VRSTNN: Visual-Relational Spatio-Temporal Neural Network for Early Hazardous Event Detection in Automated Driving Systems
IEEE Transactions on Intelligent Vehicles(2024)
摘要
Reliable and early detection of hazardous events is vital for the safe deployment of automated driving systems. Yet, it remains challenging as road environments can be highly complex and dynamic. State-of-the-art solutions utilise neural networks to learn visual features and temporal patterns from collision videos. However, in this paper, we show how visual features alone may not provide the essential context needed to detect early warning patterns. To address these limitations, we first propose an input encoding that captures the context of the scene. This is achieved by formulating a scene as a graph to provide a framework to represent the arrangement, relationships and behaviours of each road user. We then process the graphs using graph neural networks to identify scene context from: 1) the collective behaviour of nearby road users based on their relationships and 2) local node features that describe individual behaviour. We then propose a novel visual-relational spatio-temporal neural network (VRSTNN) that leverages multi-modal processing to understand scene context and fuse it with the visual characteristics of the scene for more reliable and early hazard detection. Our results show that our VRSTNN outperforms state-of-the-art models in terms of accuracy, F1 and false negative rate on a real and synthetic benchmark dataset: DOTA and GTAC.
更多查看译文
关键词
Hazardous event detection,spatio-temporal neural networks,visual and relational graph networks,visual convolutional networks,automated and autonomous vehicles
AI 理解论文
溯源树
样例
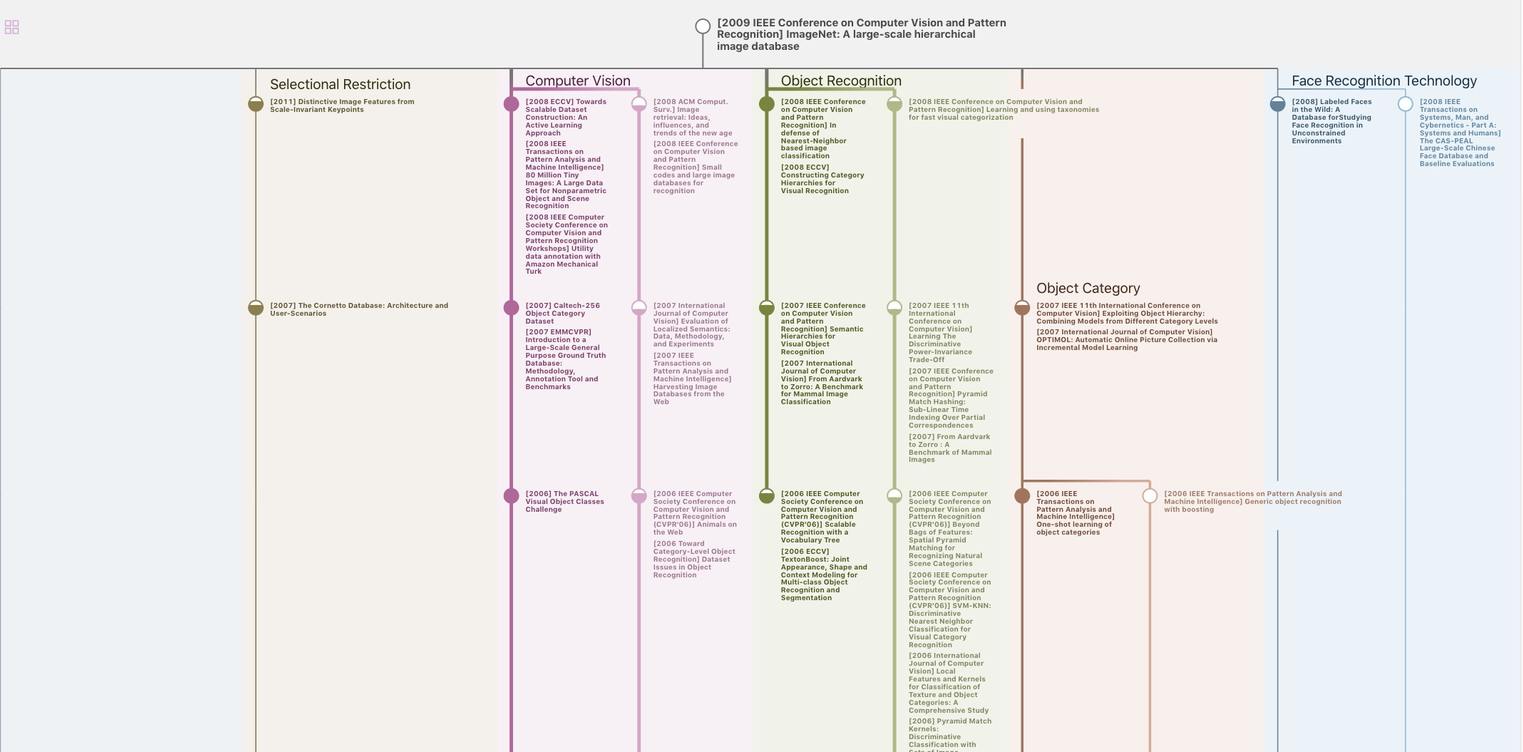
生成溯源树,研究论文发展脉络
Chat Paper
正在生成论文摘要