Adaptive Global Embedding Learning: A Two-Stage Framework for UAV-View Geo-Localization
IEEE Signal Processing Letters(2024)
摘要
This letter aims to deal with the UAV-view geolocalization problem, which is essentially to achieve bi-directional cross-view matching between UAV-view and satellite-view images. The existing studies have confirmed the importance of learning part-wise representations for this task. We go a step further by proposing a two-stage learning framework. The first stage focuses on extracting part-wise representations. In the second stage, a novel Adaptive Embedding Network (AEN) integrates these representations into a global embedding of the entire image to avoid an equal influence of all local parts on image similarity measures. Current mainstream methods typically employ CrossEntropy loss to learn location-dependent representations, aiming to push the distance between different locations in the learned representation space. Some approaches also utilize KL loss or Triplet loss to bring a pair of UAV-satellite images from the same location closer for learning view-invariant representations. However, they overlook a critical concern: a notable representation bias exists among UA-view images captured from the same location but at different viewpoints or heights. To address these issues, we devise a novel cross-view matching loss that narrows the distance between the global embeddings of a satellite-view image and the affinity-aware prototype of multiple true-matched UAV-view images. The experimental results on the University1652 dataset indicate that similarity measures in the learned embedding space exhibit excellent generalization to images from new locations, achieving superior cross-view matching performance compared to previous methods
更多查看译文
关键词
Cross-view matching loss,global embedding,part-wise representations,UAV-view geo-localization
AI 理解论文
溯源树
样例
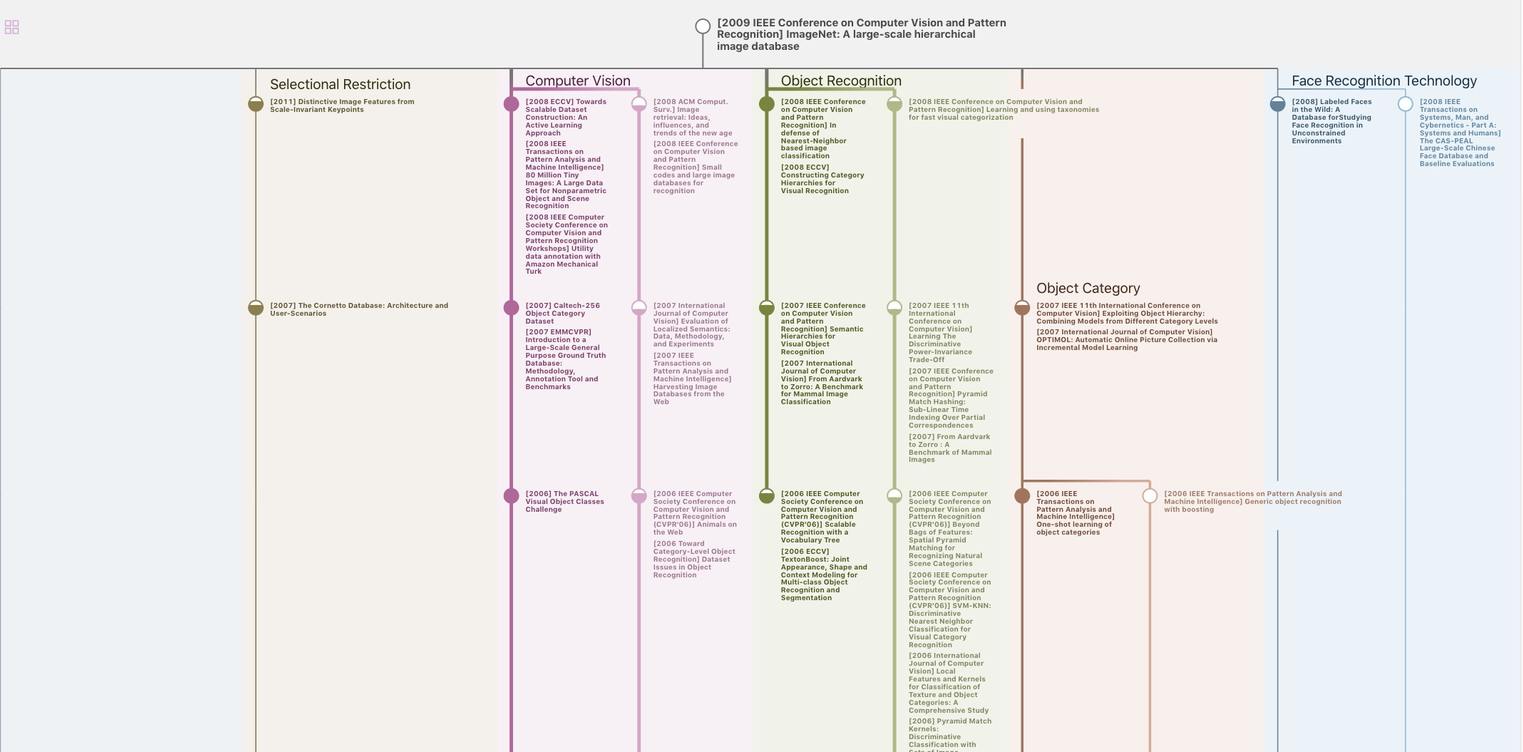
生成溯源树,研究论文发展脉络
Chat Paper
正在生成论文摘要