Assessment of Sensor Optimization Methods Toward State Estimation in a High-Dimensional System using Kalman Filter
IEEE Sensors Journal(2024)
摘要
The characteristics of the sensor selection method based on various algorithms for state estimation in a high-dimensional system using the Kalman filter were investigated. Sensors were selected based on the error covariance matrix of the Kalman filter. The performance of the sensor selection methods based on different algorithms, semidefinite programming, approximate convex relaxation, and greedy algorithm, including newly formulated methods, were compared by varying the number of potential sensor locations and the number of sensors to be selected under several noise ratio conditions. Two sensor selection methods for state estimation in a high-dimensional system using a Kalman filter were newly proposed based on the SDP with gain formulation and approximate convex relaxation, and the characteristics of the method including the previously proposed method were compared in a high-dimensional system. Although the condition was limited at n <
O
(10
3
), the approximate convex relaxation method and its randomized method are effective in terms of computational time and objective value for a small-scale problem. The objective value obtained by the greedy method shows the best performance compared to the other methods in almost all investigated conditions. Particularly, the greedy method outperforms other methods when the number of selected sensors is small. In addition, only the greedy method can handle the large-scale problem of n >
O
(10
4
). Overall, the greedy-based method is found to be favorable in large-scale problems in terms of computation time and the performance of the obtained sensor set.
更多查看译文
关键词
Sensor selection,optimal design of experiment,Kalman filter
AI 理解论文
溯源树
样例
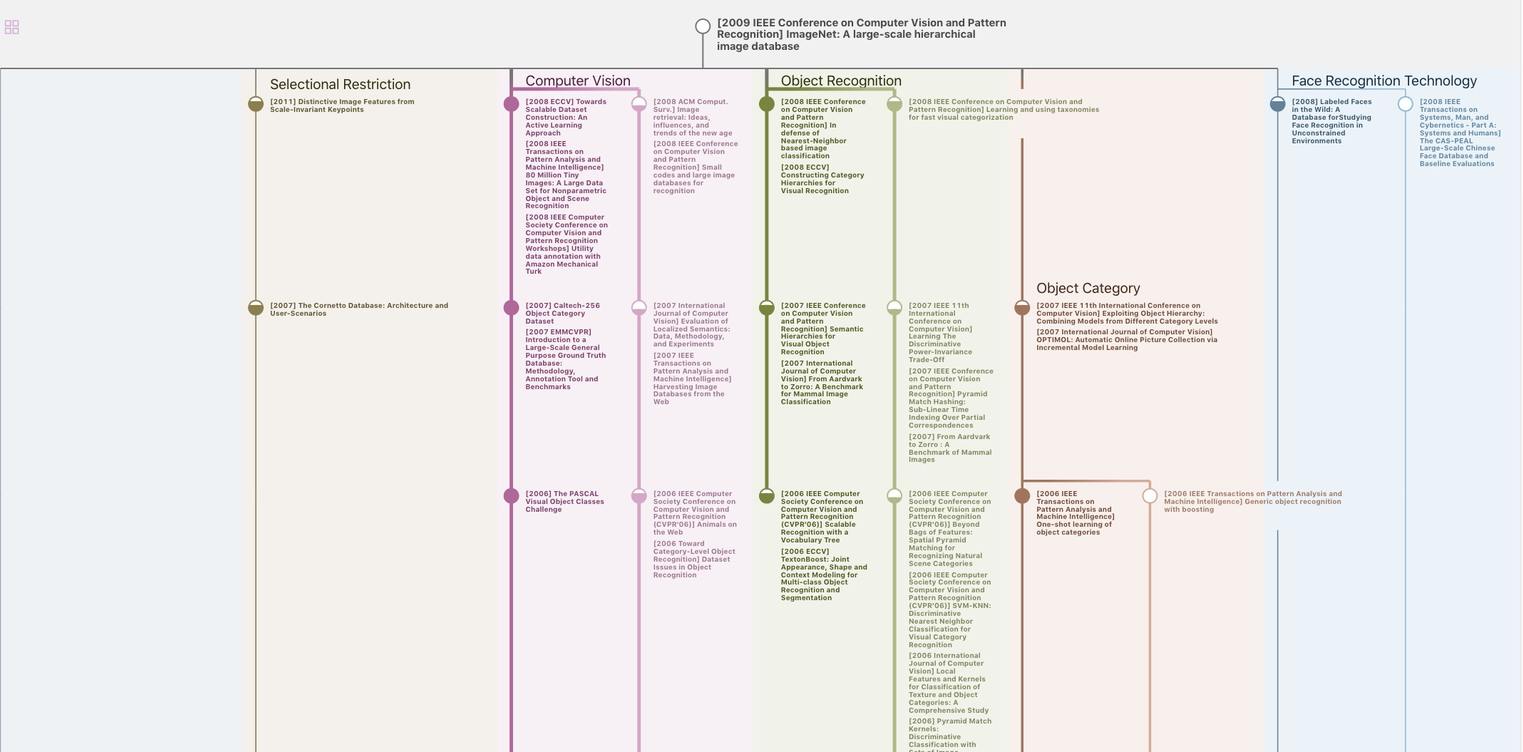
生成溯源树,研究论文发展脉络
Chat Paper
正在生成论文摘要