MFANet: Multifeature Aggregation Network for Cross-Granularity Few-Shot Seamless Steel Tubes Surface Defect Segmentation
IEEE TRANSACTIONS ON INDUSTRIAL INFORMATICS(2024)
摘要
Defect segmentation on the inner surface of seamless steel tubes (SSTs) is a crucial technical means for evaluating product quality. However, both the category and quantity of defective samples of SSTs are sparse, limiting the generalization of traditional supervised learning and general few-shot defect segmentation (FSDS) methodologies. Moreover, the existing fine-grained segmentation method results in an arduous and time-consuming dataset-building process. Motivated by this, a novel defect segmentation paradigm called cross-granularity FSDS (CG-FSDS) is proposed. This paradigm aims to learn the defect segmentation capability on the coarse-grained labeled defect dataset and subsequently generalize it to segment fine-grained labeled defective samples of SSTs. The feasibility of CG-FSDS is evaluated by the proposed multifeature aggregation network (MFANet). To address the real challenge of defect segmentation in SSTs, we establish a cross-granularity benchmark called CGFSDS-9, which consists of six categories of inner surface defects in SSTs with fine-grained annotation and three categories of general metal surface defect samples with coarse-grained annotation. Our MFANet achieves superior results compared to other FSDS methods and showcases state-of-the-art performance on this benchmark.
更多查看译文
关键词
Cross-granularity learning,few-shot segmentation (FSS),meta learning,multifeature aggregation,seamless steel tubes (SSTs)
AI 理解论文
溯源树
样例
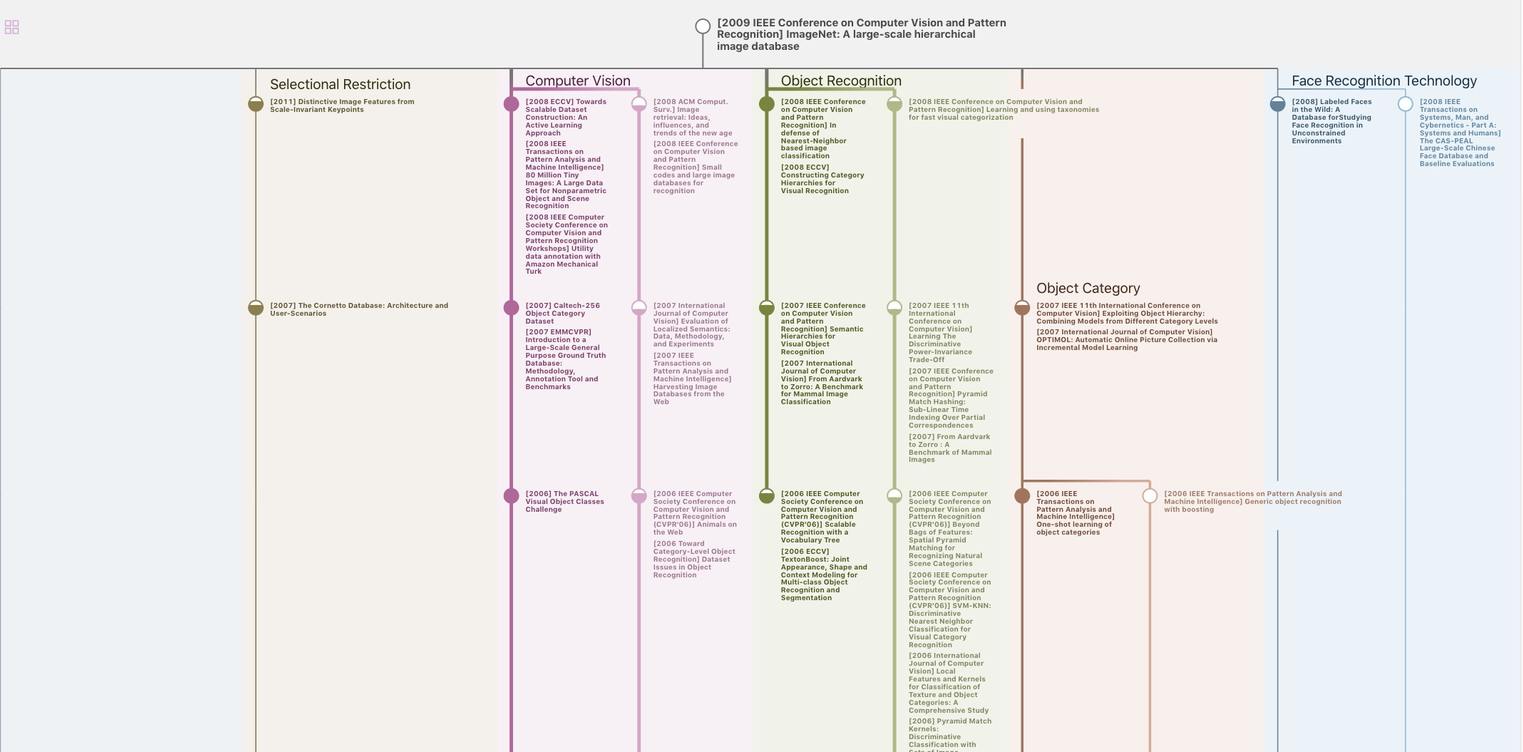
生成溯源树,研究论文发展脉络
Chat Paper
正在生成论文摘要