HGCLAMIR: Hypergraph contrastive learning with attention mechanism and integrated multi-view representation for predicting miRNA-disease associations
PLOS COMPUTATIONAL BIOLOGY(2024)
摘要
Existing studies have shown that the abnormal expression of microRNAs (miRNAs) usually leads to the occurrence and development of human diseases. Identifying disease-related miRNAs contributes to studying the pathogenesis of diseases at the molecular level. As traditional biological experiments are time-consuming and expensive, computational methods have been used as an effective complement to infer the potential associations between miRNAs and diseases. However, most of the existing computational methods still face three main challenges: (i) learning of high-order relations; (ii) insufficient representation learning ability; (iii) importance learning and integration of multi-view embedding representation. To this end, we developed a HyperGraph Contrastive Learning with view-aware Attention Mechanism and Integrated multi-view Representation (HGCLAMIR) model to discover potential miRNA-disease associations. First, hypergraph convolutional network (HGCN) was utilized to capture high-order complex relations from hypergraphs related to miRNAs and diseases. Then, we combined HGCN with contrastive learning to improve and enhance the embedded representation learning ability of HGCN. Moreover, we introduced view-aware attention mechanism to adaptively weight the embedded representations of different views, thereby obtaining the importance of multi-view latent representations. Next, we innovatively proposed integrated representation learning to integrate the embedded representation information of multiple views for obtaining more reasonable embedding information. Finally, the integrated representation information was fed into a neural network-based matrix completion method to perform miRNA-disease association prediction. Experimental results on the cross-validation set and independent test set indicated that HGCLAMIR can achieve better prediction performance than other baseline models. Furthermore, the results of case studies and enrichment analysis further demonstrated the accuracy of HGCLAMIR and unconfirmed potential associations had biological significance. Considerable studies have demonstrated that the dysregulation of miRNAs is closely related to human diseases. Therefore, inferring unconfirmed associations between miRNAs and diseases is helpful for disease diagnosis and treatment. Numerous computational models have been proposed to discover potential miRNA-disease associations on a large scale, which can accelerate the understanding of disease pathogenesis. We constructed a HGCLAMIR model to identify miRNA-disease associations through hypergraph convolutional network with contrastive learning, view-aware attention mechanism and integrated representation learning. The 5-fold cross-validation and independent testing were performed to evaluate the performance of HGCLAMIR, which was better than ten baseline models. In addition, we carried out case studies on breast neoplasms and lung neoplasms, showing that 49 and 48 of the top 50 candidate miRNAs were confirmed by experimental reports. In summary, HGCLAMIR could be considered as an effective and accurate model for predicting the associations between miRNAs and diseases.
更多查看译文
AI 理解论文
溯源树
样例
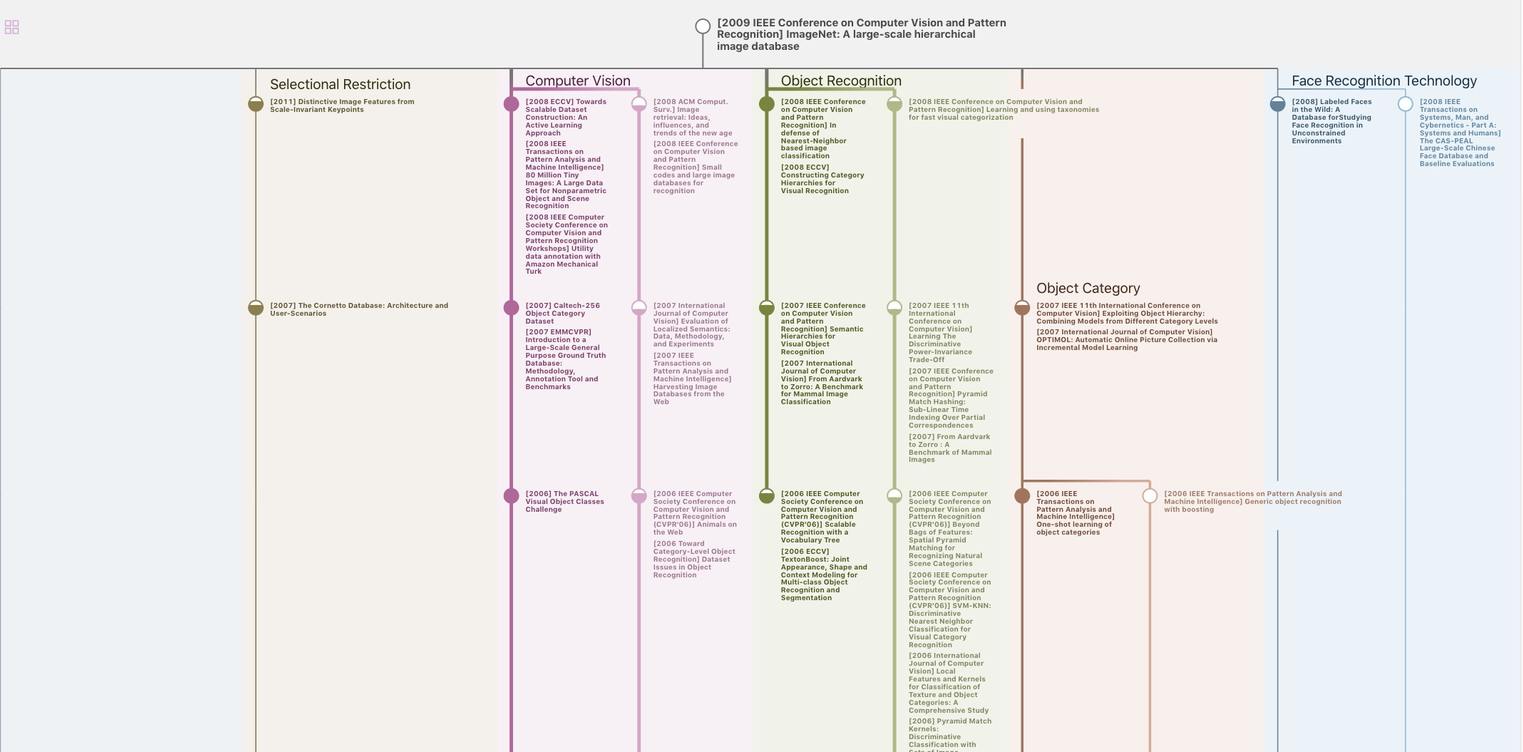
生成溯源树,研究论文发展脉络
Chat Paper
正在生成论文摘要