An Efficient Layer Selection Algorithm for Partial Federated Learning.
Annual IEEE International Conference on Pervasive Computing and Communications(2024)
摘要
This paper introduces the Partial Federated Learning for Driving Assistance (DrivePFL) algorithm, an algorithm for Vehicular Federated Learning (VFL) in Intelligent Transportation Services (ITS), addressing curreny challenges of Federated Learning (FL) in vehicular environments. Unlike traditional FL which requires transmitting entire Machine Learning (ML) models, DrivePFL optimizes resource usage by transmitting only critical model layers. It employs contact time prediction for V2V links to facilitate efficient data transfer and model accuracy in VFL scenarios. Implementing local model aggregation, eliminates the need for centralized servers, reducing bandwidth usage by 10% or more and maintaining a lower model transmission failure rate across varying vehicular densities. DrivePFL leverages mobility prediction for optimizing model layer transfer within communication windows and employs the Centered Kernel Alignment (CKA) similarity metric at model aggregation to ensure IID data collection and model personalization in heterogeneous datasets. This approach achieves an 83.40% accuracy rate, significantly outperforming state-of-the-art algorithms. The contributions include a local model aggregation mechanism, the application of contact time prediction for V2V links, and the use of the CKA similarity metric for a novel model aggregation scheme.
更多查看译文
关键词
Federated Learning,Vehicular Networks,Vehicular Ad Hoc Networks (VANETs)
AI 理解论文
溯源树
样例
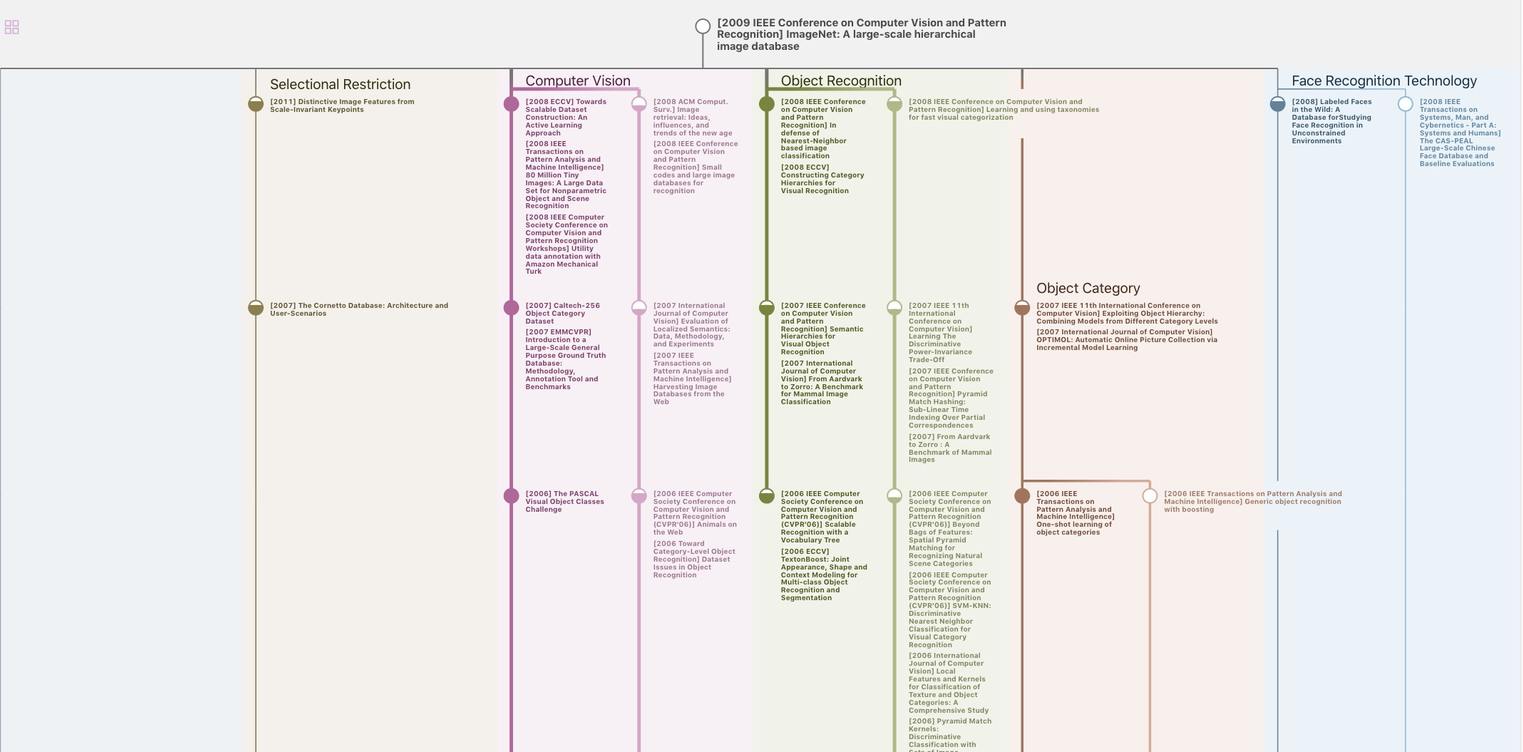
生成溯源树,研究论文发展脉络
Chat Paper
正在生成论文摘要