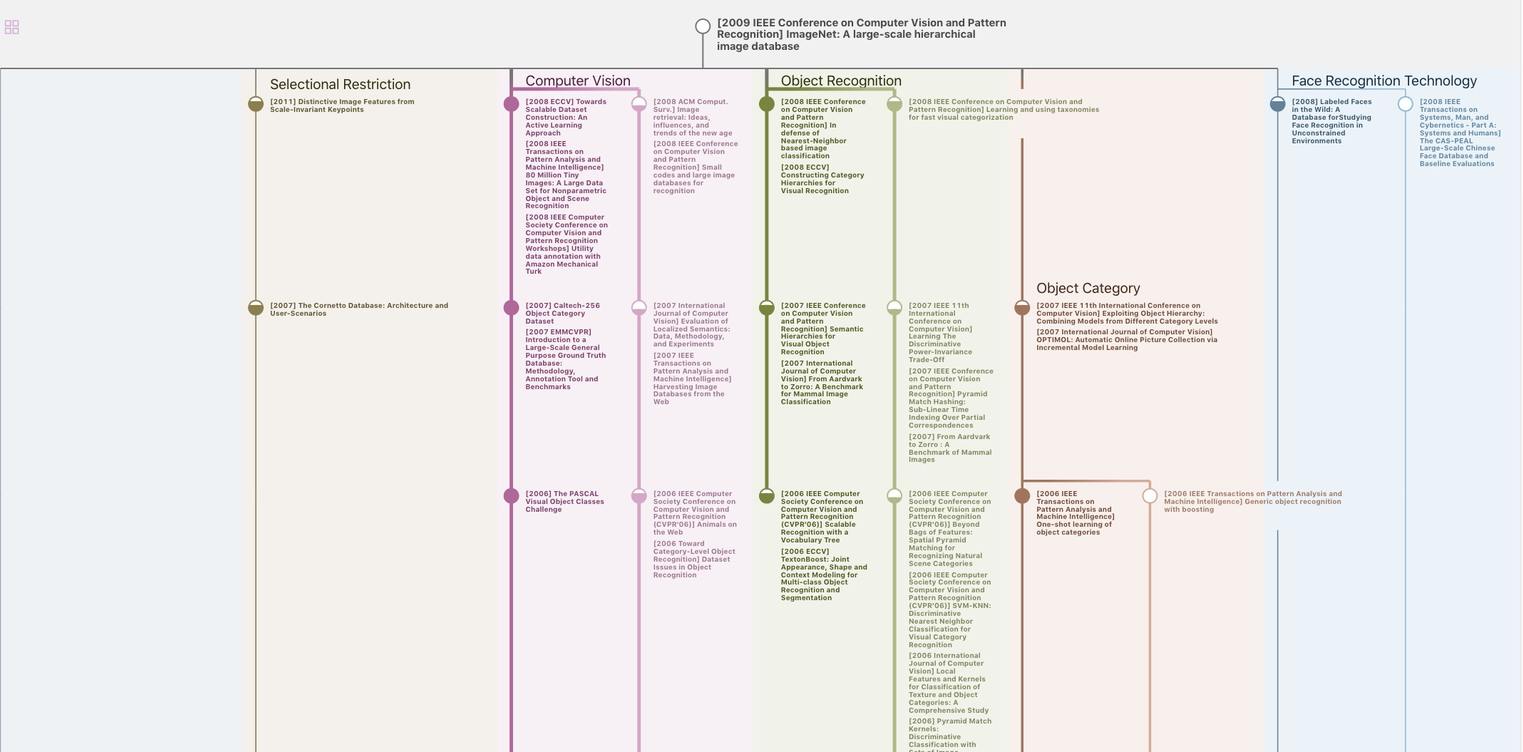
生成溯源树,研究论文发展脉络
Chat Paper
正在生成论文摘要